FunctionalChaosRandomVector¶
- class FunctionalChaosRandomVector(*args)¶
Functional chaos random vector.
Allows one to simulate a variable through a chaos decomposition, and retrieve its mean and covariance analytically from the chaos coefficients.
- Parameters:
- functionalChaosResult
FunctionalChaosResult
A result from a functional chaos decomposition.
- functionalChaosResult
Methods
Accessor to the antecedent RandomVector in case of a composite RandomVector.
Accessor to the object's name.
Accessor to the covariance of the functional chaos expansion.
Accessor to the description of the RandomVector.
Accessor to the dimension of the RandomVector.
Accessor to the distribution of the RandomVector.
Accessor to the domain of the Event.
Accessor to the Function in case of a composite RandomVector.
Accessor to the functional chaos result.
getId
()Accessor to the object's id.
getMarginal
(*args)Get the random vector corresponding to the
marginal component(s).
getMean
()Accessor to the mean of the functional chaos expansion.
getName
()Accessor to the object's name.
Accessor to the comparaison operator of the Event.
Accessor to the parameter of the distribution.
Accessor to the parameter description of the distribution.
Get the stochastic process.
Compute one realization of the RandomVector.
getSample
(size)Compute realizations of the RandomVector.
Accessor to the object's shadowed id.
Accessor to the threshold of the Event.
Accessor to the object's visibility state.
hasName
()Test if the object is named.
Test if the object has a distinguishable name.
Accessor to know if the RandomVector is a composite one.
isEvent
()Whether the random vector is an event.
setDescription
(description)Accessor to the description of the RandomVector.
setName
(name)Accessor to the object's name.
setParameter
(parameters)Accessor to the parameter of the distribution.
setShadowedId
(id)Accessor to the object's shadowed id.
setVisibility
(visible)Accessor to the object's visibility state.
- __init__(*args)¶
- getAntecedent()¶
Accessor to the antecedent RandomVector in case of a composite RandomVector.
- Returns:
- antecedent
RandomVector
Antecedent RandomVector
in case of a
CompositeRandomVector
such as:.
- antecedent
- getClassName()¶
Accessor to the object’s name.
- Returns:
- class_namestr
The object class name (object.__class__.__name__).
- getCovariance()¶
Accessor to the covariance of the functional chaos expansion.
Let
be the dimension of the input random vector, let
be the dimension of the output random vector. and let
be the number of coefficients in the basis. We consider the following functional chaos expansion:
where
is the approximation of the output random variable
by the expansion,
are the coefficients,
are the orthonormal functions in the basis, and
is the standardized random input vector. The previous equation can be equivalently written as follows:
for
where
is the
-th component of the
-th coefficient in the expansion:
The covariance matrix of the functional chaos expansion is the matrix
, where each component is:
for
. The covariance can be computed using the coefficients of the expansion:
for
. This covariance involves all the coefficients, except the first one. The diagonal of the covariance matrix is the marginal variance:
for
.
- Returns:
- covariance
CovarianceMatrix
, dimension The covariance of the functional chaos expansion.
- covariance
Examples
>>> from openturns.usecases import ishigami_function >>> import openturns as ot >>> import math >>> im = ishigami_function.IshigamiModel() >>> sampleSize = 1000 >>> inputTrain = im.distributionX.getSample(sampleSize) >>> outputTrain = im.model(inputTrain) >>> multivariateBasis = ot.OrthogonalProductPolynomialFactory([im.X1, im.X2, im.X3]) >>> selectionAlgorithm = ot.LeastSquaresMetaModelSelectionFactory() >>> projectionStrategy = ot.LeastSquaresStrategy(selectionAlgorithm) >>> totalDegree = 10 >>> enumerateFunction = multivariateBasis.getEnumerateFunction() >>> basisSize = enumerateFunction.getBasisSizeFromTotalDegree(totalDegree) >>> adaptiveStrategy = ot.FixedStrategy(multivariateBasis, basisSize) >>> chaosAlgo = ot.FunctionalChaosAlgorithm( ... inputTrain, outputTrain, im.distributionX, adaptiveStrategy, projectionStrategy ... ) >>> chaosAlgo.run() >>> chaosResult = chaosAlgo.getResult() >>> randomVector = ot.FunctionalChaosRandomVector(chaosResult) >>> covarianceMatrix = randomVector.getCovariance() >>> covarianceMatrix = randomVector.getCovariance() >>> print('covarianceMatrix=', covarianceMatrix[0, 0]) covarianceMatrix= 13.8... >>> outputDimension = outputTrain.getDimension() >>> stdDev = ot.Point([math.sqrt(covarianceMatrix[i, i]) for i in range(outputDimension)]) >>> print('stdDev=', stdDev[0]) stdDev= 3.72...
