MemoizeFunction¶
- class MemoizeFunction(*args)¶
Function which keeps tracks of input and output.
When this function is evaluated, it calls the
Function
passed as argument, and store input and outputSample
. It also has a caching behavior, enabled by default.- Parameters:
- function
Function
Delegate function
- historyStrategy
HistoryStrategy
(optional) Strategy used to store points, default is
Full
.
- function
Notes
When the function passed as argument is a
MemoizeFunction
, its input and output history are copied into current instance. This allows one to retrieve this history from aFunction
object which is in fact aMemoizeFunction
. Thus, if you create aMemoizeFunction
from an unknownFunction
, it is better to callclearHistory()
. The cache size is initialized by the value of theResourceMap
Cache-MaxSize entry.Examples
>>> import openturns as ot >>> f = ot.SymbolicFunction('x', 'x^2') >>> inputSample = ot.Sample([[1], [2], [3], [4]]) >>> f = ot.MemoizeFunction(f) >>> outputSample = f(inputSample)
Retrieve input sample:
>>> print(f.getInputHistory()) 0 : [ 1 ] 1 : [ 2 ] 2 : [ 3 ] 3 : [ 4 ]
Retrieve output sample:
>>> print(f.getOutputHistory()) 0 : [ 1 ] 1 : [ 4 ] 2 : [ 9 ] 3 : [ 16 ]
Methods
addCacheContent
(inSample, outSample)Add input numerical points and associated output to the cache.
Empty the content of the cache.
Clear input and output history.
Disable the cache mechanism.
Disable the history mechanism.
draw
(*args)Draw the output of function as a
Graph
.Enable the cache mechanism.
Enable the history mechanism.
Accessor to the number of computations saved thanks to the cache mechanism.
Accessor to all the input numerical points stored in the cache mechanism.
Accessor to all the output numerical points stored in the cache mechanism.
Accessor to the number of direct calls to the function.
Accessor to the object's name.
Accessor to the description of the inputs and outputs.
Accessor to the evaluation function.
Accessor to the number of times the evaluation of the function has been called.
Accessor to the gradient function.
Accessor to the number of times the gradient of the function has been called.
Accessor to the hessian function.
Accessor to the number of times the hessian of the function has been called.
Accessor to the description of the input vector.
Accessor to the dimension of the input vector.
Get the input sample.
getMarginal
(*args)Accessor to marginal.
getName
()Accessor to the object's name.
Accessor to the description of the output vector.
Accessor to the number of the outputs.
Get the output sample.
Accessor to the parameter values.
Accessor to the parameter description.
Accessor to the dimension of the parameter.
gradient
(inP)Return the Jacobian transposed matrix of the function at a point.
hasName
()Test if the object is named.
hessian
(inP)Return the hessian of the function at a point.
Test whether the cache mechanism is enabled or not.
Test whether the history mechanism is enabled or not.
isLinear
()Accessor to the linearity of the function.
isLinearlyDependent
(index)Accessor to the linearity of the function with regard to a specific variable.
parameterGradient
(inP)Accessor to the gradient against the parameter.
setDescription
(description)Accessor to the description of the inputs and outputs.
setEvaluation
(evaluation)Accessor to the evaluation function.
setGradient
(gradient)Accessor to the gradient function.
setHessian
(hessian)Accessor to the hessian function.
setInputDescription
(inputDescription)Accessor to the description of the input vector.
setName
(name)Accessor to the object's name.
setOutputDescription
(outputDescription)Accessor to the description of the output vector.
setParameter
(parameter)Accessor to the parameter values.
setParameterDescription
(description)Accessor to the parameter description.
setStopCallback
(callBack[, state])Set up a stop callback.
- __init__(*args)¶
- addCacheContent(inSample, outSample)¶
Add input numerical points and associated output to the cache.
- Parameters:
- input_sample2-d sequence of float
Input numerical points to be added to the cache.
- output_sample2-d sequence of float
Output numerical points associated with the input_sample to be added to the cache.
- clearCache()¶
Empty the content of the cache.
- clearHistory()¶
Clear input and output history.
- disableCache()¶
Disable the cache mechanism.
- disableHistory()¶
Disable the history mechanism.
- draw(*args)¶
Draw the output of function as a
Graph
.- Available usages:
draw(inputMarg, outputMarg, centralPoint, xiMin, xiMax, ptNb, scale)
draw(firstInputMarg, secondInputMarg, outputMarg, centralPoint, xiMin_xjMin, xiMax_xjMax, ptNbs, scale, isFilled)
draw(xiMin, xiMax, ptNb, scale)
draw(xiMin_xjMin, xiMax_xjMax, ptNbs, scale)
- Parameters:
- outputMarg, inputMargint,
outputMarg is the index of the marginal to draw as a function of the marginal with index inputMarg.
