Note
Click here to download the full example code
Calibration of the logistic model¶
We present a calibration study of the logistic growth model defined here.
Analysis of the data¶
import openturns as ot
import numpy as np
import openturns.viewer as viewer
from matplotlib import pylab as plt
ot.Log.Show(ot.Log.NONE)
We load the logistic model from the usecases module :
from openturns.usecases import logistic_model as logistic_model
lm = logistic_model.LogisticModel()
The data is based on 22 dates from 1790 to 2000. The observation points are stored in the data field :
observedSample = lm.data
nbobs = observedSample.getSize()
nbobs
Out:
22
timeObservations = observedSample[:,0]
timeObservations[0:5]
populationObservations = observedSample[:,1]
populationObservations[0:5]
graph = ot.Graph('', 'Time (years)', 'Population (Millions)', True, 'topleft')
cloud = ot.Cloud(timeObservations, populationObservations)
cloud.setLegend("Observations")
graph.add(cloud)
view = viewer.View(graph)
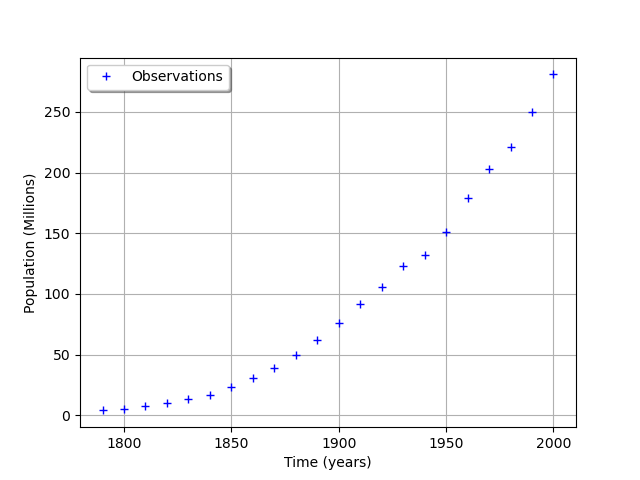
We consider the times and populations as dimension 22 vectors. The logisticModel function takes a dimension 24 vector as input and returns a dimension 22 vector. The first 22 components of the input vector contains the dates and the remaining 2 components are and
.
nbdates = 22
def logisticModel(X):
t = [X[i] for i in range(nbdates)]
a = X[22]
c = X[23]
t0 = 1790.
y0 = 3.9e6
b = np.exp(c)
y = [0.0] * nbdates
for i in range(nbdates):
y[i] = a*y0/(b*y0+(a-b*y0)*np.exp(-a*(t[i]-t0)))
z = [yi/1.e6 for yi in y] # Convert into millions
return z
logisticModelPy = ot.PythonFunction(24, nbdates, logisticModel)
The reference values of the parameters.
Because is so small with respect to
, we use the logarithm.
np.log(1.5587e-10)
Out:
-22.581998789427587
a=0.03134
c=-22.58
thetaPrior = [a,c]
logisticParametric = ot.ParametricFunction(logisticModelPy,[22,23],thetaPrior)
Check that we can evaluate the parametric function.
populationPredicted = logisticParametric(timeObservations.asPoint())
populationPredicted
graph = ot.Graph('', 'Time (years)', 'Population (Millions)', True, 'topleft')
# Observations
cloud = ot.Cloud(timeObservations, populationObservations)
cloud.setLegend("Observations")
cloud.setColor("blue")
graph.add(cloud)
# Predictions
cloud = ot.Cloud(timeObservations.asPoint(), populationPredicted)
cloud.setLegend("Predictions")
cloud.setColor("green")
graph.add(cloud)
view = viewer.View(graph)
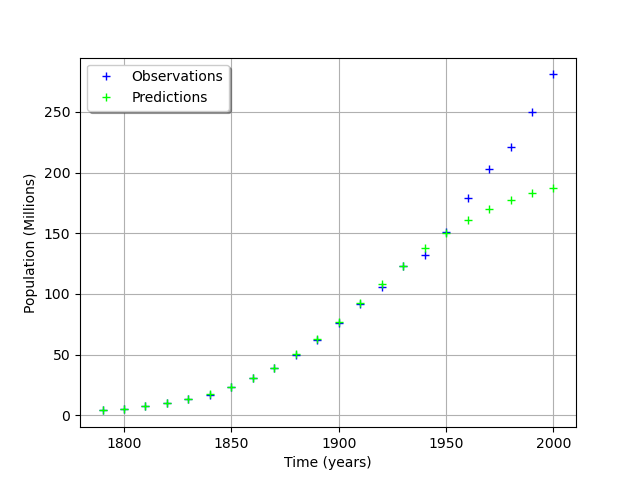
We see that the fit is not good: the observations continue to grow after 1950, while the growth of the prediction seem to fade.
