MultiStart¶
- class MultiStart(*args)¶
Multi-start optimization algorithm.
The algorithm runs an optimization solver for multiple starting points and returns the best result of each local search. The algorithm succeeds when at least one local search succeeds.
- Parameters:
- solver
OptimizationAlgorithm
The internal solver
- startingSample2-d sequence of float
Starting points set
- solver
Methods
Accessor to check status flag.
Accessor to the object's name.
Flag to keep intermediate results accessor.
Accessor to maximum allowed absolute error.
Accessor to maximum allowed number of calls.
Accessor to maximum allowed constraint error.
Accessor to maximum allowed number of iterations.
Accessor to maximum allowed relative error.
Accessor to maximum allowed residual error.
Accessor to the maximum duration.
getName
()Accessor to the object's name.
Internal solver accessor.
Accessor to optimization problem.
Accessor to optimization result.
Intermediate optimization results accessor.
Inherited but raises an Exception.
Accessor to the sample of starting points.
hasName
()Test if the object is named.
run
()Launch the optimization.
setCheckStatus
(checkStatus)Accessor to check status flag.
setKeepResults
(keepResults)Flag to keep intermediate results accessor.
setMaximumAbsoluteError
(maximumAbsoluteError)Accessor to maximum allowed absolute error.
setMaximumCallsNumber
(maximumCallsNumber)Accessor to maximum allowed number of calls
setMaximumConstraintError
(maximumConstraintError)Accessor to maximum allowed constraint error.
setMaximumIterationNumber
(maximumIterationNumber)Accessor to maximum allowed number of iterations.
setMaximumRelativeError
(maximumRelativeError)Accessor to maximum allowed relative error.
setMaximumResidualError
(maximumResidualError)Accessor to maximum allowed residual error.
setMaximumTimeDuration
(maximumTime)Accessor to the maximum duration.
setName
(name)Accessor to the object's name.
setOptimizationAlgorithm
(solver)Internal solver accessor.
setProblem
(problem)Sets the optimization problem.
setProgressCallback
(*args)Set up a progress callback.
setResult
(result)Accessor to optimization result.
setStartingPoint
(point)Inherited but raises an Exception.
setStartingSample
(startingSample)Accessor to the sample of starting points.
setStopCallback
(*args)Set up a stop callback.
Notes
The starting point of the internal solver is ignored. If you want to use it, add it to startingSample.
Stopping criteria used are the ones set in the internal solver.
A global number of evaluations can be set, in that case all starting points might not be used depending on the number of evaluations allocated to the internal solver.
Starting points provided through the startingSample parameter should be within the bounds of the
OptimizationProblem
, but this is not enforced.Examples
First define the
OptimizationAlgorithm
to be run from multiple starting points.>>> import openturns as ot >>> dim = 2 >>> model = ot.SymbolicFunction(['x', 'y'], ['x^2+y^2*(1-x)^3']) >>> bounds = ot.Interval([-2.0] * dim, [3.0] * dim) >>> problem = ot.OptimizationProblem(model) >>> problem.setBounds(bounds) >>> solver = ot.TNC(problem)
Starting points must be manually specified.
>>> uniform = ot.JointDistribution([ot.Uniform(-2.0, 3.0)] * dim) >>> ot.RandomGenerator.SetSeed(0) >>> startingSample = uniform.getSample(5) >>> print(startingSample) [ X0 X1 ] 0 : [ 1.14938 2.84712 ] 1 : [ 2.41403 2.6034 ] 2 : [ -1.32362 0.515201 ] 3 : [ -1.83749 -1.68397 ] 4 : [ -0.264715 -0.536216 ] >>> algo = ot.MultiStart(solver, startingSample) >>> algo.run() >>> result = algo.getResult() >>> print(result.getOptimalPoint()) [3,3]
- __init__(*args)¶
- getCheckStatus()¶
Accessor to check status flag.
- Returns:
- checkStatusbool
Whether to check the termination status. If set to False,
run()
will not throw an exception if the algorithm does not fully converge and will allow one to still find a feasible candidate.