- firstInputMarg, secondInputMargint,
In the 2D case, the marginal outputMarg is drawn as a function of the two marginals with indexes firstInputMarg and secondInputMarg.
- centralPointsequence of float
Central point with dimension equal to the input dimension of the function.
- xiMin, xiMaxfloat
Define the interval where the curve is plotted.
- xiMin_xjMin, xiMax_xjMaxsequence of float of dimension 2.
In the 2D case, define the intervals where the curves are plotted.
- ptNbint
The number of points to draw the curves.
- ptNbslist of int of dimension 2
The number of points to draw the contour in the 2D case.
- scalebool
scale indicates whether the logarithmic scale is used either for one or both axes:
ot.GraphImplementation.NONE or 0: no log scale is used,
ot.GraphImplementation.LOGX or 1: log scale is used only for horizontal data,
ot.GraphImplementation.LOGY or 2: log scale is used only for vertical data,
ot.GraphImplementation.LOGXY or 3: log scale is used for both data.
- isFilledbool
isFilled indicates whether the contour graph is filled or not
- outputMarg, inputMargint,
Notes
We note
where
and
, with
and
.
In the first usage:
Draws graph of the given 1D outputMarg marginal
as a function of the given 1D inputMarg marginal with respect to the variation of
in the interval
, when all the other components of
are fixed to the corresponding components of the centralPoint
. Then OpenTURNS draws the graph:
for any
where
is defined by the equation:
In the second usage:
Draws the iso-curves of the given outputMarg marginal
as a function of the given 2D firstInputMarg and secondInputMarg marginals with respect to the variation of
in the interval
, when all the other components of
are fixed to the corresponding components of the centralPoint
. Then OpenTURNS draws the graph:
for any
where
is defined by the equation:
In the third usage:
The same as the first usage but only for function
.
In the fourth usage:
The same as the second usage but only for function
.
Examples
>>> import openturns as ot >>> from openturns.viewer import View >>> f = ot.SymbolicFunction('x', 'sin(2*pi_*x)*exp(-x^2/2)') >>> graph = f.draw(-1.2, 1.2, 100) >>> View(graph).show()
- enableCache()¶
Enable the cache mechanism.
- enableHistory()¶
Enable the history mechanism.
- getCacheHits()¶
Accessor to the number of computations saved thanks to the cache mechanism.
- Returns:
- cacheHitsint
Integer that counts the number of computations saved thanks to the cache mechanism.
- getCacheInput()¶
Accessor to all the input numerical points stored in the cache mechanism.
- Returns:
- cacheInput
Sample
All the input numerical points stored in the cache mechanism.
- cacheInput
- getCacheOutput()¶
Accessor to all the output numerical points stored in the cache mechanism.
- Returns:
- cacheInput
Sample
All the output numerical points stored in the cache mechanism.
- cacheInput
- getCallsNumber()¶
Accessor to the number of direct calls to the function.
- Returns:
- calls_numberint
Integer that counts the number of times the function has been called directly through the () operator.
- getClassName()¶
Accessor to the object’s name.
- Returns:
- class_namestr
The object class name (object.__class__.__name__).
- getDescription()¶
Accessor to the description of the inputs and outputs.
- Returns:
- description
Description
Description of the inputs and the outputs.
- description
Examples
>>> import openturns as ot >>> f = ot.SymbolicFunction(['x1', 'x2'], ... ['2 * x1^2 + x1 + 8 * x2 + 4 * cos(x1) * x2 + 6']) >>> print(f.getDescription()) [x1,x2,y0]
- getEvaluation()¶
Accessor to the evaluation function.
- Returns:
- function
EvaluationImplementation
The evaluation function.
- function
Examples
>>> import openturns as ot >>> f = ot.SymbolicFunction(['x1', 'x2'], ... ['2 * x1^2 + x1 + 8 * x2 + 4 * cos(x1) * x2 + 6']) >>> print(f.getEvaluation()) [x1,x2]->[2 * x1^2 + x1 + 8 * x2 + 4 * cos(x1) * x2 + 6]
- getEvaluationCallsNumber()¶
Accessor to the number of times the evaluation of the function has been called.
- Returns:
- evaluation_calls_numberint
Integer that counts the number of times the evaluation of the function has been called since its creation. This may include indirect calls via finite-difference gradient or Hessian.
- getGradient()¶
Accessor to the gradient function.
- Returns:
- gradient
GradientImplementation
The gradient function.
- gradient
- getGradientCallsNumber()¶
Accessor to the number of times the gradient of the function has been called.
