CompositeDistribution¶
(Source code
, png
)
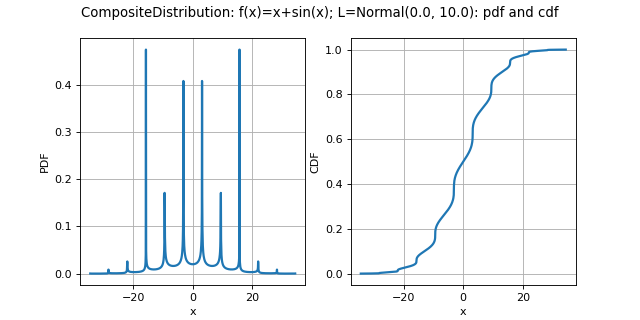
- class CompositeDistribution(*args)¶
Composite distribution.
Helper class for defining the push-forward distribution of a given univariate distribution by a given scalar function.
- Available constructors:
CompositeDistribution(g, distX)
CompositeDistribution(g, distX, a, v)
- Parameters:
- g
Function
, - distX
Distribution
, univariate - asequence of float of dimension
,
,
The bounds of the intervals on which
is monotone, sorted in ascending order.
- vsequence of float of dimension
,
The values taken by
on each bound:
.
- g
Methods
abs
()Transform distribution by absolute value function.
acos
()Transform distribution by arccosine function.
acosh
()Transform distribution by acosh function.
asin
()Transform distribution by arcsine function.
asinh
()Transform distribution by asinh function.
atan
()Transform distribution by arctangent function.
atanh
()Transform distribution by atanh function.
cbrt
()Transform distribution by cubic root function.
Compute a bilateral confidence interval.
computeBilateralConfidenceIntervalWithMarginalProbability
(prob)Compute a bilateral confidence interval.
computeCDF
(*args)Compute the cumulative distribution function.
computeCDFGradient
(*args)Compute the gradient of the cumulative distribution function.
Compute the characteristic function.
computeComplementaryCDF
(*args)Compute the complementary cumulative distribution function.
computeConditionalCDF
(*args)Compute the conditional cumulative distribution function.
computeConditionalDDF
(x, y)Compute the conditional derivative density function of the last component.
computeConditionalPDF
(*args)Compute the conditional probability density function.
computeConditionalQuantile
(*args)Compute the conditional quantile function of the last component.
computeDDF
(*args)Compute the derivative density function.
Compute the entropy of the distribution.
computeGeneratingFunction
(*args)Compute the probability-generating function.
Compute the inverse survival function.
Compute the logarithm of the characteristic function.
computeLogGeneratingFunction
(*args)Compute the logarithm of the probability-generating function.
computeLogPDF
(*args)Compute the logarithm of the probability density function.
computeLogPDFGradient
(*args)Compute the gradient of the log probability density function.
Compute the lower extremal dependence coefficients.
Compute the lower tail dependence coefficients.
Compute the confidence interval with minimum volume.
Compute the confidence interval with minimum volume.
Compute the confidence domain with minimum volume.
Compute the confidence domain with minimum volume.
computePDF
(*args)Compute the probability density function.
computePDFGradient
(*args)Compute the gradient of the probability density function.
computeProbability
(interval)Compute the interval probability.
computeQuantile
(*args)Compute the quantile function.
computeRadialDistributionCDF
(radius[, tail])Compute the cumulative distribution function of the squared radius.
computeScalarQuantile
(prob[, tail])Compute the quantile function for univariate distributions.
Compute the sequential conditional cumulative distribution functions.
Compute the sequential conditional derivative density function.
Compute the sequential conditional probability density function.
Compute the conditional quantile function of the last component.
computeSurvivalFunction
(*args)Compute the survival function.
computeUnilateralConfidenceInterval
(prob[, tail])Compute a unilateral confidence interval.
computeUnilateralConfidenceIntervalWithMarginalProbability
(...)Compute a unilateral confidence interval.
Compute the upper extremal dependence coefficients.
Compute the upper tail dependence coefficients.
cos
()Transform distribution by cosine function.
cosh
()Transform distribution by cosh function.
drawCDF
(*args)Draw the cumulative distribution function.
drawLogPDF
(*args)Draw the graph or of iso-lines of log-probability density function.
Draw the lower extremal dependence function.