- getClassName()¶
Accessor to the object’s name.
- Returns:
- class_namestr
The object class name (object.__class__.__name__).
- getKeepResults()¶
Flag to keep intermediate results accessor.
- Returns:
- keepResultsbool
If True all the intermediate results are stored, otherwise they are ignored. Default value is MultiStart-KeepResults in
ResourceMap
- getMaximumAbsoluteError()¶
Accessor to maximum allowed absolute error.
- Returns:
- maximumAbsoluteErrorfloat
Maximum allowed absolute error, where the absolute error is defined by
where
and
are two consecutive approximations of the optimum.
- getMaximumCallsNumber()¶
Accessor to maximum allowed number of calls.
- Returns:
- maximumEvaluationNumberint
Maximum allowed number of direct objective function calls through the () operator. Does not take into account eventual indirect calls through finite difference gradient calls.
- getMaximumConstraintError()¶
Accessor to maximum allowed constraint error.
- Returns:
- maximumConstraintErrorfloat
Maximum allowed constraint error, where the constraint error is defined by
where
is the current approximation of the optimum and
is the function that gathers all the equality and inequality constraints (violated values only)
- getMaximumIterationNumber()¶
Accessor to maximum allowed number of iterations.
- Returns:
- maximumIterationNumberint
Maximum allowed number of iterations.
- getMaximumRelativeError()¶
Accessor to maximum allowed relative error.
- Returns:
- maximumRelativeErrorfloat
Maximum allowed relative error, where the relative error is defined by
if
, else
.
- getMaximumResidualError()¶
Accessor to maximum allowed residual error.
- Returns:
- maximumResidualErrorfloat
Maximum allowed residual error, where the residual error is defined by
if
, else
.
- getMaximumTimeDuration()¶
Accessor to the maximum duration.
- Returns:
- maximumTimefloat
Maximum optimization duration in seconds.
- getName()¶
Accessor to the object’s name.
- Returns:
- namestr
The name of the object.
- getOptimizationAlgorithm()¶
Internal solver accessor.
- Returns:
- solver
OptimizationAlgorithm
The internal solver
- solver
- getProblem()¶
Accessor to optimization problem.
- Returns:
- problem
OptimizationProblem
Optimization problem.
- problem
- getResult()¶
Accessor to optimization result.
- Returns:
- result
OptimizationResult
Result class.
- result
- getResultCollection()¶
Intermediate optimization results accessor.
- Returns:
- results
OptimizationResultCollection
Intermediate optimization results
- results
- getStartingPoint()¶
Inherited but raises an Exception.
Notes
This method is inherited from
OptimizationAlgorithm
but makes no sense in a multi-start context.
- getStartingSample()¶
Accessor to the sample of starting points.
- hasName()¶
Test if the object is named.
- Returns:
- hasNamebool
True if the name is not empty.
- run()¶
Launch the optimization.
- setCheckStatus(checkStatus)¶
Accessor to check status flag.
- Parameters:
- checkStatusbool
Whether to check the termination status. If set to False,
run()
will not throw an exception if the algorithm does not fully converge and will allow one to still find a feasible candidate.
- setKeepResults(keepResults)¶
Flag to keep intermediate results accessor.
- Parameters:
- keepResultsbool
If True all the intermediate results are stored, otherwise they are ignored. Default value is MultiStart-KeepResults in
ResourceMap
- setMaximumAbsoluteError(maximumAbsoluteError)¶
Accessor to maximum allowed absolute error.
- Parameters:
- maximumAbsoluteErrorfloat
Maximum allowed absolute error, where the absolute error is defined by
where
and
are two consecutive approximations of the optimum.
- setMaximumCallsNumber(maximumCallsNumber)¶
Accessor to maximum allowed number of calls
- Parameters:
- maximumEvaluationNumberint
Maximum allowed number of direct objective function calls through the () operator. Does not take into account eventual indirect calls through finite difference gradient calls.
- setMaximumConstraintError(maximumConstraintError)¶
Accessor to maximum allowed constraint error.