- Returns:
- gradient_calls_numberint
Integer that counts the number of times the gradient of the Function has been called since its creation. Note that if the gradient is implemented by a finite difference method, the gradient calls number is equal to 0 and the different calls are counted in the evaluation calls number.
- getHessian()¶
Accessor to the hessian function.
- Returns:
- hessian
HessianImplementation
The hessian function.
- hessian
- getHessianCallsNumber()¶
Accessor to the number of times the hessian of the function has been called.
- Returns:
- hessian_calls_numberint
Integer that counts the number of times the hessian of the Function has been called since its creation. Note that if the hessian is implemented by a finite difference method, the hessian calls number is equal to 0 and the different calls are counted in the evaluation calls number.
- getInputDescription()¶
Accessor to the description of the input vector.
- Returns:
- description
Description
Description of the input vector.
- description
Examples
>>> import openturns as ot >>> f = ot.SymbolicFunction(['x1', 'x2'], ... ['2 * x1^2 + x1 + 8 * x2 + 4 * cos(x1) * x2 + 6']) >>> print(f.getInputDescription()) [x1,x2]
- getInputDimension()¶
Accessor to the dimension of the input vector.
- Returns:
- inputDimint
Dimension of the input vector
.
Examples
>>> import openturns as ot >>> f = ot.SymbolicFunction(['x1', 'x2'], ... ['2 * x1^2 + x1 + 8 * x2 + 4 * cos(x1) * x2 + 6']) >>> print(f.getInputDimension()) 2
- getInputHistory()¶
Get the input sample.
- Returns:
- inputSample
Sample
Input points which have been evaluated.
- inputSample
- getMarginal(*args)¶
Accessor to marginal.
- Parameters:
- indicesint or list of ints
Set of indices for which the marginal is extracted.
- Returns:
- marginal
Function
Function corresponding to either
or
, with
and
.
- marginal
- getName()¶
Accessor to the object’s name.
- Returns:
- namestr
The name of the object.
- getOutputDescription()¶
Accessor to the description of the output vector.
- Returns:
- description
Description
Description of the output vector.
- description
Examples
>>> import openturns as ot >>> f = ot.SymbolicFunction(['x1', 'x2'], ... ['2 * x1^2 + x1 + 8 * x2 + 4 * cos(x1) * x2 + 6']) >>> print(f.getOutputDescription()) [y0]
- getOutputDimension()¶
Accessor to the number of the outputs.
- Returns:
- number_outputsint
Dimension of the output vector
.
Examples
>>> import openturns as ot >>> f = ot.SymbolicFunction(['x1', 'x2'], ... ['2 * x1^2 + x1 + 8 * x2 + 4 * cos(x1) * x2 + 6']) >>> print(f.getOutputDimension()) 1
- getOutputHistory()¶
Get the output sample.
- Returns:
- outputSample
Sample
Output points which have been evaluated.
- outputSample
- getParameterDescription()¶
Accessor to the parameter description.
- Returns:
- parameter
Description
The parameter description.
- parameter
- getParameterDimension()¶
Accessor to the dimension of the parameter.
- Returns:
- parameterDimensionint
Dimension of the parameter.
- gradient(inP)¶
Return the Jacobian transposed matrix of the function at a point.
- Parameters:
- pointsequence of float
Point where the Jacobian transposed matrix is calculated.
- Returns:
- gradient
Matrix
The Jacobian transposed matrix of the function at point.
- gradient
Examples
>>> import openturns as ot >>> f = ot.SymbolicFunction(['x1', 'x2'], ... ['2 * x1^2 + x1 + 8 * x2 + 4 * cos(x1) * x2 + 6','x1 + x2']) >>> print(f.gradient([3.14, 4])) [[ 13.5345 1 ] [ 4.00001 1 ]]
- hasName()¶
Test if the object is named.
- Returns:
- hasNamebool
True if the name is not empty.
- hessian(inP)¶
Return the hessian of the function at a point.
- Parameters:
- pointsequence of float
Point where the hessian of the function is calculated.
- Returns:
- hessian
SymmetricTensor
Hessian of the function at point.
- hessian
Examples
>>> import openturns as ot >>> f = ot.SymbolicFunction(['x1', 'x2'], ... ['2 * x1^2 + x1 + 8 * x2 + 4 * cos(x1) * x2 + 6','x1 + x2']) >>> print(f.hessian([3.14, 4])) sheet #0 [[ 20 -0.00637061 ] [ -0.00637061 0 ]] sheet #1 [[ 0 0 ] [ 0 0 ]]
- isCacheEnabled()¶
Test whether the cache mechanism is enabled or not.
- Returns:
- isCacheEnabledbool
Flag telling whether the cache mechanism is enabled. It is enabled by default.
- isHistoryEnabled()¶
Test whether the history mechanism is enabled or not.