Calibration with linear least squares¶
timeObservationsVector = ot.Sample([[timeObservations[i,0] for i in range(nbobs)]])
timeObservationsVector[0:10]
populationObservationsVector = ot.Sample([[populationObservations[i, 0] for i in range(nbobs)]])
populationObservationsVector[0:10]
The reference values of the parameters.
a=0.03134
c=-22.58
thetaPrior = [a,c]
logisticParametric = ot.ParametricFunction(logisticModelPy,[22,23],thetaPrior)
Check that we can evaluate the parametric function.
populationPredicted = logisticParametric(timeObservationsVector)
populationPredicted[0:10]
Calibration¶
algo = ot.LinearLeastSquaresCalibration(logisticParametric, timeObservationsVector, populationObservationsVector, thetaPrior)
algo.run()
calibrationResult = algo.getResult()
thetaMAP = calibrationResult.getParameterMAP()
thetaMAP
thetaPosterior = calibrationResult.getParameterPosterior()
thetaPosterior.computeBilateralConfidenceIntervalWithMarginalProbability(0.95)[0]
transpose samples to interpret several observations instead of several input/outputs as it is a field model
if calibrationResult.getInputObservations().getSize() == 1:
calibrationResult.setInputObservations([timeObservations[i] for i in range(nbdates)])
calibrationResult.setOutputObservations([populationObservations[i] for i in range(nbdates)])
outputAtPrior = [[calibrationResult.getOutputAtPriorMean()[0, i]] for i in range(nbdates)]
outputAtPosterior = [[calibrationResult.getOutputAtPosteriorMean()[0, i]] for i in range(nbdates)]
calibrationResult.setOutputAtPriorAndPosteriorMean(outputAtPrior, outputAtPosterior)
graph = calibrationResult.drawObservationsVsInputs()
view = viewer.View(graph)
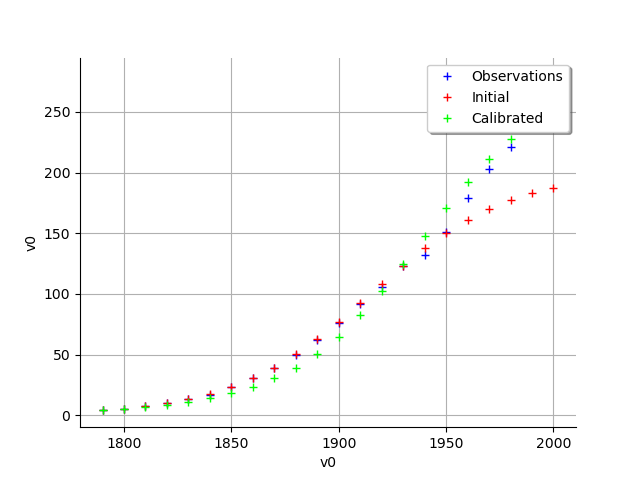
graph = calibrationResult.drawObservationsVsInputs()
view = viewer.View(graph)
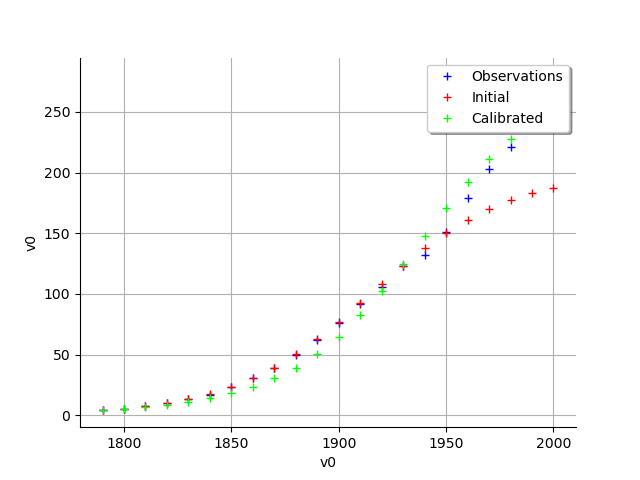
graph = calibrationResult.drawObservationsVsPredictions()
view = viewer.View(graph)
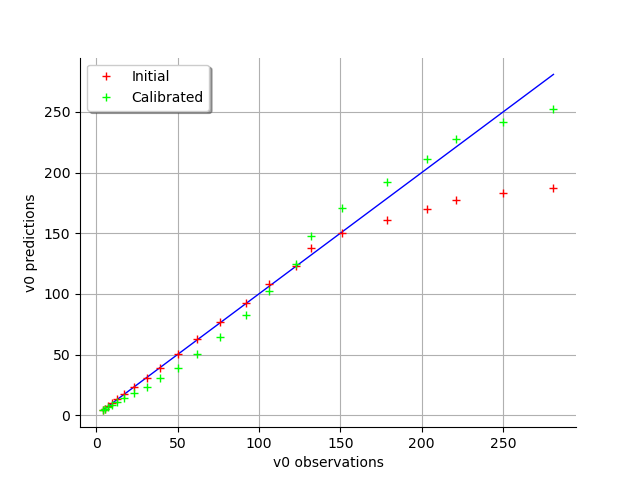
graph = calibrationResult.drawResiduals()
view = viewer.View(graph)
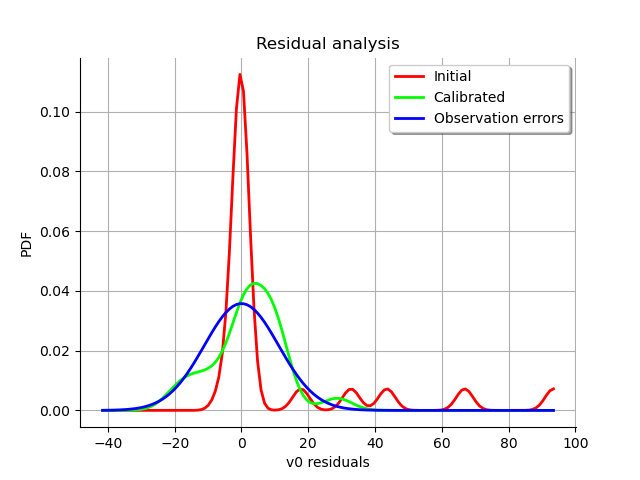
graph = calibrationResult.drawParameterDistributions()
view = viewer.View(graph)
plt.show()
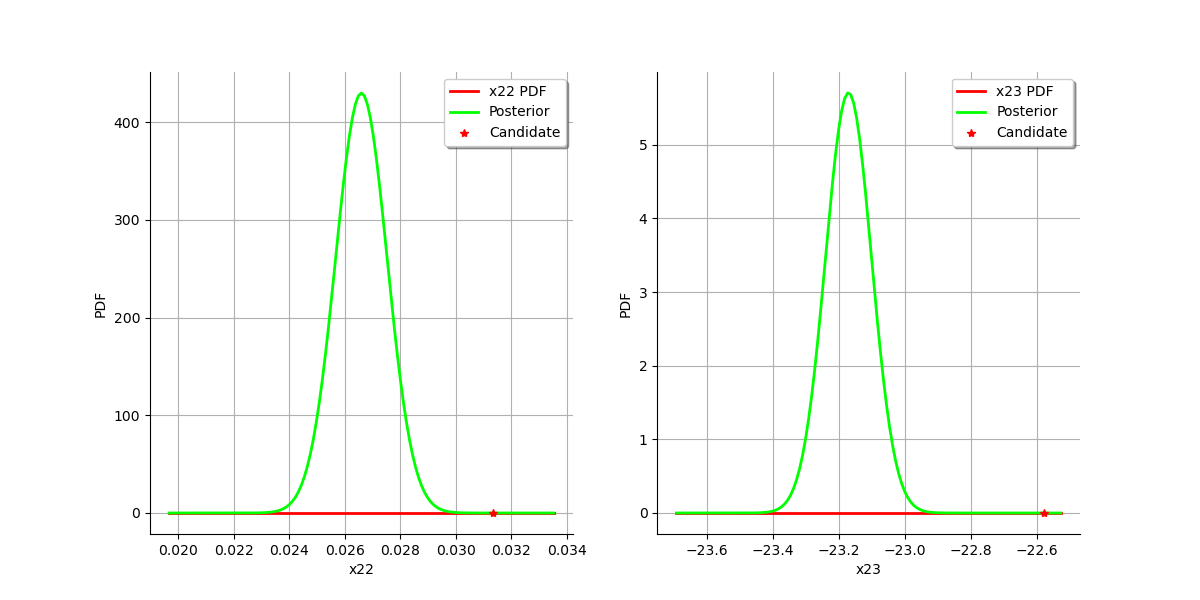
Total running time of the script: ( 0 minutes 2.387 seconds)