Note
Go to the end to download the full example code.
Draw multidimensional functions, distributions and events¶
This example shows how to represent multidimensional functions, distributions and events. When 2D plots are to draw, contours are used. We use 2D cross-sections to represent multidimensional objects when required, which leads to cross-cuts representations.
import otbenchmark as otb
import openturns.viewer as otv
import matplotlib.pyplot as plt
problem = otb.ReliabilityProblem33()
event = problem.getEvent()
g = event.getFunction()
Compute the bounds of the domain¶
inputVector = event.getAntecedent()
distribution = inputVector.getDistribution()
inputDimension = distribution.getDimension()
inputDimension
3
alpha = 1 - 1.0e-5
bounds, marginalProb = distribution.computeMinimumVolumeIntervalWithMarginalProbability(
alpha
)
referencePoint = distribution.getMean()
referencePoint
crossCut = otb.CrossCutFunction(g, referencePoint)
fig = crossCut.draw(bounds)
# Remove the legend labels because there
# are too many for such a small figure
for ax in fig.axes:
ax.legend("")
# Increase space between sub-figures so that
# there are no overlap
plt.subplots_adjust(hspace=0.4, wspace=0.4)
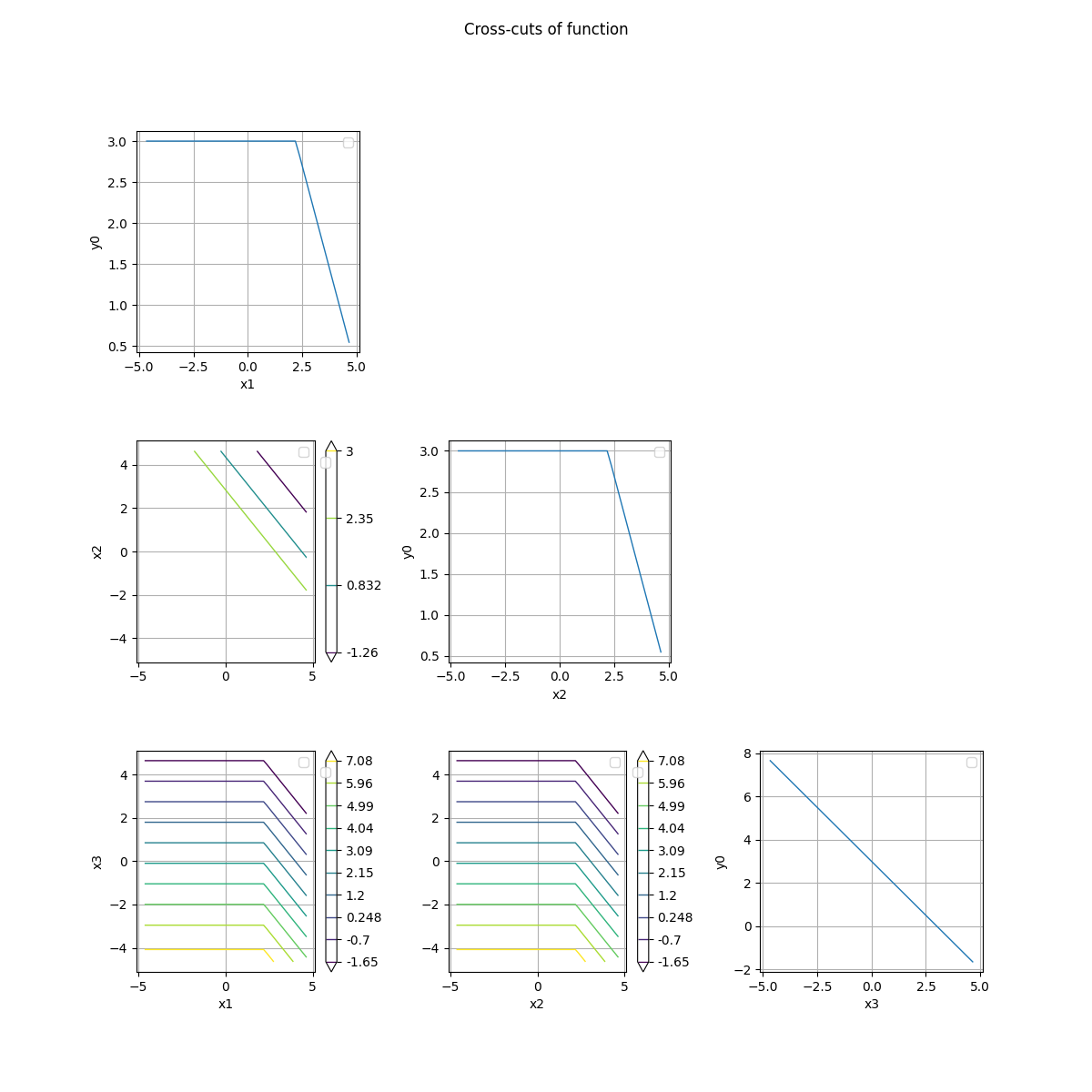
Plot cross-cuts of the distribution
crossCut = otb.CrossCutDistribution(distribution)
fig = crossCut.drawMarginalPDF()
# Remove the legend labels because there
# are too many for such a small figure
for ax in fig.axes:
ax.legend("")
# Increase space between sub-figures so that
# there are no overlap
plt.subplots_adjust(hspace=0.4, wspace=0.4)
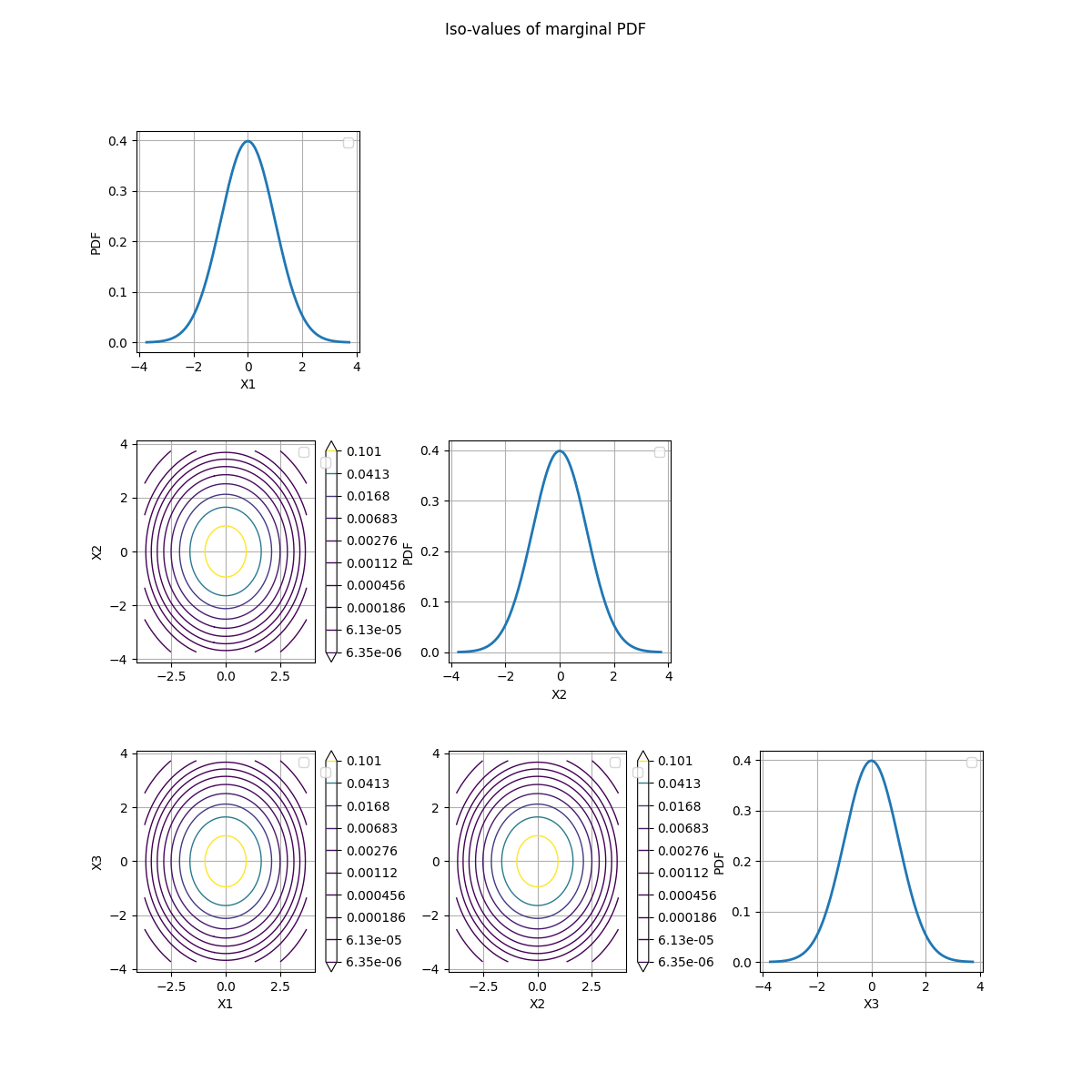
The correct way to represent cross-cuts of a distribution is to draw the contours of the PDF of the conditional distribution.
