DirectionalSampling¶
- class DirectionalSampling(*args)¶
Directional sampling algorithm.
Refer to Directional Simulation.
- Available constructors:
DirectionalSampling(event)
DirectionalSampling(event, rootStrategy, samplingStrategy)
- Parameters:
- event
RandomVector
Event we are computing the probability of.
- rootStrategy
RootStrategy
Strategy used to evaluate the frontiers of the event along each direction in the standard space.
By default
SafeAndSlow
.- samplingStrategy
SamplingStrategy
Strategy used to sample directions in the standard space.
By default
RandomDirection
.
- event
Methods
drawProbabilityConvergence
(*args)Draw the probability convergence at a given level.
Accessor to the block size.
Accessor to the object's name.
Accessor to the convergence strategy.
getEvent
()Accessor to the event.
Accessor to the maximum coefficient of variation.
Accessor to the maximum iterations number.
Accessor to the maximum standard deviation.
Accessor to the maximum duration.
getName
()Accessor to the object's name.
Accessor to the results.
Get the root strategy.
Get the direction sampling strategy.
hasName
()Test if the object is named.
run
()Launch simulation.
setBlockSize
(blockSize)Accessor to the block size.
setConvergenceStrategy
(convergenceStrategy)Accessor to the convergence strategy.
Accessor to the maximum coefficient of variation.
setMaximumOuterSampling
(maximumOuterSampling)Accessor to the maximum iterations number.
Accessor to the maximum standard deviation.
setMaximumTimeDuration
(maximumTimeDuration)Accessor to the maximum duration.
setName
(name)Accessor to the object's name.
setProgressCallback
(*args)Set up a progress callback.
setRootStrategy
(rootStrategy)Set the root strategy.
setSamplingStrategy
(samplingStrategy)Set the direction sampling strategy.
setStopCallback
(*args)Set up a stop callback.
Notes
Let
be a random vector of dimension
, distributed according to the measure
, and
be the limit state function (where we only wrote the random input parameters). We define the event
by:
The directional sampling algorithm estimates the probability of the event
:
The directional sampling algorithm is a variance reduction sampling method. As it is performed in the standard space, it uses the iso-probabilistic transformation associated to
(see Isoprobabilistic transformations to get more details).
Let
be the iso-probabilistic transformation:
. The random vector
follows a spherical distribution, which means that it is written as:
where
is a scalar random variable following the radial distribution,
is a random vector that follows the uniform distribution on the unit sphere of dimension
, denoted by
, and
are independent.
Then the probability
can be written as:
For a given direction
, let
be:
Then, we have:
As the radial distribution of
is known, we can evaluate exactly the probability
as soon as we know the direction
.
The integral with respect to the directions is evaluated using a sampling method, defined by the class:~openturns.SamplingStrategy.
The directional sampling algorithm follows the steps:
A sample is generated from the uniform distribution on
: from this sample, some directions are defined, according to the specified
SamplingStrategy
. We denote bythe sample of the directions;
Along each direction
, we find the frontiers of
, i.e. the solutions of
according to the
RootStrategy
. From these solutions, we deduce the subsetthat belongs to
;
For each subset
, we compute the probability
.
The probability
is computed from all the
probabilities. If
directions have been used, then:
Examples
>>> import openturns as ot >>> ot.RandomGenerator.SetSeed(0)
Create the output random vector
:
>>> myFunction = ot.SymbolicFunction(['E', 'F', 'L', 'I'], ['-F*L^3/(3*E*I)']) >>> myDistribution = ot.Normal([50.0, 1.0, 10.0, 5.0], [1.0]*4, ot.IdentityMatrix(4)) >>> input_RV = ot.RandomVector(myDistribution) >>> output_RV = ot.CompositeRandomVector(myFunction, input_RV)
Create the event
:
>>> threshold = -3.0 >>> event = ot.ThresholdEvent(output_RV, ot.Less(), -3.0)
Create the directional sampling algorithm and the convergence criteria:
>>> myAlgo = ot.DirectionalSampling(event, ot.MediumSafe(), ot.OrthogonalDirection()) >>> myAlgo.setMaximumOuterSampling(150) >>> myAlgo.setBlockSize(4) >>> myAlgo.setMaximumCoefficientOfVariation(0.1) >>> # Perform the simulation
Run the algorithm and extract the estimated probability:
>>> myAlgo.run() >>> print('Probability estimate=%.6f' % myAlgo.getResult().getProbabilityEstimate()) Probability estimate=0.169716
- __init__(*args)¶
- drawProbabilityConvergence(*args)¶
Draw the probability convergence at a given level.
