Note
Click here to download the full example code
Function manipulation¶
In this example we are going to exhibit some of the generic function services such as:
to ask the dimension of its input and output vectors
to evaluate itself, its gradient and hessian
to set a finite difference method for the evaluation of the gradient or the hessian
to evaluate the number of times the function or its gradient or its hessian have been evaluated
to disable or enable (enabled by default) the history mechanism
to disable or enable the cache mechanism
to get all the values evaluated by the function and the associated inputs with the methods
to clear the history
to ask the description of its input and output vectors
to extract output components
to get a graphical representation
import openturns as ot
import openturns.viewer as viewer
from matplotlib import pylab as plt
import math as m
ot.Log.Show(ot.Log.NONE)
Create a vectorial function R ^n –> R^p for example R^2 –> R^2
f = ot.SymbolicFunction(['x1', 'x2'], ['1+2*x1+x2', '2+x1+2*x2'])
# Create a scalar function R --> R
func1 = ot.SymbolicFunction(['x'], ['x^2'])
# Create another function R^2 --> R
func2 = ot.SymbolicFunction(['x', 'y'], ['x*y'])
# Create a vectorial function R ^3 --> R^2
func3 = ot.SymbolicFunction(
['x1', 'x2', 'x3'], ['1+2*x1+x2+x3^3', '2+sin(x1+2*x2)-sin(x3) * x3^4'])
# Create a second vectorial function R ^n --> R^p
# for example R^2 --> R^2
g = ot.SymbolicFunction(['x1', 'x2'], ['x1+x2', 'x1^2+2*x2^2'])
def python_eval(X):
a, b = X
y = a+b
return [y]
func4 = ot.PythonFunction(2, 1, python_eval)
Ask for the dimension of the input and output vectors
print(f.getInputDimension())
print(f.getOutputDimension())
Out:
2
2
Enable the history mechanism
f = ot.MemoizeFunction(f)
Evaluate the function at a particular point
x = [1.0] * f.getInputDimension()
y = f(x)
print('x=', x, 'y=', y)
Out:
x= [1.0, 1.0] y= [4,5]
Get the history
samplex = f.getInputHistory()
sampley = f.getOutputHistory()
print('evaluation history = ', samplex, sampley)
Out:
evaluation history = 0 : [ 1 1 ] 0 : [ 4 5 ]
Clear the history mechanism
f.clearHistory()
Disable the history mechanism
f.disableHistory()
Enable the cache mechanism
f4 = ot.MemoizeFunction(func4)
f4.enableCache()
for i in range(10):
f4(x)
Get the number of times cached values are reused
f4.getCacheHits()
Out:
9
Evaluate the gradient of the function at a particular point
gradientMatrix = f.gradient(x)
gradientMatrix
Evaluate the hessian of the function at a particular point
hessianMatrix = f.hessian(x)
hessianMatrix
Change the gradient method to a non centered finite difference method
step = [1e-7] * f.getInputDimension()
gradient = ot.NonCenteredFiniteDifferenceGradient(step, f.getEvaluation())
f.setGradient(gradient)
gradient
Change the hessian method to a centered finite difference method
step = [1e-7] * f.getInputDimension()
hessian = ot.CenteredFiniteDifferenceHessian(step, f.getEvaluation())
f.setHessian(hessian)
hessian
Get the number of times the function has been evaluated
f.getEvaluationCallsNumber()
Out:
1
Get the number of times the gradient has been evaluated
f.getGradientCallsNumber()
Out:
0
Get the number of times the hessian has been evaluated
f.getHessianCallsNumber()
Out:
0
Get the component i
f.getMarginal(1)
Compose two functions : h = f o g
ot.ComposedFunction(f, g)
Get the valid symbolic constants
ot.SymbolicFunction.GetValidConstants()
Graph 1 : z –> f_2(x_0,y_0,z) for z in [-1.5, 1.5] and y_0 = 2. and z_0 = 2.5 Specify the input component that varies Care : numerotation begins at 0
inputMarg = 2
# Give its variation intervall
zMin = -1.5
zMax = 1.5
# Give the coordinates of the fixed input components
centralPt = [1.0, 2.0, 2.5]