- Returns:
- isHistoryEnabledbool
Flag telling whether the history mechanism is enabled.
- isLinear()¶
Accessor to the linearity of the function.
- Returns:
- linearbool
True if the function is linear, False otherwise.
- isLinearlyDependent(index)¶
Accessor to the linearity of the function with regard to a specific variable.
- Parameters:
- indexint
The index of the variable with regard to which linearity is evaluated.
- Returns:
- linearbool
True if the function is linearly dependent on the specified variable, False otherwise.
- parameterGradient(inP)¶
Accessor to the gradient against the parameter.
- Returns:
- gradient
Matrix
The gradient.
- gradient
- setDescription(description)¶
Accessor to the description of the inputs and outputs.
- Parameters:
- descriptionsequence of str
Description of the inputs and the outputs.
Examples
>>> import openturns as ot >>> f = ot.SymbolicFunction(['x1', 'x2'], ... ['2 * x1^2 + x1 + 8 * x2 + 4 * cos(x1) * x2 + 6']) >>> print(f.getDescription()) [x1,x2,y0] >>> f.setDescription(['a','b','y']) >>> print(f.getDescription()) [a,b,y]
- setEvaluation(evaluation)¶
Accessor to the evaluation function.
- Parameters:
- function
EvaluationImplementation
The evaluation function.
- function
- setGradient(gradient)¶
Accessor to the gradient function.
- Parameters:
- gradient_function
GradientImplementation
The gradient function.
- gradient_function
Examples
>>> import openturns as ot >>> f = ot.SymbolicFunction(['x1', 'x2'], ... ['2 * x1^2 + x1 + 8 * x2 + 4 * cos(x1) * x2 + 6']) >>> f.setGradient(ot.CenteredFiniteDifferenceGradient( ... ot.ResourceMap.GetAsScalar('CenteredFiniteDifferenceGradient-DefaultEpsilon'), ... f.getEvaluation()))
- setHessian(hessian)¶
Accessor to the hessian function.
- Parameters:
- hessian_function
HessianImplementation
The hessian function.
- hessian_function
Examples
>>> import openturns as ot >>> f = ot.SymbolicFunction(['x1', 'x2'], ... ['2 * x1^2 + x1 + 8 * x2 + 4 * cos(x1) * x2 + 6']) >>> f.setHessian(ot.CenteredFiniteDifferenceHessian( ... ot.ResourceMap.GetAsScalar('CenteredFiniteDifferenceHessian-DefaultEpsilon'), ... f.getEvaluation()))
- setInputDescription(inputDescription)¶
Accessor to the description of the input vector.
- Parameters:
- description
Description
Description of the input vector.
- description
- setName(name)¶
Accessor to the object’s name.
- Parameters:
- namestr
The name of the object.
- setOutputDescription(outputDescription)¶
Accessor to the description of the output vector.
- Parameters:
- description
Description
Description of the output vector.
- description
- setParameter(parameter)¶
Accessor to the parameter values.
- Parameters:
- parametersequence of float
The parameter values.
- setParameterDescription(description)¶
Accessor to the parameter description.
- Parameters:
- parameter
Description
The parameter description.
- parameter
- setStopCallback(callBack, state=None)¶
Set up a stop callback.
Can be used to programmatically stop an evaluation.
- Parameters:
- callbackcallable
Returns a bool deciding whether to stop or continue.
Examples using the class¶
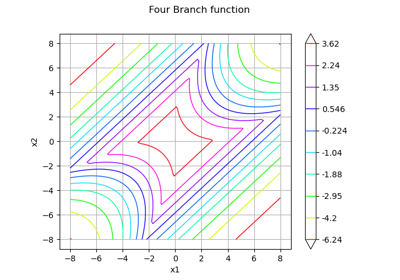
Non parametric Adaptive Importance Sampling (NAIS)
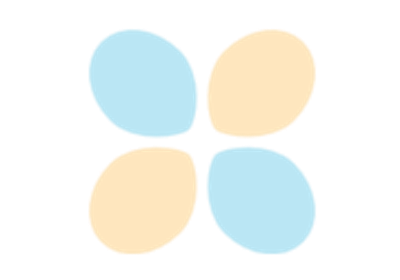
Defining Python and symbolic functions: a quick start introduction to functions
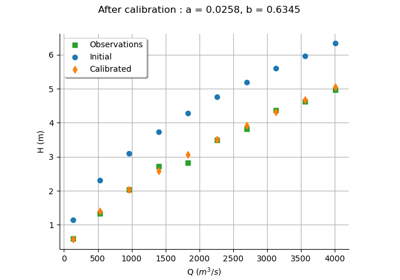
Calibrate a parametric model: a quick-start guide to calibration