- getDescription()¶
Accessor to the description of the RandomVector.
- Returns:
- description
Description
Describes the components of the RandomVector.
- description
- getDimension()¶
Accessor to the dimension of the RandomVector.
- Returns:
- dimensionpositive int
Dimension of the RandomVector.
- getDistribution()¶
Accessor to the distribution of the RandomVector.
- Returns:
- distribution
Distribution
Distribution of the considered
UsualRandomVector
.
- distribution
Examples
>>> import openturns as ot >>> distribution = ot.Normal([0.0, 0.0], [1.0, 1.0], ot.CorrelationMatrix(2)) >>> randomVector = ot.RandomVector(distribution) >>> ot.RandomGenerator.SetSeed(0) >>> print(randomVector.getDistribution()) Normal(mu = [0,0], sigma = [1,1], R = [[ 1 0 ] [ 0 1 ]])
- getDomain()¶
Accessor to the domain of the Event.
- Returns:
- domain
Domain
Describes the domain of an event.
- domain
- getFunction()¶
Accessor to the Function in case of a composite RandomVector.
- Returns:
- function
Function
Function used to define a
CompositeRandomVector
as the image through this function of the antecedent:
.
- function
- getFunctionalChaosResult()¶
Accessor to the functional chaos result.
- Returns:
- functionalChaosResult
FunctionalChaosResult
The result from a functional chaos decomposition.
- functionalChaosResult
- getId()¶
Accessor to the object’s id.
- Returns:
- idint
Internal unique identifier.
- getMarginal(*args)¶
Get the random vector corresponding to the
marginal component(s).
- Parameters:
- iint or list of ints,
Indicates the component(s) concerned.
is the dimension of the RandomVector.
- iint or list of ints,
- Returns:
- vector
RandomVector
RandomVector restricted to the concerned components.
- vector
Notes
Let’s note
a random vector and
a set of indices. If
is a
UsualRandomVector
, the subvector is defined by. If
is a
CompositeRandomVector
, defined bywith
,
some scalar functions, the subvector is
.
Examples
>>> import openturns as ot >>> distribution = ot.Normal([0.0, 0.0], [1.0, 1.0], ot.CorrelationMatrix(2)) >>> randomVector = ot.RandomVector(distribution) >>> ot.RandomGenerator.SetSeed(0) >>> print(randomVector.getMarginal(1).getRealization()) [0.608202] >>> print(randomVector.getMarginal(1).getDistribution()) Normal(mu = 0, sigma = 1)
- getMean()¶
Accessor to the mean of the functional chaos expansion.
Let
be the dimension of the input random vector, let
be the dimension of the output random vector. and let
be the number of coefficients in the basis. We consider the following functional chaos expansion:
where
is the approximation of the output random variable
by the expansion,
are the coefficients,
are the orthonormal functions in the basis, and
is the standardized random input vector. The previous equation can be equivalently written as follows:
for
where
is the
-th component of the
-th coefficient in the expansion:
The mean of the functional chaos expansion is the first coefficient in the expansion:
for
.
- Returns:
- mean
Point
, dimension The mean of the functional chaos expansion.
- mean
Examples
>>> from openturns.usecases import ishigami_function >>> import openturns as ot >>> im = ishigami_function.IshigamiModel() >>> sampleSize = 1000 >>> inputTrain = im.distributionX.getSample(sampleSize) >>> outputTrain = im.model(inputTrain) >>> multivariateBasis = ot.OrthogonalProductPolynomialFactory([im.X1, im.X2, im.X3]) >>> selectionAlgorithm = ot.LeastSquaresMetaModelSelectionFactory() >>> projectionStrategy = ot.LeastSquaresStrategy(selectionAlgorithm) >>> totalDegree = 10 >>> enumerateFunction = multivariateBasis.getEnumerateFunction() >>> basisSize = enumerateFunction.getBasisSizeFromTotalDegree(totalDegree) >>> adaptiveStrategy = ot.FixedStrategy(multivariateBasis, basisSize) >>> chaosAlgo = ot.FunctionalChaosAlgorithm( ... inputTrain, outputTrain, im.distributionX, adaptiveStrategy, projectionStrategy ... ) >>> chaosAlgo.run() >>> chaosResult = chaosAlgo.getResult() >>> randomVector = ot.FunctionalChaosRandomVector(chaosResult) >>> mean = randomVector.getMean() >>> print('mean=', mean[0]) mean= 3.50...
