Note
Click here to download the full example code
Taylor approximationsΒΆ
In this example we are going to build a local approximation of a model using the taylor decomposition:
Here is the decomposition at the first order:
Here .
from __future__ import print_function
import openturns as ot
import openturns.viewer as viewer
from matplotlib import pylab as plt
ot.Log.Show(ot.Log.NONE)
# prepare some data
formulas = ['cos(x1 + x2)', '(x2 + 1) * exp(x1 - 2 * x2)']
model = ot.SymbolicFunction(['x1', 'x2'], formulas)
# center of the approximation
x0 = [-0.4, -0.4]
# drawing bounds
a=-0.4
b=0.0
create a linear (first order) Taylor approximation
algo = ot.LinearTaylor(x0, model)
algo.run()
responseSurface = algo.getMetaModel()
plot 2nd output of our model with x1=x0_1
graph = ot.ParametricFunction(responseSurface, [0], [x0[1]]).getMarginal(1).draw(a, b)
graph.setLegends(['taylor'])
curve = ot.ParametricFunction(model, [0], [x0[1]]).getMarginal(1).draw(a, b).getDrawable(0)
curve.setColor('red')
curve.setLegend('model')
graph.add(curve)
graph.setLegendPosition('topright')
view = viewer.View(graph)
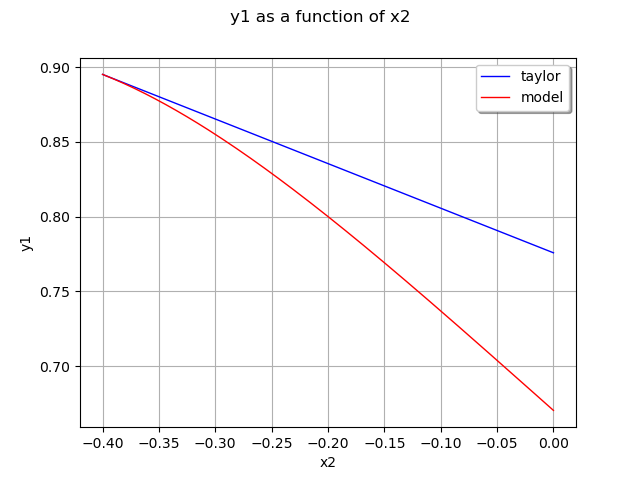
Here is the decomposition at the second order:
create a quadratic (2nd order) Taylor approximation
algo = ot.QuadraticTaylor(x0, model)
algo.run()
responseSurface = algo.getMetaModel()
plot 2nd output of our model with x1=x0_1
graph = ot.ParametricFunction(responseSurface, [0], [x0[1]]).getMarginal(1).draw(a, b)
graph.setLegends(['taylor'])
curve = ot.ParametricFunction(model, [0], [x0[1]]).getMarginal(1).draw(a, b).getDrawable(0)
curve.setColor('red')
curve.setLegend('model')
graph.add(curve)
graph.setLegendPosition('topright')
view = viewer.View(graph)
plt.show()
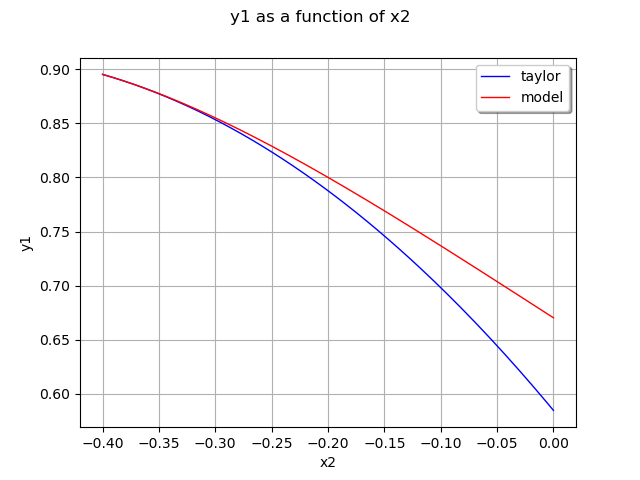
Total running time of the script: ( 0 minutes 0.225 seconds)