BootstrapExperiment¶
(Source code
, png
)
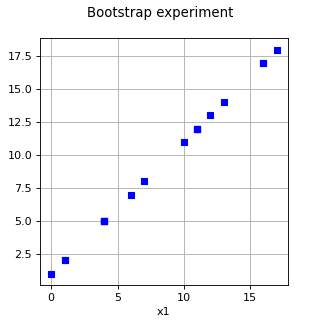
- class BootstrapExperiment(*args)¶
Bootstrap experiment.
- Parameters:
- sample2-d sequence of float
Points to defined a
UserDefined
distribution.
Methods
GenerateSelection
(size, length)Generate a list of indices of points with replacement.
generate
()Generate points according to the type of the experiment.
Generate points and their associated weight according to the type of the experiment.
Accessor to the object's name.
Accessor to the distribution.
getName
()Accessor to the object's name.
getSize
()Accessor to the size of the generated sample.
hasName
()Test if the object is named.
Ask whether the experiment has uniform weights.
isRandom
()Accessor to the randomness of quadrature.
setDistribution
(distribution)Accessor to the distribution.
setName
(name)Accessor to the object's name.
setSize
(size)Accessor to the size of the generated sample.
See also
Notes
BootstrapExperiment is a random weighted design of experiments. Calling the
BootstrapExperiment
constructor is equivalent to calling theWeightedExperiment
constructor as follows:WeightedExperiment(UserDefined(sample), sample.getSize())
Examples
>>> import openturns as ot >>> ot.RandomGenerator.SetSeed(0) >>> sample = [[i,i+1] for i in range(5)] >>> experiment = ot.BootstrapExperiment(sample) >>> print(experiment.generate()) [ v0 v1 ] 0 : [ 4 5 ] 1 : [ 1 2 ] 2 : [ 1 2 ] 3 : [ 1 2 ] 4 : [ 2 3 ] >>> print(experiment.getDistribution()) UserDefined({x = [0,1], p = 0.2}, {x = [1,2], p = 0.2}, {x = [2,3], p = 0.2}, {x = [3,4], p = 0.2}, {x = [4,5], p = 0.2})
- __init__(*args)¶
- static GenerateSelection(size, length)¶
Generate a list of indices of points with replacement.
- Parameters:
- sizepositive int
Number of indices to choose.
- npositive int
Upper bound of the interval in which the indices are chosen.
- Returns:
- selection
Indices
Sequence of size size of indices
such that
.
- selection
- generate()¶
Generate points according to the type of the experiment.
- Returns:
- sample
Sample
Points
of the design of experiments. The sampling method is defined by the type of the weighted experiment.
- sample
Examples
>>> import openturns as ot >>> ot.RandomGenerator.SetSeed(0) >>> myExperiment = ot.MonteCarloExperiment(ot.Normal(2), 5) >>> sample = myExperiment.generate() >>> print(sample) [ X0 X1 ] 0 : [ 0.608202 -1.26617 ] 1 : [ -0.438266 1.20548 ] 2 : [ -2.18139 0.350042 ] 3 : [ -0.355007 1.43725 ] 4 : [ 0.810668 0.793156 ]
- generateWithWeights()¶
Generate points and their associated weight according to the type of the experiment.
- Returns:
Examples
>>> import openturns as ot >>> ot.RandomGenerator.SetSeed(0) >>> myExperiment = ot.MonteCarloExperiment(ot.Normal(2), 5) >>> sample, weights = myExperiment.generateWithWeights() >>> print(sample) [ X0 X1 ] 0 : [ 0.608202 -1.26617 ] 1 : [ -0.438266 1.20548 ] 2 : [ -2.18139 0.350042 ] 3 : [ -0.355007 1.43725 ] 4 : [ 0.810668 0.793156 ] >>> print(weights) [0.2,0.2,0.2,0.2,0.2]
- getClassName()¶
Accessor to the object’s name.
- Returns:
- class_namestr
The object class name (object.__class__.__name__).
- getDistribution()¶
Accessor to the distribution.
- Returns:
- distribution
Distribution
Distribution of the input random vector.
- distribution
- getName()¶
Accessor to the object’s name.
- Returns:
- namestr
The name of the object.
- getSize()¶
Accessor to the size of the generated sample.
- Returns:
- sizepositive int
Number
of points constituting the design of experiments.
- hasName()¶
Test if the object is named.
- Returns:
- hasNamebool
True if the name is not empty.
- hasUniformWeights()¶
Ask whether the experiment has uniform weights.
- Returns:
- hasUniformWeightsbool
Whether the experiment has uniform weights.
- isRandom()¶
Accessor to the randomness of quadrature.
- Parameters:
- isRandombool
Is true if the design of experiments is random. Otherwise, the design of experiment is assumed to be deterministic.
- setDistribution(distribution)¶
Accessor to the distribution.
- Parameters:
- distribution
Distribution
Distribution of the input random vector.
- distribution
- setName(name)¶
Accessor to the object’s name.
- Parameters:
- namestr
The name of the object.
- setSize(size)¶
Accessor to the size of the generated sample.
- Parameters:
- sizepositive int
Number
of points constituting the design of experiments.