Exploitation of simulation algorithm resultsΒΆ
In this example we are going to retrieve all the results proposed by probability simulation algorithms:
the probability estimate
the estimator variance
the confidence interval
the convergence graph of the estimator
the stored input and output numerical samples
importance factors
[1]:
from __future__ import print_function
import openturns as ot
Create the joint distribution of the parameters.
[2]:
distribution_R = ot.LogNormalMuSigma(300.0, 30.0, 0.0).getDistribution()
distribution_F = ot.Normal(75e3, 5e3)
marginals = [distribution_R, distribution_F]
distribution = ot.ComposedDistribution(marginals)
Create the model.
[3]:
model = ot.SymbolicFunction(['R', 'F'], ['R-F/(pi_*100.0)'])
[4]:
modelCallNumberBefore = model.getEvaluationCallsNumber()
modelGradientCallNumberBefore = model.getGradientCallsNumber()
modelHessianCallNumberBefore = model.getHessianCallsNumber()
To have access to the input and output samples after the simulation, activate the History mechanism.
[5]:
model = ot.MemoizeFunction(model)
Remove all the values stored in the history mechanism. Care : it is done regardless the status of the History mechanism.
[6]:
model.clearHistory()
Create the event whose probability we want to estimate.
[7]:
vect = ot.RandomVector(distribution)
G = ot.CompositeRandomVector(model, vect)
event = ot.ThresholdEvent(G, ot.Less(), 0.0)
Create a Monte Carlo algorithm.
[8]:
experiment = ot.MonteCarloExperiment()
algo = ot.ProbabilitySimulationAlgorithm(event, experiment)
algo.setMaximumCoefficientOfVariation(0.1)
algo.setMaximumStandardDeviation(0.001)
algo.setMaximumOuterSampling(int(1e4))
Define the HistoryStrategy to store the values of and
used ot draw the convergence graph. Compact strategy : N points
[9]:
N = 1000
algo.setConvergenceStrategy(ot.Compact(N))
algo.run()
Retrieve result structure.
[10]:
result = algo.getResult()
Display the simulation event probability.
[11]:
result.getProbabilityEstimate()
[11]:
0.030580075662042867
[12]:
# Criteria 3 : Display the Standard Deviation of the estimator
result.getStandardDeviation()
[12]:
0.003057093060511293
Display the variance of the simulation probability estimator.
[13]:
result.getVarianceEstimate()
[13]:
9.345817980626304e-06
[14]:
# Criteria 2 : Display the number of iterations of the simulation
result.getOuterSampling()
[14]:
3172
[15]:
# Display the total number of evaluations of the model
result.getOuterSampling() * result.getBlockSize()
[15]:
3172
Save the number of calls to the model, its gradient and hessian done so far.
[16]:
modelCallNumberAfter = model.getEvaluationCallsNumber()
modelGradientCallNumberAfter = model.getGradientCallsNumber()
modelHessianCallNumberAfter = model.getHessianCallsNumber()
Display the number of iterations executed and the number of evaluations of the model.
[17]:
modelCallNumberAfter - modelCallNumberBefore
[17]:
3172
Get the mean point in event domain care : only for Monte Carlo and LHS sampling methods.
[18]:
result.getMeanPointInEventDomain()
[18]:
[245.843,80222]
Get the associated importance factors care : only for Monte Carlo and LHS sampling methods.
[19]:
result.getImportanceFactors()
[19]:
[X0 : 0.776373, X1 : 0.223627]
[20]:
result.drawImportanceFactors()
[20]:
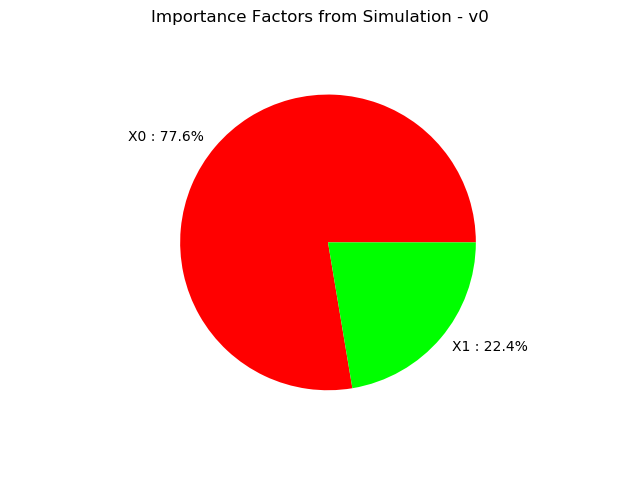
Display the confidence interval length centered around the MonteCarlo probability. The confidence interval is
with level 0.95, where is the estimated probability and
is the confidence interval length.
[21]:
probability = result.getProbabilityEstimate()
length95 = result.getConfidenceLength(0.95)
print("0.95 Confidence Interval length = ", length95)
print("IC at 0.95 = [", probability - 0.5*length95, "; ", probability + 0.5*length95, "]")
0.95 Confidence Interval length = 0.011983584591978923
IC at 0.95 = [ 0.024588283366053405 ; 0.03657186795803233 ]
Draw the convergence graph and the confidence interval of level alpha. By default, alpha = 0.95.
[22]:
alpha = 0.90
algo.drawProbabilityConvergence(alpha)
[22]:
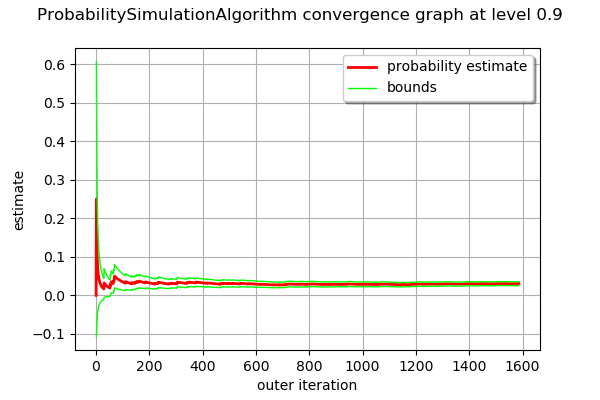
Get the numerical samples of the input and output random vectors stored according to the History Strategy specified and used to evaluate the probability estimator and its variance.
[23]:
inputSampleStored = model.getInputHistory()
outputSampleStored = model.getOutputHistory()
inputSampleStored
[23]:
v0 | v1 | |
---|---|---|
0 | 317.18215872560563 | 68669.13448891672 |
1 | 285.74211953628173 | 81027.39100414288 |
2 | 240.13738518981802 | 76750.21043265145 |
... | ||
3169 | 266.95634238937606 | 74830.12233857176 |
3170 | 282.28504401568824 | 78480.19105287212 |
3171 | 247.17242375744675 | 81708.23104995265 |
Get the values of the estimator and its variance stored according to the History Strategy specified and used to draw the convergence graph.
[24]:
estimator_probability_sample = algo.getConvergenceStrategy().getSample()[0]
estimator_variance_sample = algo.getConvergenceStrategy().getSample()[1]
print(estimator_probability_sample, estimator_variance_sample)
[0,-1] [0.25,0.046875]