# Specify the output marginal function
# Care : numerotation begins at 0
outputMarg = 1
# Specify the point number of the final curve
ptNb = 101
# Draw the curve!
graph = func3.draw(inputMarg, outputMarg, centralPt, zMin, zMax, ptNb)
view = viewer.View(graph)
![y1 as a function of x3 around [1,2,2.5]](../../_images/sphx_glr_plot_function_manipulation_001.png)
Graph 2 : (x,z) –> f_1(x,y_0,z) for x in [-1.5, 1.5], z in [-2.5, 2.5] and y_0 = 2.5 Specify the input components that vary
firstInputMarg = 0
secondInputMarg = 2
# Give their variation interval
inputMin2 = [-1.5, -2.5]
inputMax2 = [1.5, 2.5]
# Give the coordinates of the fixed input components
centralPt = [0.0, 2., 2.5]
# Specify the output marginal function
outputMarg = 1
# Specify the point number of the final curve
ptNb = [101, 101]
graph = func3.draw(firstInputMarg, secondInputMarg,
outputMarg, centralPt, inputMin2, inputMax2, ptNb)
view = viewer.View(graph)
![y1 as a function of (x1,x3) around [0,2,2.5]](../../_images/sphx_glr_plot_function_manipulation_002.png)
Graph 3 : simplified method for x –> func1(x) for x in [-1.5, 1.5] Give the variation interval
xMin3 = -1.5
xMax3 = 1.5
# Specify the point number of the final curve
ptNb = 101
# Draw the curve!
graph = func1.draw(xMin3, xMax3, ptNb)
view = viewer.View(graph)
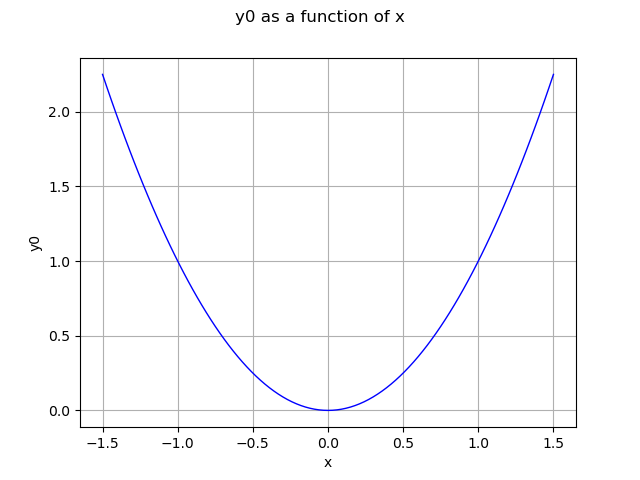
Graph 4 : (x,y) –> func2(x,y) for x in [-1.5, 1.5], y in [-2.5, 2.5] Give their variation interval
inputMin4 = [-1.5, -2.5]
inputMax4 = [1.5, 2.5]
# Give the coordinates of the fixed input components
centralPt = [0.0, 2., 2.5]
# Specify the output marginal function
outputMarg = 1
# Specify the point number of the final curve
ptNb = [101, 101]
graph = func2.draw(inputMin4, inputMax4, ptNb)
view = viewer.View(graph)
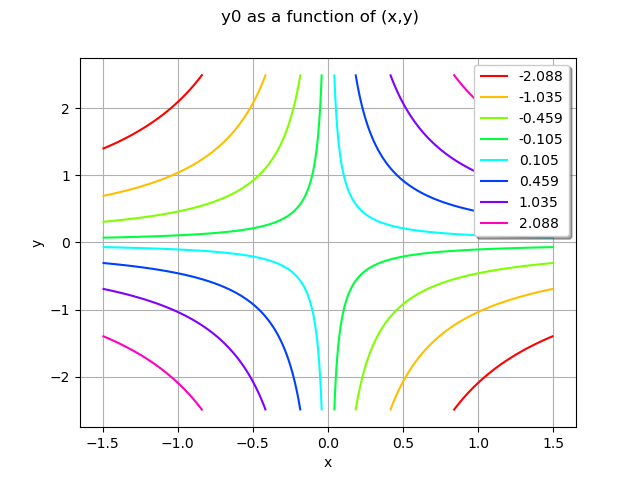
Total running time of the script: ( 0 minutes 0.313 seconds)