ProbabilitySimulationAlgorithm¶
- class ProbabilitySimulationAlgorithm(*args)¶
Iterative sampling methods.
Refer to Monte Carlo simulation, Importance Simulation, Latin Hypercube Simulation, Quasi Monte Carlo.
- Available constructor:
ProbabilitySimulationAlgorithm(event, experiment, convergenceStrategy=ot.Compact())
ProbabilitySimulationAlgorithm(event, convergenceStrategy=ot.Compact())
- Parameters:
- event
RandomVector
The event we are computing the probability of, must be composite.
- experiment
WeightedExperiment
Sequential experiment
- convergenceStrategy
HistoryStrategy
, optional Storage strategy used to store the values of the probability estimator and its variance during the simulation algorithm.
- event
Methods
drawProbabilityConvergence
(*args)Draw the probability convergence at a given level.
Accessor to the block size.
Accessor to the object's name.
Accessor to the convergence strategy.
getEvent
()Accessor to the event.
Accessor to the experiment.
Accessor to the maximum coefficient of variation.
Accessor to the maximum iterations number.
Accessor to the maximum standard deviation.
Accessor to the maximum duration.
getName
()Accessor to the object's name.
Accessor to the results.
hasName
()Test if the object is named.
run
()Launch simulation.
setBlockSize
(blockSize)Accessor to the block size.
setConvergenceStrategy
(convergenceStrategy)Accessor to the convergence strategy.
setExperiment
(experiment)Accessor to the experiment.
Accessor to the maximum coefficient of variation.
setMaximumOuterSampling
(maximumOuterSampling)Accessor to the maximum iterations number.
Accessor to the maximum standard deviation.
setMaximumTimeDuration
(maximumTimeDuration)Accessor to the maximum duration.
setName
(name)Accessor to the object's name.
setProgressCallback
(*args)Set up a progress callback.
setStopCallback
(*args)Set up a stop callback.
See also
Notes
Using the probability distribution of a random vector
, we seek to evaluate the following probability:
Here,
is a random vector,
a deterministic vector,
the function known as limit state function which enables the definition of the event
If we have the set
of
independent samples of the random vector
, we can estimate
as follows:
where
describes the indicator function equal to 1 if
and equal to 0 otherwise; the idea here is in fact to estimate the required probability by the proportion of cases, among the
samples of
, for which the event
occurs.
By the law of large numbers, we know that this estimation converges to the required value
as the sample size
tends to infinity.
The Central Limit Theorem allows one to build an asymptotic confidence interval using the normal limit distribution as follows:
with
,
and
is the
-quantile of the standard normal distribution.
A ProbabilitySimulationAlgorithm object makes sense with the following sequential experiments:
The estimator built by Monte Carlo method is:
where
describes the indicator function equal to 1 if
and equal to 0 otherwise; the idea here is in fact to estimate the required probability by the proportion of cases, among the
samples of
, for which the event
occurs.
By the law of large numbers, we know that this estimation converges to the required value
as the sample size
tends to infinity.
The Central Limit Theorem allows one to build an asymptotic confidence interval using the normal limit distribution as follows:
with
,
and
is the
-quantile of the standard normal distribution.
The estimator built by Importance Sampling method is:
where:
is the total number of computations,
the random vectors
are independent, identically distributed and following the probability density function
.
Examples
Estimate a probability by Monte Carlo
>>> import openturns as ot >>> ot.RandomGenerator.SetSeed(0) >>> myFunction = ot.SymbolicFunction(['E', 'F', 'L', 'I'], ['-F*L^3/(3*E*I)']) >>> myDistribution = ot.Normal([50.0, 1.0, 10.0, 5.0], [1.0]*4, ot.IdentityMatrix(4)) >>> # We create a 'usual' RandomVector from the Distribution >>> vect = ot.RandomVector(myDistribution) >>> # We create a composite random vector >>> output = ot.CompositeRandomVector(myFunction, vect) >>> # We create an Event from this RandomVector >>> event = ot.ThresholdEvent(output, ot.Less(), -3.0) >>> # We create a Monte Carlo algorithm >>> experiment = ot.MonteCarloExperiment() >>> algo = ot.ProbabilitySimulationAlgorithm(event, experiment) >>> algo.setMaximumOuterSampling(150) >>> algo.setBlockSize(4) >>> algo.setMaximumCoefficientOfVariation(0.1) >>> # Perform the simulation >>> algo.run() >>> print('Probability estimate=%.6f' % algo.getResult().getProbabilityEstimate()) Probability estimate=0.140000
Estimate a probability by Importance Sampling
>>> ot.RandomGenerator.SetSeed(0) >>> # assume we obtained a design point from FORM >>> standardSpaceDesignPoint = [-0.0310363,0.841879,0.445462,-0.332318] >>> standardEvent = ot.StandardEvent(event) >>> importanceDensity = ot.Normal(standardSpaceDesignPoint, ot.CovarianceMatrix(4)) >>> experiment = ot.ImportanceSamplingExperiment(importanceDensity) >>> algo = ot.ProbabilitySimulationAlgorithm(standardEvent, experiment) >>> algo.setMaximumOuterSampling(150) >>> algo.setBlockSize(4) >>> algo.setMaximumCoefficientOfVariation(0.1) >>> # Perform the simulation >>> algo.run() >>> print('Probability estimate=%.6f' % algo.getResult().getProbabilityEstimate()) Probability estimate=0.153315