fig = crossCut.drawConditionalPDF(referencePoint)
# Remove the legend labels because there
# are too many for such a small figure
for ax in fig.axes:
ax.legend("")
# Increase space between sub-figures so that
# there are no overlap
plt.subplots_adjust(hspace=0.4, wspace=0.4)
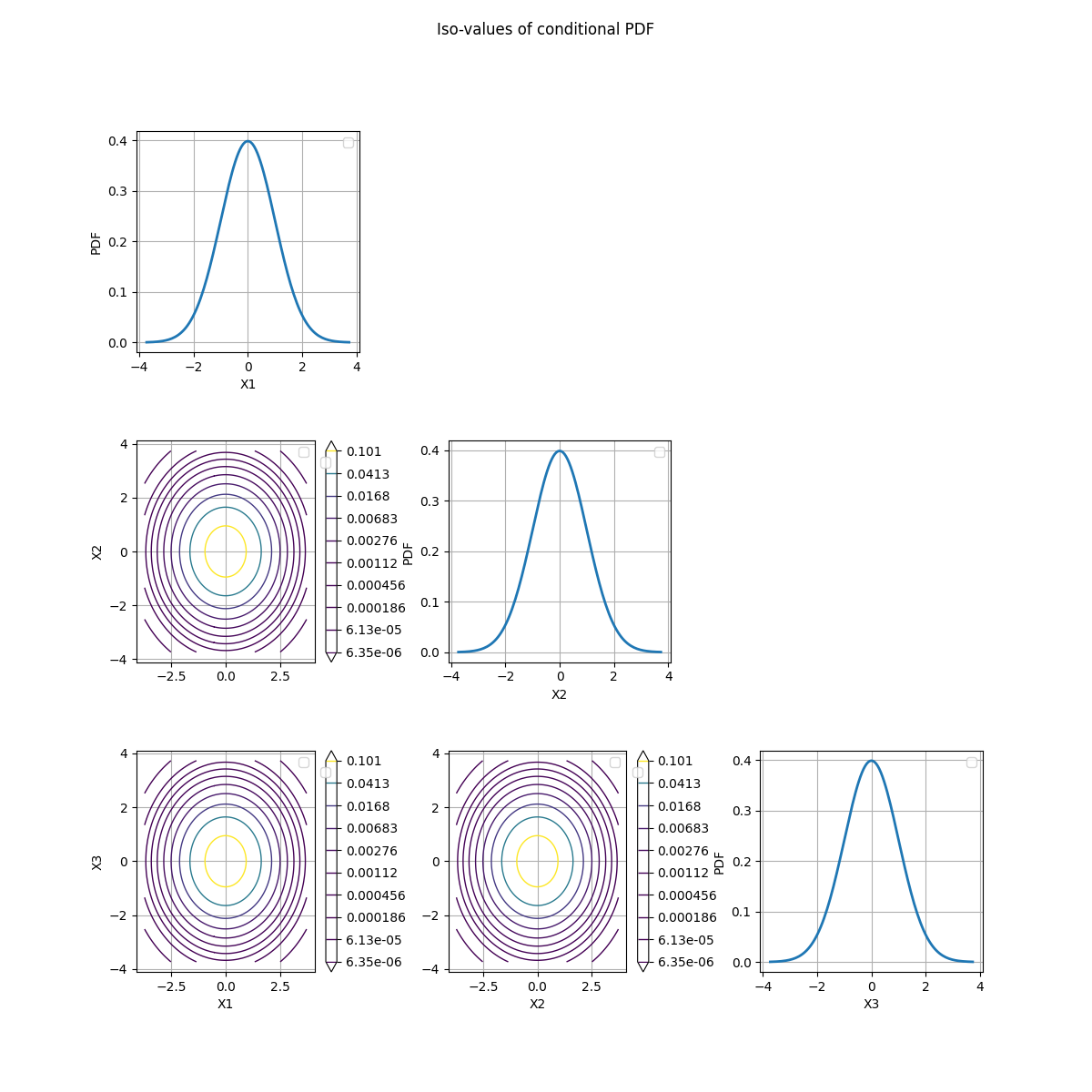
Descr = 1 0
Descr = 2 0
Descr = 2 1
inputVector = event.getAntecedent()
event = problem.getEvent()
g = event.getFunction()
drawEvent = otb.DrawEvent(event)
_ = drawEvent.drawLimitState(bounds)
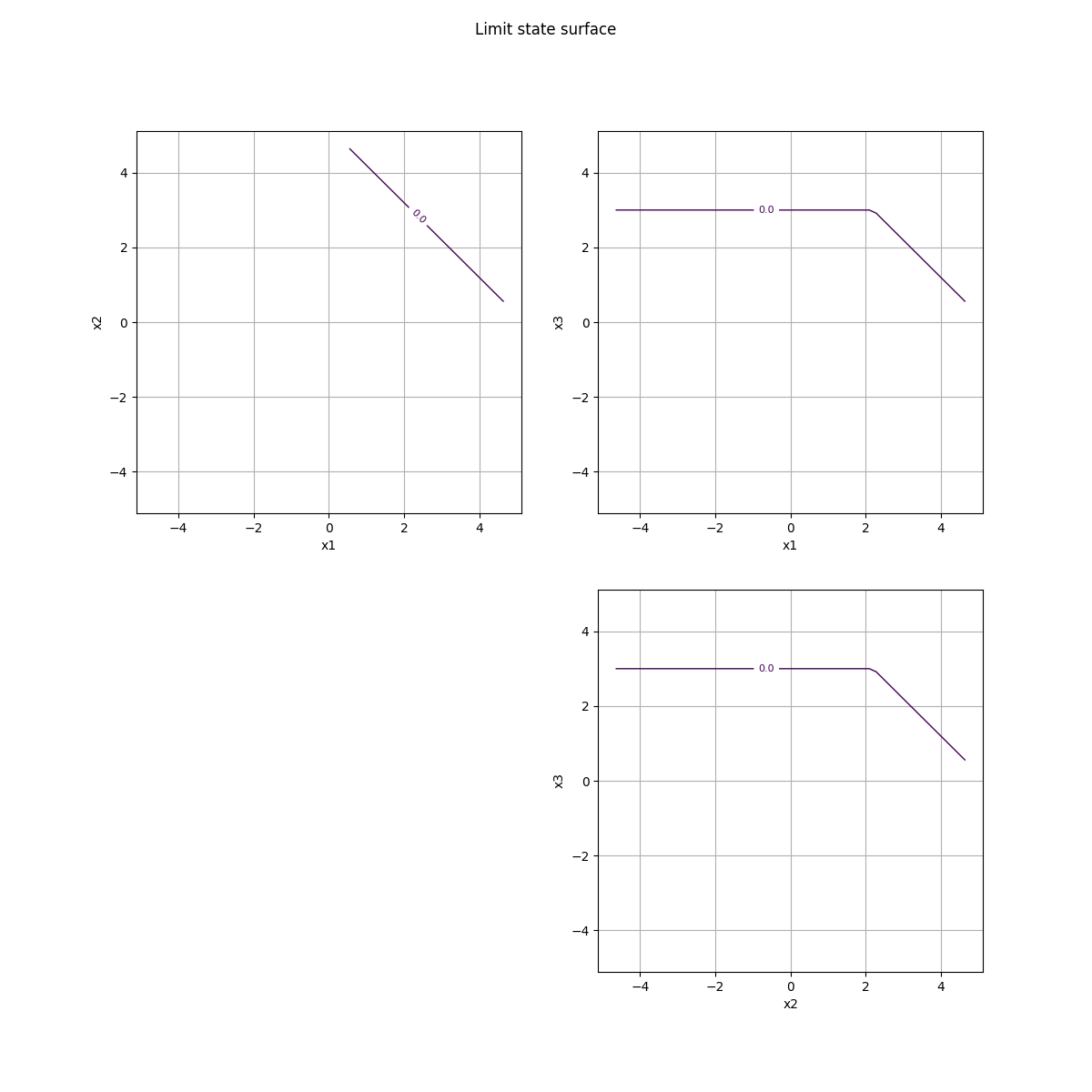
In the following figure, we present the cross-cuts of samples with size equal to 500. These are three different samples, each of which is plotted with the drawSampleCrossCut method. For each cross-cut plot (i,j), the current implementation uses the marginal bivariate distribution, then generates a sample from this distribution. A more rigorous method would draw the conditional distribution, but this might reduce the performance in general. See https://github.com/mbaudin47/otbenchmark/issues/47 for details.