- Parameters:
- levelfloat, optional
The probability convergence is drawn at this given confidence length level. By default level is 0.95.
- Returns:
- grapha
Graph
probability convergence graph
- grapha
- getBlockSize()¶
Accessor to the block size.
- Returns:
- blockSizeint
Number of simultaneous evaluations of the limit-state function. It is set by default to 1.
- getClassName()¶
Accessor to the object’s name.
- Returns:
- class_namestr
The object class name (object.__class__.__name__).
- getConvergenceStrategy()¶
Accessor to the convergence strategy.
- Returns:
- storage_strategy
HistoryStrategy
Storage strategy used to store the values of the probability estimator and its variance during the simulation algorithm.
- storage_strategy
- getEvent()¶
Accessor to the event.
- Returns:
- event
RandomVector
Event we want to evaluate the probability.
- event
- getMaximumCoefficientOfVariation()¶
Accessor to the maximum coefficient of variation.
- Returns:
- coefficientfloat
Maximum coefficient of variation of the simulated sample.
- getMaximumOuterSampling()¶
Accessor to the maximum iterations number.
- Returns:
- outerSamplingint
Maximum number of iterations, each iteration performing a block of evaluations.
- getMaximumStandardDeviation()¶
Accessor to the maximum standard deviation.
- Returns:
- sigmafloat,
Maximum standard deviation of the estimator.
- sigmafloat,
- getMaximumTimeDuration()¶
Accessor to the maximum duration.
- Returns:
- maximumTimeDurationfloat
Maximum optimization duration in seconds.
- getName()¶
Accessor to the object’s name.
- Returns:
- namestr
The name of the object.
- getResult()¶
Accessor to the results.
- Returns:
- results
SimulationResult
Structure containing all the results obtained after simulation and created by the method
run()
.
- results
- getRootStrategy()¶
Get the root strategy.
- Returns:
- strategy
RootStrategy
Root strategy used to evaluate the frontiers of the event along each direction in the standard space.
- strategy
- getSamplingStrategy()¶
Get the direction sampling strategy.
- Returns:
- strategy
SamplingStrategy
Direction sampling strategy used to sample directions in the standard space.
- strategy
- hasName()¶
Test if the object is named.
- Returns:
- hasNamebool
True if the name is not empty.
- run()¶
Launch simulation.
See also
Notes
It launches the simulation and creates a
SimulationResult
, structure containing all the results obtained after simulation. It computes the probability of occurrence of the given event by computing the empirical mean of a sample of size at most outerSampling * blockSize, this sample being built by blocks of size blockSize. It allows one to use efficiently the distribution of the computation as well as it allows one to deal with a sample sizeby a combination of blockSize and outerSampling.
- setBlockSize(blockSize)¶
Accessor to the block size.
- Parameters:
- blockSizeint,
Number of simultaneous evaluations of the limit-state function. It is set by default to 1.
- blockSizeint,
Notes
If the function supports batch evaluations this parameter can be set to the number of available CPUs to benefit from parallel execution (multithreading, multiprocessing, …); except for the Directional Sampling, where we recommend to set it to 1. It also decides the frequency of the stopping criteria and progress callbacks update as they are called once the whole block of fonction evaluations is completed.