- getName()¶
Accessor to the object’s name.
- Returns:
- namestr
The name of the object.
- getOperator()¶
Accessor to the comparaison operator of the Event.
- Returns:
- operator
ComparisonOperator
Comparaison operator used to define the
RandomVector
.
- operator
- getParameter()¶
Accessor to the parameter of the distribution.
- Returns:
- parameter
Point
Parameter values.
- parameter
- getParameterDescription()¶
Accessor to the parameter description of the distribution.
- Returns:
- description
Description
Parameter names.
- description
- getProcess()¶
Get the stochastic process.
- Returns:
- process
Process
Stochastic process used to define the
RandomVector
.
- process
- getRealization()¶
Compute one realization of the RandomVector.
- Returns:
- aRealization
Point
Sequence of values randomly determined from the RandomVector definition. In the case of an event: one realization of the event (considered as a Bernoulli variable) which is a boolean value (1 for the realization of the event and 0 else).
- aRealization
See also
Examples
>>> import openturns as ot >>> distribution = ot.Normal([0.0, 0.0], [1.0, 1.0], ot.CorrelationMatrix(2)) >>> randomVector = ot.RandomVector(distribution) >>> ot.RandomGenerator.SetSeed(0) >>> print(randomVector.getRealization()) [0.608202,-1.26617] >>> print(randomVector.getRealization()) [-0.438266,1.20548]
- getSample(size)¶
Compute realizations of the RandomVector.
- Parameters:
- nint,
Number of realizations needed.
- nint,
- Returns:
- realizations
Sample
n sequences of values randomly determined from the RandomVector definition. In the case of an event: n realizations of the event (considered as a Bernoulli variable) which are boolean values (1 for the realization of the event and 0 else).
- realizations
See also
Examples
>>> import openturns as ot >>> distribution = ot.Normal([0.0, 0.0], [1.0, 1.0], ot.CorrelationMatrix(2)) >>> randomVector = ot.RandomVector(distribution) >>> ot.RandomGenerator.SetSeed(0) >>> print(randomVector.getSample(3)) [ X0 X1 ] 0 : [ 0.608202 -1.26617 ] 1 : [ -0.438266 1.20548 ] 2 : [ -2.18139 0.350042 ]
- getShadowedId()¶
Accessor to the object’s shadowed id.
- Returns:
- idint
Internal unique identifier.
- getThreshold()¶
Accessor to the threshold of the Event.
- Returns:
- thresholdfloat
Threshold of the
RandomVector
.
- getVisibility()¶
Accessor to the object’s visibility state.
- Returns:
- visiblebool
Visibility flag.
- hasName()¶
Test if the object is named.
- Returns:
- hasNamebool
True if the name is not empty.
- hasVisibleName()¶
Test if the object has a distinguishable name.
- Returns:
- hasVisibleNamebool
True if the name is not empty and not the default one.
- isComposite()¶
Accessor to know if the RandomVector is a composite one.
- Returns:
- isCompositebool
Indicates if the RandomVector is of type Composite or not.
- isEvent()¶
Whether the random vector is an event.
- Returns:
- isEventbool
Whether it takes it values in {0, 1}.
- setDescription(description)¶
Accessor to the description of the RandomVector.
- Parameters:
- descriptionstr or sequence of str
Describes the components of the RandomVector.
- setName(name)¶
Accessor to the object’s name.
- Parameters:
- namestr
The name of the object.
- setParameter(parameters)¶
Accessor to the parameter of the distribution.
- Parameters:
- parametersequence of float
Parameter values.
- setShadowedId(id)¶
Accessor to the object’s shadowed id.
- Parameters:
- idint
Internal unique identifier.
- setVisibility(visible)¶
Accessor to the object’s visibility state.
- Parameters:
- visiblebool
Visibility flag.
Examples using the class¶
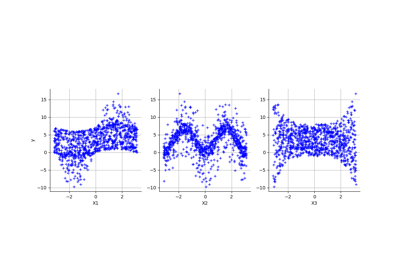
Create a polynomial chaos for the Ishigami function: a quick start guide to polynomial chaos