Estimate a probability by Quasi Monte Carlo
>>> ot.RandomGenerator.SetSeed(0) >>> experiment = ot.LowDiscrepancyExperiment() >>> algo = ot.ProbabilitySimulationAlgorithm(event, experiment) >>> algo.setMaximumOuterSampling(150) >>> algo.setBlockSize(4) >>> algo.setMaximumCoefficientOfVariation(0.1) >>> # Perform the simulation >>> algo.run() >>> print('Probability estimate=%.6f' % algo.getResult().getProbabilityEstimate()) Probability estimate=0.141667
Estimate a probability by Randomized Quasi Monte Carlo
>>> ot.RandomGenerator.SetSeed(0) >>> experiment = ot.LowDiscrepancyExperiment() >>> experiment.setRandomize(True) >>> algo = ot.ProbabilitySimulationAlgorithm(event, experiment) >>> algo.setMaximumOuterSampling(150) >>> algo.setBlockSize(4) >>> algo.setMaximumCoefficientOfVariation(0.1) >>> # Perform the simulation >>> algo.run() >>> print('Probability estimate=%.6f' % algo.getResult().getProbabilityEstimate()) Probability estimate=0.160000
Estimate a probability by Randomized LHS
>>> ot.RandomGenerator.SetSeed(0) >>> experiment = ot.LHSExperiment() >>> experiment.setAlwaysShuffle(True) >>> algo = ot.ProbabilitySimulationAlgorithm(event, experiment) >>> algo.setMaximumOuterSampling(150) >>> algo.setBlockSize(4) >>> algo.setMaximumCoefficientOfVariation(0.1) >>> # Perform the simulation >>> algo.run() >>> print('Probability estimate=%.6f' % algo.getResult().getProbabilityEstimate()) Probability estimate=0.140000
- __init__(*args)¶
- drawProbabilityConvergence(*args)¶
Draw the probability convergence at a given level.
- Parameters:
- levelfloat, optional
The probability convergence is drawn at this given confidence length level. By default level is 0.95.
- Returns:
- grapha
Graph
probability convergence graph
- grapha
- getBlockSize()¶
Accessor to the block size.
- Returns:
- blockSizeint
Number of simultaneous evaluations of the limit-state function. It is set by default to 1.
- getClassName()¶
Accessor to the object’s name.
- Returns:
- class_namestr
The object class name (object.__class__.__name__).
- getConvergenceStrategy()¶
Accessor to the convergence strategy.
- Returns:
- storage_strategy
HistoryStrategy
Storage strategy used to store the values of the probability estimator and its variance during the simulation algorithm.
- storage_strategy
- getEvent()¶
Accessor to the event.
- Returns:
- event
RandomVector
Event we want to evaluate the probability.
- event
- getExperiment()¶
Accessor to the experiment.
- Returns:
- experiment
WeightedExperiment
The experiment that is sampled at each iteration.
- experiment
- getMaximumCoefficientOfVariation()¶
Accessor to the maximum coefficient of variation.
- Returns:
- coefficientfloat
Maximum coefficient of variation of the simulated sample.
- getMaximumOuterSampling()¶
Accessor to the maximum iterations number.
- Returns:
- outerSamplingint
Maximum number of iterations, each iteration performing a block of evaluations.
- getMaximumStandardDeviation()¶
Accessor to the maximum standard deviation.
- Returns:
- sigmafloat,
Maximum standard deviation of the estimator.
- sigmafloat,
- getMaximumTimeDuration()¶
Accessor to the maximum duration.
- Returns:
- maximumTimeDurationfloat
Maximum optimization duration in seconds.
- getName()¶
Accessor to the object’s name.
- Returns:
- namestr
The name of the object.
- getResult()¶
Accessor to the results.
- Returns:
- results
SimulationResult
Structure containing all the results obtained after simulation and created by the method
run()
.
- results
- hasName()¶
Test if the object is named.
- Returns:
- hasNamebool
True if the name is not empty.
- run()¶
Launch simulation.
See also
Notes
It launches the simulation and creates a
SimulationResult
, structure containing all the results obtained after simulation. It computes the probability of occurrence of the given event by computing the empirical mean of a sample of size at most outerSampling * blockSize, this sample being built by blocks of size blockSize. It allows one to use efficiently the distribution of the computation as well as it allows one to deal with a sample sizeby a combination of blockSize and outerSampling.
- setBlockSize(blockSize)¶
Accessor to the block size.
- Parameters:
- blockSizeint,
Number of simultaneous evaluations of the limit-state function. It is set by default to 1.
- blockSizeint,
Notes
If the function supports batch evaluations this parameter can be set to the number of available CPUs to benefit from parallel execution (multithreading, multiprocessing, …); except for the Directional Sampling, where we recommend to set it to 1. It also decides the frequency of the stopping criteria and progress callbacks update as they are called once the whole block of fonction evaluations is completed.