- setConvergenceStrategy(convergenceStrategy)¶
Accessor to the convergence strategy.
- Parameters:
- storage_strategy
HistoryStrategy
Storage strategy used to store the values of the probability estimator and its variance during the simulation algorithm.
- storage_strategy
- setMaximumCoefficientOfVariation(maximumCoefficientOfVariation)¶
Accessor to the maximum coefficient of variation.
- Parameters:
- coefficientfloat
Maximum coefficient of variation of the simulated sample.
- setMaximumOuterSampling(maximumOuterSampling)¶
Accessor to the maximum iterations number.
- Parameters:
- outerSamplingint
Maximum number of iterations, each iteration performing a block of evaluations.
- setMaximumStandardDeviation(maximumStandardDeviation)¶
Accessor to the maximum standard deviation.
- Parameters:
- sigmafloat,
Maximum standard deviation of the estimator.
- sigmafloat,
- setMaximumTimeDuration(maximumTimeDuration)¶
Accessor to the maximum duration.
- Parameters:
- maximumTimeDurationfloat
Maximum optimization duration in seconds.
- setName(name)¶
Accessor to the object’s name.
- Parameters:
- namestr
The name of the object.
- setProgressCallback(*args)¶
Set up a progress callback.
Can be used to programmatically report the progress of a simulation.
- Parameters:
- callbackcallable
Takes a float as argument as percentage of progress.
Examples
>>> import sys >>> import openturns as ot >>> experiment = ot.MonteCarloExperiment() >>> X = ot.RandomVector(ot.Normal()) >>> Y = ot.CompositeRandomVector(ot.SymbolicFunction(['X'], ['1.1*X']), X) >>> event = ot.ThresholdEvent(Y, ot.Less(), -2.0) >>> algo = ot.ProbabilitySimulationAlgorithm(event, experiment) >>> algo.setMaximumOuterSampling(100) >>> algo.setMaximumCoefficientOfVariation(-1.0) >>> def report_progress(progress): ... sys.stderr.write('-- progress=' + str(progress) + '%\n') >>> algo.setProgressCallback(report_progress) >>> algo.run()
- setRootStrategy(rootStrategy)¶
Set the root strategy.
- Parameters:
- strategy
RootStrategy
Root strategy to evaluate the frontiers of the event along each direction in the standard space.
- strategy
- setSamplingStrategy(samplingStrategy)¶
Set the direction sampling strategy.
- Parameters:
- strategy
SamplingStrategy
Direction sampling strategy used to sample directions in the standard space.
- strategy
- setStopCallback(*args)¶
Set up a stop callback.
Can be used to programmatically stop a simulation.
- Parameters:
- callbackcallable
Returns an int deciding whether to stop or continue.
Examples
Stop a Monte Carlo simulation algorithm using a time limit
>>> import openturns as ot >>> experiment = ot.MonteCarloExperiment() >>> X = ot.RandomVector(ot.Normal()) >>> Y = ot.CompositeRandomVector(ot.SymbolicFunction(['X'], ['1.1*X']), X) >>> event = ot.ThresholdEvent(Y, ot.Less(), -2.0) >>> algo = ot.ProbabilitySimulationAlgorithm(event, experiment) >>> algo.setMaximumOuterSampling(10000000) >>> algo.setMaximumCoefficientOfVariation(-1.0) >>> algo.setMaximumTimeDuration(0.1) >>> algo.run()
Examples using the class¶
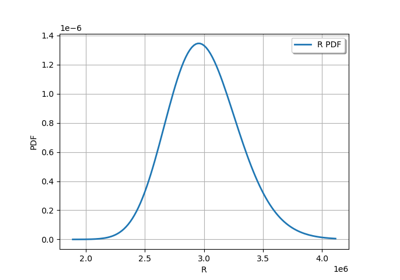
Axial stressed beam : comparing different methods to estimate a probability