sampleSize = 500
_ = drawEvent.drawSample(sampleSize)
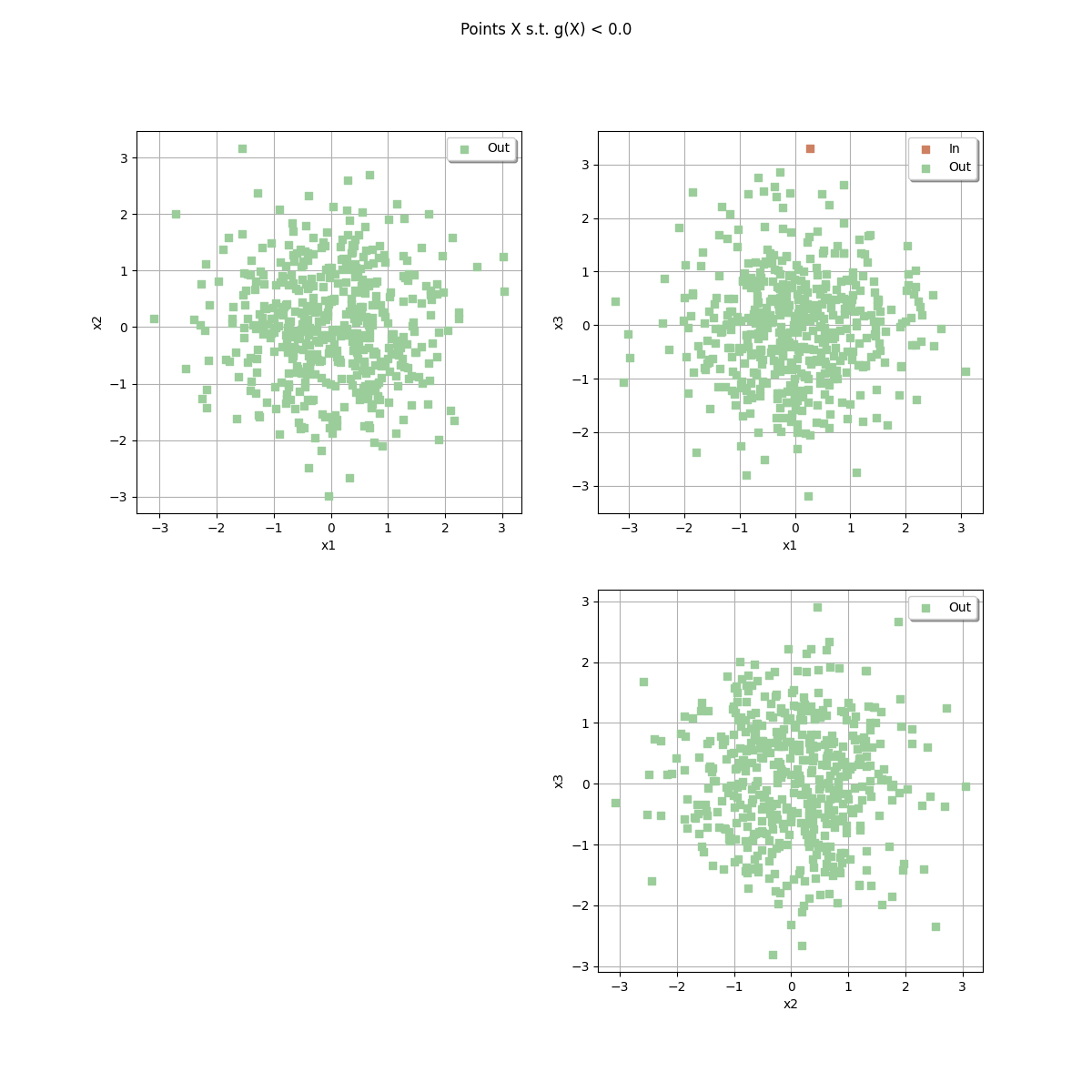
_ = drawEvent.fillEvent(bounds)
