Note
Go to the end to download the full example code.
Kriging: choose a polynomial trend¶
import openturns as ot
import openturns.viewer as otv
from matplotlib import pylab as plt
Introduction¶
In this example we present the polynomial trends which we may use in a kriging metamodel. This example focuses on three polynomial trends:
In the Kriging: choose a polynomial trend on the beam model example, we give another example of this method. In the Kriging: choose an arbitrary trend example, we show how to configure an arbitrary trend.
The model is the real function:
for any .
We consider the
MaternModel
covariance kernel
where is the vector of hyperparameters.
The covariance model is fixed but its parameters must be calibrated
depending on the data.
The kriging metamodel is:
where is the trend and
is a Gaussian process with zero mean and covariance model
.
The trend is deterministic and the Gaussian process is probabilistic but they both contribute to the metamodel.
A special feature of the kriging is the interpolation property: the metamodel is exact at the
training data.
covarianceModel = ot.SquaredExponential([1.0], [1.0])
Define the model¶
First, we define the MaternModel
covariance model.
covarianceModel = ot.MaternModel([1.0], [1.0], 2.5)
We define our exact model with a SymbolicFunction
.
model = ot.SymbolicFunction(["x"], ["x * sin(0.5 * x)"])
We use the following sample to train our metamodel.
xmin = 0.0
xmax = 10.0
ot.RandomGenerator.SetSeed(0)
nTrain = 8
Xtrain = ot.Uniform(xmin, xmax).getSample(nTrain).sort()
Xtrain
The values of the exact model are also needed for training.
Ytrain = model(Xtrain)
Ytrain
We shall test the model on a set of points based on a regular grid.
nTest = 100
step = (xmax - xmin) / (nTest - 1)
x_test = ot.RegularGrid(xmin, step, nTest).getVertices()
We draw the training points and the model at the testing points. We encapsulate it into a function to use it again later.
def plot_exact_model(color):
graph = ot.Graph("", "x", "", True, "")
y_test = model(x_test)
curveModel = ot.Curve(x_test, y_test)
curveModel.setLineStyle("solid")
curveModel.setColor(color)
curveModel.setLegend("Model")
graph.add(curveModel)
cloud = ot.Cloud(Xtrain, Ytrain)
cloud.setColor(color)
cloud.setPointStyle("fsquare")
cloud.setLegend("Data")
graph.add(cloud)
graph.setLegendPosition("bottom")
return graph
palette = ot.Drawable.BuildDefaultPalette(5)
graph = plot_exact_model(palette[0])
graph.setTitle("1D Kriging: exact model")
view = otv.View(graph)
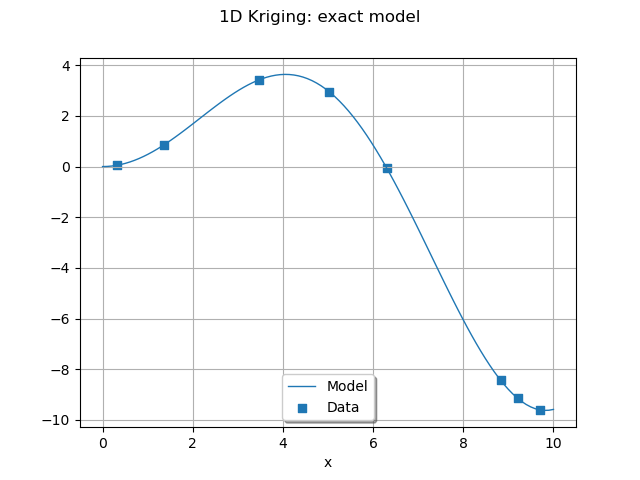
Scale the input training sample¶
We often have to apply a transform on the input data before performing the kriging.
This make the estimation of the hyperparameters of the kriging metamodel
easier for the optimization algorithm.
To do so we write a linear transform of our input data: we make it unit centered at its mean.
Then we fix the mean and the standard deviation to their values with the ParametricFunction
.
We build the inverse transform as well.
