Note
Go to the end to download the full example code.
Initialize FMUFunction
The interest of using FMUs in Python lies in the ease to change its input / parameter values. This notably enables to study the behavior of the FMU with uncertain inputs / parameters.
Initialization scripts can gather a large number of initial values. The use of initialization scripts (.mos files) is common in Dymola :
to save the value of all the variables of a model after simulation,
to restart simulation from a given point.
First, retrieve the path to the FMU deviation.fmu. Recall the deviation model is static, i.e. its output does not evolve over time.
import openturns as ot
from os.path import abspath
import otfmi.example.utility
import otfmi
import openturns.viewer as viewer
path_fmu = otfmi.example.utility.get_path_fmu("deviation")
The initialization script must be provided to FMUFunction constructor. We thus create it now (using Python for clarity).
Note
The initialization script can be automatically created in Dymola.
temporary_file = "initialization.mos"
with open(temporary_file, "w") as f:
f.write("L = 300;\n")
f.write("F = 25000;\n")
If no initial value is provided for an input / parameter, it is set to its default initial value (as set in the FMU).
We can now build the FMUFunction. In the example below, we use the
initialization script to fix the values of L
and F
in the FMU whereas
E
and I
are the function variables.
function = otfmi.FMUFunction(
path_fmu,
inputs_fmu=["E", "I"],
outputs_fmu=["y"],
initialization_script=abspath("initialization.mos"),
)
inputPoint = ot.Point([2e9, 7e7])
outputPoint = function(inputPoint)
print(outputPoint)
[1.60714e-06]
Note
It is possible to set the value of a model input in the initialization script and use it as a function input variable. In this case, the initial value from the initialization script is overriden.
For instance, we consider the 4 model parameters as variables. Note the result is different from above, as the input point overrides the values from the initialization script.
smallExampleFunction = otfmi.FMUFunction(
path_fmu,
inputs_fmu=["E", "F", "L", "I"],
outputs_fmu=["y"],
initialization_script=abspath("initialization.mos"),
)
inputPoint = ot.Point([2e9, 2e4, 800, 7e7])
outputPoint = smallExampleFunction(inputPoint)
print(outputPoint)
[2.4381e-05]
Come back to the function with 2 input variables defined above.
F
and L
initial values are defined in the initialization script, and
remain constant over time. We can now set probability laws on the function
input variables E
and I
to propagate their uncertainty through the
model:
lawE = ot.Uniform(65e9, 75e9)
lawI = ot.Uniform(1.3e7, 1.7e7)
dist = ot.ComposedDistribution([lawE, lawI])
inputSample = dist.getSample(10)
outputSample = function(inputSample)
graph = ot.HistogramFactory().build(outputSample).drawPDF()
view = viewer.View(graph)
view.ShowAll()
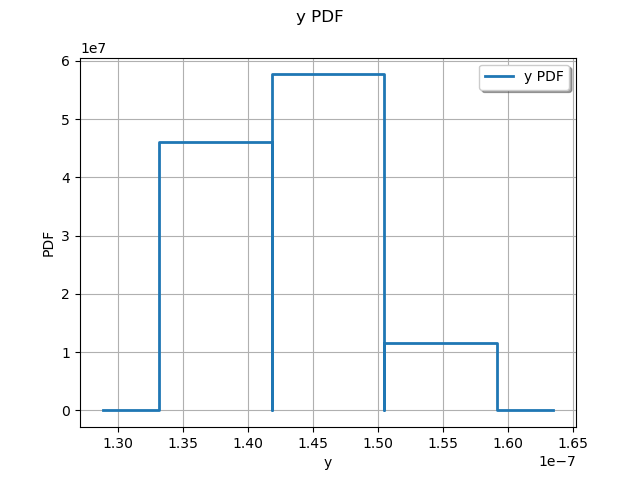
Total running time of the script: (0 minutes 0.307 seconds)