- Parameters:
- maximumConstraintErrorfloat
Maximum allowed constraint error, where the constraint error is defined by
where
is the current approximation of the optimum and
is the function that gathers all the equality and inequality constraints (violated values only)
- setMaximumIterationNumber(maximumIterationNumber)¶
Accessor to maximum allowed number of iterations.
- Parameters:
- maximumIterationNumberint
Maximum allowed number of iterations.
- setMaximumRelativeError(maximumRelativeError)¶
Accessor to maximum allowed relative error.
- Parameters:
- maximumRelativeErrorfloat
Maximum allowed relative error, where the relative error is defined by
if
, else
.
- setMaximumResidualError(maximumResidualError)¶
Accessor to maximum allowed residual error.
- Parameters:
- maximumResidualErrorfloat
Maximum allowed residual error, where the residual error is defined by
if
, else
.
- setMaximumTimeDuration(maximumTime)¶
Accessor to the maximum duration.
- Parameters:
- maximumTimefloat
Maximum optimization duration in seconds.
- setName(name)¶
Accessor to the object’s name.
- Parameters:
- namestr
The name of the object.
- setOptimizationAlgorithm(solver)¶
Internal solver accessor.
- Parameters:
- solver
OptimizationAlgorithm
The internal solver
- solver
- setProblem(problem)¶
Sets the optimization problem.
- Parameters:
- problem
OptimizationProblem
Optimization problem.
- problem
- setProgressCallback(*args)¶
Set up a progress callback.
Can be used to programmatically report the progress of an optimization.
- Parameters:
- callbackcallable
Takes a float as argument as percentage of progress.
Examples
>>> import sys >>> import openturns as ot >>> rosenbrock = ot.SymbolicFunction(['x1', 'x2'], ['(1-x1)^2+100*(x2-x1^2)^2']) >>> problem = ot.OptimizationProblem(rosenbrock) >>> solver = ot.OptimizationAlgorithm(problem) >>> solver.setStartingPoint([0, 0]) >>> solver.setMaximumResidualError(1.e-3) >>> solver.setMaximumCallsNumber(10000) >>> def report_progress(progress): ... sys.stderr.write('-- progress=' + str(progress) + '%\n') >>> solver.setProgressCallback(report_progress) >>> solver.run()
- setResult(result)¶
Accessor to optimization result.
- Parameters:
- result
OptimizationResult
Result class.
- result
- setStartingPoint(point)¶
Inherited but raises an Exception.
Notes
This method is inherited from
OptimizationAlgorithm
but makes no sense in a multi-start context.
- setStartingSample(startingSample)¶
Accessor to the sample of starting points.
- Parameters:
- startingSample2-d sequence of float
A new sample of starting points to overwrite the existing sample
- setStopCallback(*args)¶
Set up a stop callback.
Can be used to programmatically stop an optimization.
- Parameters:
- callbackcallable
Returns an int deciding whether to stop or continue.
Examples
>>> import openturns as ot >>> rosenbrock = ot.SymbolicFunction(['x1', 'x2'], ['(1-x1)^2+100*(x2-x1^2)^2']) >>> problem = ot.OptimizationProblem(rosenbrock) >>> solver = ot.OptimizationAlgorithm(problem) >>> solver.setStartingPoint([0, 0]) >>> solver.setMaximumResidualError(1.e-3) >>> solver.setMaximumCallsNumber(10000) >>> def ask_stop(): ... return True >>> solver.setStopCallback(ask_stop) >>> solver.run()
Examples using the class¶
Gaussian Process Regression: surrogate model with continuous and categorical variables
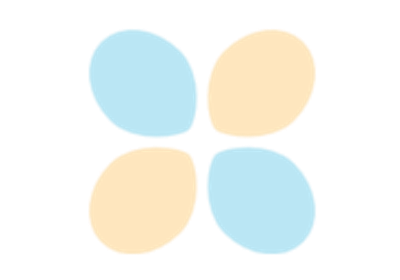
Gaussian process fitter: configure the optimization solver