We first compute the mean and standard deviation of the input data.
mean = Xtrain.computeMean()[0]
stdDev = Xtrain.computeStandardDeviation()[0]
print("Xtrain, mean: %.3f" % mean)
print("Xtrain, standard deviation: %.3f" % stdDev)
Xtrain, mean: 5.526
Xtrain, standard deviation: 3.613
tf = ot.SymbolicFunction(["mu", "sigma", "x"], ["(x - mu) / sigma"])
itf = ot.SymbolicFunction(["mu", "sigma", "x"], ["sigma * x + mu"])
myInverseTransform = ot.ParametricFunction(itf, [0, 1], [mean, stdDev])
myTransform = ot.ParametricFunction(tf, [0, 1], [mean, stdDev])
Scale the input training sample.
scaledXtrain = myTransform(Xtrain)
scaledXtrain
Constant basis¶
In this paragraph we choose a basis constant for the kriging.
This trend only has one parameter which is the
value of the constant.
The basis is built with the ConstantBasisFactory
class.
dimension = 1
basis = ot.ConstantBasisFactory(dimension).build()
We build the kriging algorithm by giving it the transformed data, the output data, the covariance model and the basis.
algo = ot.KrigingAlgorithm(scaledXtrain, Ytrain, covarianceModel, basis)
We can run the algorithm and store the result.
algo.run()
result = algo.getResult()
The metamodel is the following ComposedFunction
.
metamodel = ot.ComposedFunction(result.getMetaModel(), myTransform)
Define a function to plot the metamodel
def plotMetamodel(x_test, krigingResult, myTransform, color):
scaled_x_test = myTransform(x_test)
metamodel = result.getMetaModel()
y_test = metamodel(scaled_x_test)
curve = ot.Curve(x_test, y_test)
curve.setLineStyle("dashed")
curve.setColor(color)
curve.setLegend("Metamodel")
return curve
We can draw the metamodel and the exact model on the same graph.
graph = plot_exact_model(palette[0])
graph.add(plotMetamodel(x_test, result, myTransform, palette[1]))
graph.setTitle("1D Kriging: exact model and metamodel")
view = otv.View(graph)
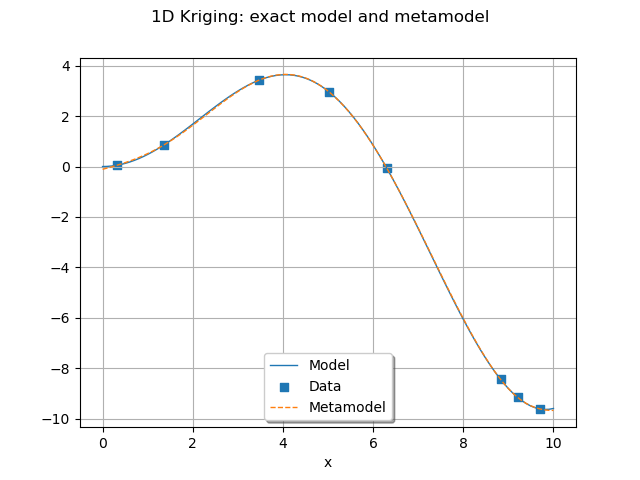
We can retrieve the calibrated trend coefficient with getTrendCoefficients()
.
c0 = result.getTrendCoefficients()
print("The trend is the curve m(x) = %.6e" % c0[0])
The trend is the curve m(x) = -2.726225e+00
We also observe the values of the hyperparameters of the trained covariance model.
rho = result.getCovarianceModel().getScale()[0]
print("Scale parameter: %.3e" % rho)
sigma = result.getCovarianceModel().getAmplitude()[0]
print("Amplitude parameter: %.3e" % sigma)
Scale parameter: 1.368e+00
Amplitude parameter: 6.077e+00
We build the trend from the coefficient.
constantTrend = ot.SymbolicFunction(["a", "x"], ["a"])
myTrend = ot.ParametricFunction(constantTrend, [0], [c0[0]])
Define a function to plot the trend
def plotTrend(x_test, myTrend, myTransform, color):
scale_x_test = myTransform(x_test)
y_test = myTrend(scale_x_test)
curve = ot.Curve(x_test, y_test)
curve.setLineStyle("dotdash")
curve.setColor(color)
curve.setLegend("Trend")
return curve
We draw the trend found by the kriging procedure.