- setConvergenceStrategy(convergenceStrategy)¶
Accessor to the convergence strategy.
- Parameters:
- storage_strategy
HistoryStrategy
Storage strategy used to store the values of the probability estimator and its variance during the simulation algorithm.
- storage_strategy
- setExperiment(experiment)¶
Accessor to the experiment.
- Parameters:
- experiment
WeightedExperiment
The experiment that is sampled at each iteration.
- experiment
- setMaximumCoefficientOfVariation(maximumCoefficientOfVariation)¶
Accessor to the maximum coefficient of variation.
- Parameters:
- coefficientfloat
Maximum coefficient of variation of the simulated sample.
- setMaximumOuterSampling(maximumOuterSampling)¶
Accessor to the maximum iterations number.
- Parameters:
- outerSamplingint
Maximum number of iterations, each iteration performing a block of evaluations.
- setMaximumStandardDeviation(maximumStandardDeviation)¶
Accessor to the maximum standard deviation.
- Parameters:
- sigmafloat,
Maximum standard deviation of the estimator.
- sigmafloat,
- setMaximumTimeDuration(maximumTimeDuration)¶
Accessor to the maximum duration.
- Parameters:
- maximumTimeDurationfloat
Maximum optimization duration in seconds.
- setName(name)¶
Accessor to the object’s name.
- Parameters:
- namestr
The name of the object.
- setProgressCallback(*args)¶
Set up a progress callback.
Can be used to programmatically report the progress of a simulation.
- Parameters:
- callbackcallable
Takes a float as argument as percentage of progress.
Examples
>>> import sys >>> import openturns as ot >>> experiment = ot.MonteCarloExperiment() >>> X = ot.RandomVector(ot.Normal()) >>> Y = ot.CompositeRandomVector(ot.SymbolicFunction(['X'], ['1.1*X']), X) >>> event = ot.ThresholdEvent(Y, ot.Less(), -2.0) >>> algo = ot.ProbabilitySimulationAlgorithm(event, experiment) >>> algo.setMaximumOuterSampling(100) >>> algo.setMaximumCoefficientOfVariation(-1.0) >>> def report_progress(progress): ... sys.stderr.write('-- progress=' + str(progress) + '%\n') >>> algo.setProgressCallback(report_progress) >>> algo.run()
- setStopCallback(*args)¶
Set up a stop callback.
Can be used to programmatically stop a simulation.
- Parameters:
- callbackcallable
Returns an int deciding whether to stop or continue.
Examples
Stop a Monte Carlo simulation algorithm using a time limit
>>> import openturns as ot >>> experiment = ot.MonteCarloExperiment() >>> X = ot.RandomVector(ot.Normal()) >>> Y = ot.CompositeRandomVector(ot.SymbolicFunction(['X'], ['1.1*X']), X) >>> event = ot.ThresholdEvent(Y, ot.Less(), -2.0) >>> algo = ot.ProbabilitySimulationAlgorithm(event, experiment) >>> algo.setMaximumOuterSampling(10000000) >>> algo.setMaximumCoefficientOfVariation(-1.0) >>> algo.setMaximumTimeDuration(0.1) >>> algo.run()
Examples using the class¶
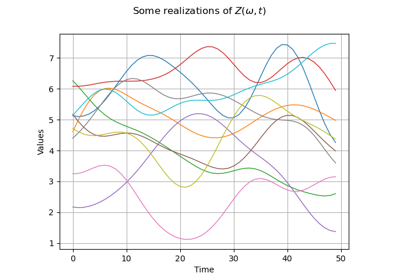
Create a process from random vectors and processes
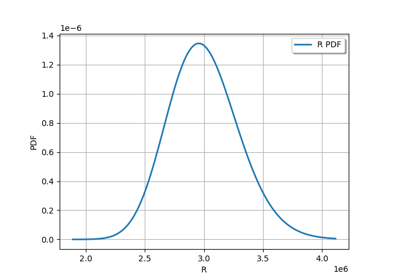
Estimate a probability with Monte-Carlo on axial stressed beam: a quick start guide to reliability
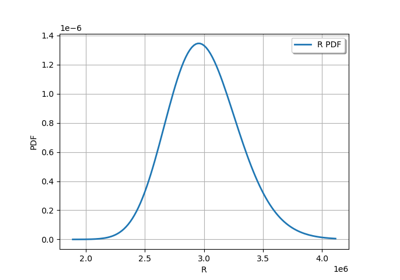
Axial stressed beam : comparing different methods to estimate a probability
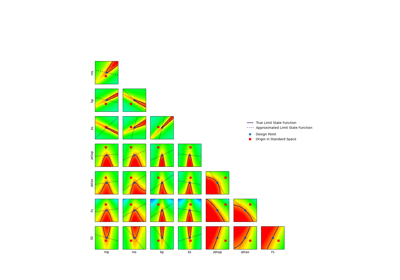
Using the FORM - SORM algorithms on a nonlinear function