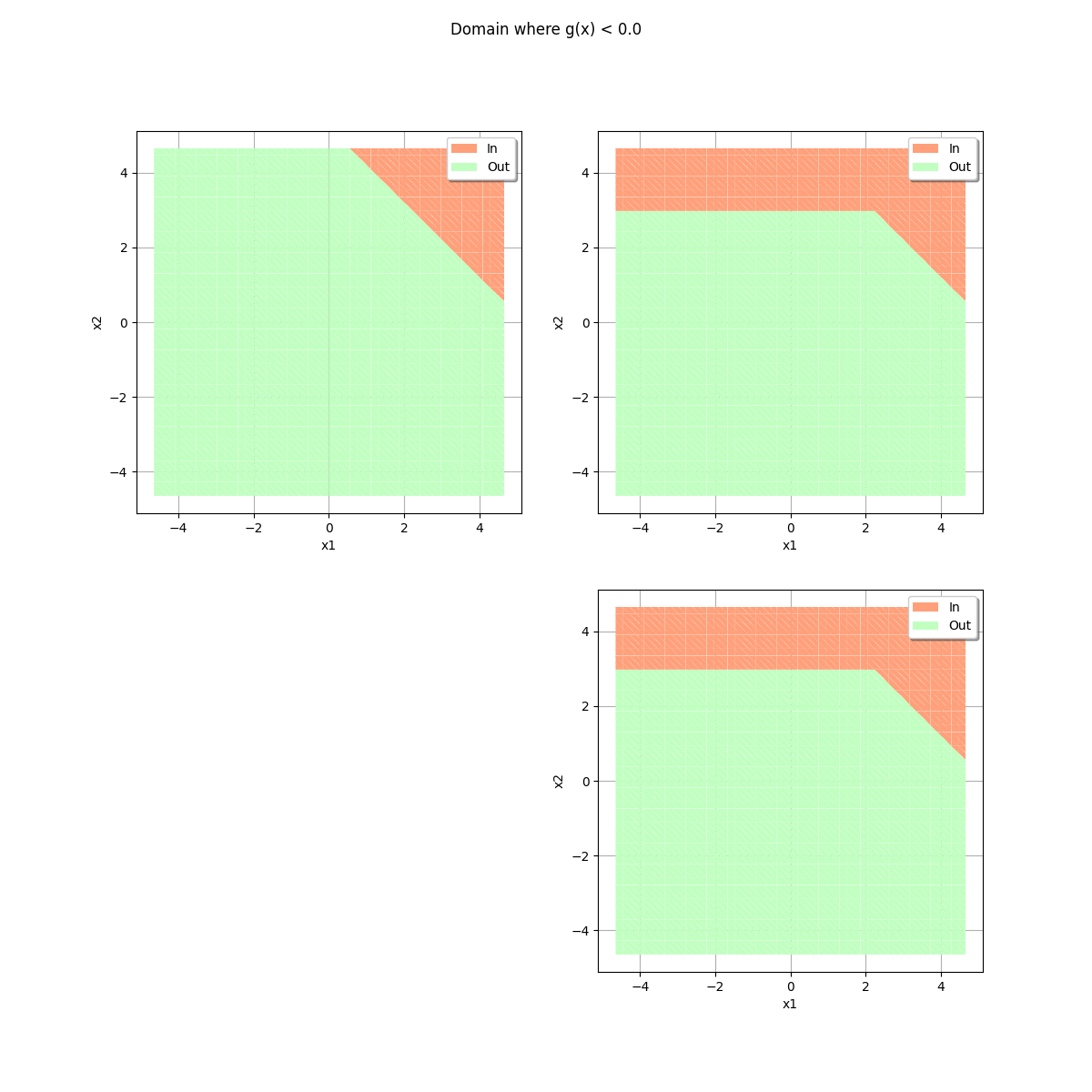
otv.View.ShowAll()
Total running time of the script: (0 minutes 8.697 seconds)