graph.add(plotTrend(x_test, myTrend, myTransform, palette[2]))
graph.setTitle("1D Kriging with a constant trend")
view = otv.View(graph)
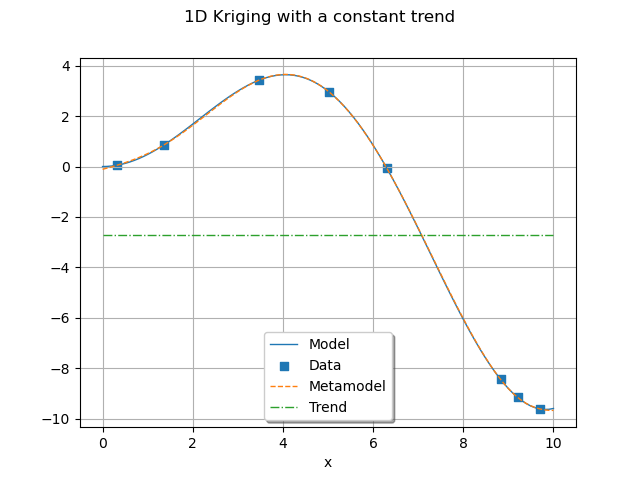
Create a function to plot confidence bounds.
def plotKrigingConfidenceBounds(krigingResult, x_test, myTransform, color, alpha=0.05):
bilateralCI = ot.Normal().computeBilateralConfidenceInterval(1.0 - alpha)
quantileAlpha = bilateralCI.getUpperBound()[0]
sqrt = ot.SymbolicFunction(["x"], ["sqrt(x)"])
n_test = x_test.getSize()
epsilon = ot.Sample(n_test, [1.0e-8])
scaled_x_test = myTransform(x_test)
conditionalVariance = (
krigingResult.getConditionalMarginalVariance(scaled_x_test) + epsilon
)
conditionalSigma = sqrt(conditionalVariance)
metamodel = krigingResult.getMetaModel()
y_test = metamodel(scaled_x_test)
dataLower = [
y_test[i, 0] - quantileAlpha * conditionalSigma[i, 0] for i in range(n_test)
]
dataUpper = [
y_test[i, 0] + quantileAlpha * conditionalSigma[i, 0] for i in range(n_test)
]
boundsPoly = ot.Polygon.FillBetween(x_test.asPoint(), dataLower, dataUpper)
boundsPoly.setColor(color)
boundsPoly.setLegend("%d%% C.I." % ((1.0 - alpha) * 100))
return boundsPoly
Plot a confidence interval.
graph.add(plotKrigingConfidenceBounds(result, x_test, myTransform, palette[3]))
graph.setTitle("1D Kriging with a constant trend")
view = otv.View(
graph,
legend_kw={"bbox_to_anchor": (1.0, 1.0), "loc": "upper left"},
figure_kw={"figsize": (7.0, 3.0)},
)
plt.subplots_adjust(right=0.6)
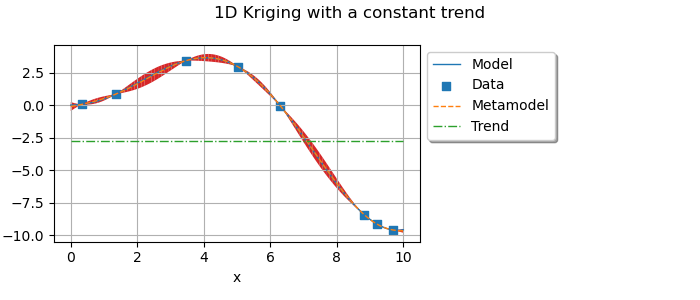
As expected with a constant basis, the trend obtained is an horizontal line.
Linear basis¶
With a linear basis, the vector space is defined by the basis : that is
all the lines of the form
where
and
are
real parameters.
basis = ot.LinearBasisFactory(dimension).build()
We run the kriging analysis and store the result.
algo = ot.KrigingAlgorithm(scaledXtrain, Ytrain, covarianceModel, basis)
algo.run()
result = algo.getResult()
metamodel = ot.ComposedFunction(result.getMetaModel(), myTransform)
We can draw the metamodel and the exact model on the same graph.
graph = plot_exact_model(palette[0])
graph.add(plotMetamodel(x_test, result, myTransform, palette[1]))
We can retrieve the calibrated trend coefficients with getTrendCoefficients()
.
c0 = result.getTrendCoefficients()
print("Trend is the curve m(X) = %.6e X + %.6e" % (c0[1], c0[0]))
Trend is the curve m(X) = -2.578407e+00 X + -3.020888e+00
We observe the values of the hyperparameters of the trained covariance model.
rho = result.getCovarianceModel().getScale()[0]
print("Scale parameter: %.3e" % rho)
sigma = result.getCovarianceModel().getAmplitude()[0]
print("Amplitude parameter: %.3e" % sigma)
Scale parameter: 1.100e+00
Amplitude parameter: 4.627e+00
We draw the linear trend that we are interested in.
linearTrend = ot.SymbolicFunction(["a", "b", "z"], ["a * z + b"])
myTrend = ot.ParametricFunction(linearTrend, [0, 1], [c0[1], c0[0]])
graph.add(plotTrend(x_test, myTrend, myTransform, palette[2]))
Add the 95% confidence interval.
graph.add(plotKrigingConfidenceBounds(result, x_test, myTransform, palette[3]))
graph.setTitle("1D Kriging with a linear trend")
view = otv.View(
graph,
legend_kw={"bbox_to_anchor": (1.0, 1.0), "loc": "upper left"},
figure_kw={"figsize": (7.0, 3.0)},
)
plt.subplots_adjust(right=0.6)
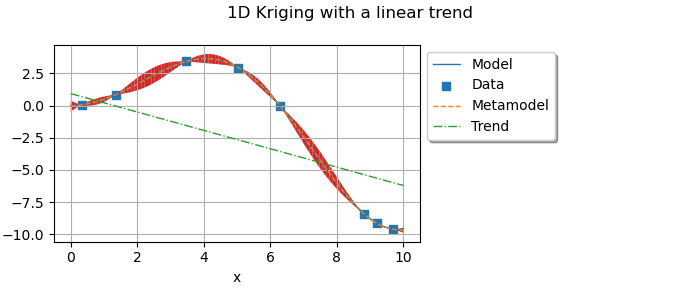
Quadratic basis¶
In this last paragraph we turn to the quadratic basis. All subsequent analysis should remain the same.
basis = ot.QuadraticBasisFactory(dimension).build()
We run the kriging analysis and store the result.
algo = ot.KrigingAlgorithm(scaledXtrain, Ytrain, covarianceModel, basis)
algo.run()
result = algo.getResult()
metamodel = ot.ComposedFunction(result.getMetaModel(), myTransform)
We can draw the metamodel and the exact model on the same graph.
graph = plot_exact_model(palette[0])
graph.add(plotMetamodel(x_test, result, myTransform, palette[1]))
We can retrieve the calibrated trend coefficients with getTrendCoefficients()
.
c0 = result.getTrendCoefficients()
print("Trend is the curve m(X) = %.6e Z**2 + %.6e Z + %.6e" % (c0[2], c0[1], c0[0]))
Trend is the curve m(X) = -4.583776e+00 Z**2 + -5.327795e+00 Z + 1.564663e+00
We observe the values of the hyperparameters of the trained covariance model.
rho = result.getCovarianceModel().getScale()[0]
print("Scale parameter: %.3e" % rho)
sigma = result.getCovarianceModel().getAmplitude()[0]
print("Amplitude parameter: %.3e" % sigma)
Scale parameter: 8.374e-02
Amplitude parameter: 9.440e-01
The quadratic trend obtained.
quadraticTrend = ot.SymbolicFunction(["a", "b", "c", "z"], ["a * z^2 + b * z + c"])
myTrend = ot.ParametricFunction(quadraticTrend, [0, 1, 2], [c0[2], c0[1], c0[0]])
Add the quadratic trend
y_test = myTrend(myTransform(x_test))
graph.add(plotTrend(x_test, myTrend, myTransform, palette[2]))
Add the 95% confidence interval.
sphinx_gallery_thumbnail_number = 6
graph.add(plotKrigingConfidenceBounds(result, x_test, myTransform, palette[3]))
graph.setTitle("1D Kriging with a quadratic trend")
view = otv.View(
graph,
legend_kw={"bbox_to_anchor": (1.0, 1.0), "loc": "upper left"},
figure_kw={"figsize": (7.0, 3.0)},
)
plt.subplots_adjust(right=0.6)
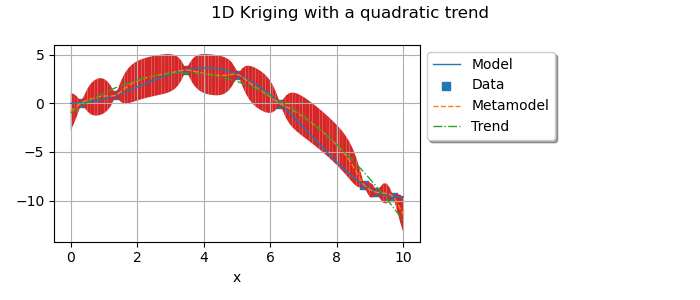
Display figures
otv.View.ShowAll()