Draw the lower tail dependence function.
drawMarginal1DCDF
(marginalIndex, xMin, xMax, ...)Draw the cumulative distribution function of a margin.
drawMarginal1DLogPDF
(marginalIndex, xMin, ...)Draw the log-probability density function of a margin.
drawMarginal1DPDF
(marginalIndex, xMin, xMax, ...)Draw the probability density function of a margin.
drawMarginal1DSurvivalFunction
(...[, logScale])Draw the cumulative distribution function of a margin.
drawMarginal2DCDF
(firstMarginal, ...[, ...])Draw the cumulative distribution function of a couple of margins.
drawMarginal2DLogPDF
(firstMarginal, ...[, ...])Draw the log-probability density function of a couple of margins.
drawMarginal2DPDF
(firstMarginal, ...[, ...])Draw the probability density function of a couple of margins.
drawMarginal2DSurvivalFunction
(...[, ...])Draw the cumulative distribution function of a couple of margins.
drawPDF
(*args)Draw the graph or of iso-lines of probability density function.
drawQuantile
(*args)Draw the quantile function.
drawSurvivalFunction
(*args)Draw the cumulative distribution function.
Draw the upper extremal dependence function.
Draw the upper tail dependence function.
exp
()Transform distribution by exponential function.
Accessor to the antecedent distribution.
getCDF
()Return the CDF as a
Function
.Accessor to the CDF computation precision.
Accessor to the componentwise central moments.
Accessor to the Cholesky factor of the covariance matrix.
Accessor to the object's name.
Accessor to the copula of the distribution.
Accessor to the correlation matrix.
Accessor to the covariance matrix.
Accessor to the componentwise description.
Accessor to the dimension of the distribution.
Dispersion indicator accessor.
Accessor to the function.
Accessor to the number of Gauss integration points.
Accessor to the inverse Cholesky factor of the covariance matrix.
Accessor to the inverse iso-probabilistic transformation.
Accessor to the iso-probabilistic transformation.
Accessor to the Kendall coefficients matrix.
Accessor to the componentwise kurtosis.
Return the logarithm of the PDF as a
Function
.getMarginal
(*args)Accessor to marginal distributions.
getMean
()Accessor to the mean.
getMoment
(n)Accessor to the componentwise moments.
getName
()Accessor to the object's name.
getPDF
()Return the PDF as a
Function
.Accessor to the PDF computation precision.
Accessor to the parameter of the distribution.
Accessor to the parameter description of the distribution.
Accessor to the number of parameters in the distribution.
Accessor to the parameter of the distribution.
Accessor to the Pearson correlation matrix.
Position indicator accessor.
Accessor to the discrete probability levels.
getRange
()Accessor to the range of the distribution.
Accessor to a pseudo-random realization from the distribution.
Accessor to roughness of the distribution.
getSample
(size)Accessor to a pseudo-random sample from the distribution.
getSampleByInversion
(size)Accessor to a pseudo-random sample from the distribution.
getSampleByQMC
(size)Accessor to a low discrepancy sample from the distribution.
Accessor to the shape matrix of the underlying copula if it is elliptical.
getShiftedMoment
(n, shift)Accessor to the componentwise shifted moments.
Accessor to the singularities of the PDF function.
Accessor to the componentwise skewness.
Solver accessor.
Accessor to the Spearman correlation matrix.
Accessor to the componentwise standard deviation.
Accessor to the standard distribution.
Accessor to the standard representative distribution in the parametric family.
getSupport
(*args)Accessor to the support of the distribution.
Accessor to the support tolerance for discrete distributions.
Test whether the copula of the distribution is elliptical or not.
Test whether the copula of the distribution is the independent one.
hasName
()Test if the object is named.
inverse
()Transform distribution by inverse function.
Test whether the distribution is continuous or not.
isCopula
()Test whether the distribution is a copula or not.
Test whether the distribution is discrete or not.
Test whether the distribution is elliptical or not.
Test whether the distribution is integer-valued or not.
ln
()Transform distribution by natural logarithm function.
log
()Transform distribution by natural logarithm function.
setAntecedent
(antecedent)Fix the antecedent distribution which is push-forwarded.
setDescription
(description)Accessor to the componentwise description.
setFunction
(function)Fix the function through which the distribution is push-forwarded.
setIntegrationNodesNumber
(integrationNodesNumber)Accessor to the number of Gauss integration points.
setName
(name)Accessor to the object's name.
setParameter
(parameter)Accessor to the parameter of the distribution.
setParametersCollection
(*args)Accessor to the parameter of the distribution.
setSolver
(solver)Solver accessor.
setSupportEpsilon
(epsilon)Accessor to the support tolerance for discrete distributions.
sin
()Transform distribution by sine function.
sinh
()Transform distribution by sinh function.
sqr
()Transform distribution by square function.
sqrt
()Transform distribution by square root function.
tan
()Transform distribution by tangent function.
tanh
()Transform distribution by tanh function.
- Returns:
- distY
Distribution
, univariate is the push-forward distribution of
by
.
- distY
Notes
We note
a scalar random variable which distribution is
, which probability density function is
.
Then
is the distribution of the scalar random variable
, which probability density function
is defined as:
with
,
and
such that
is monotone over
for
.
Its first moments are obtained by numerical integration.
Examples
Create a distribution:
>>> import openturns as ot >>> g = ot.SymbolicFunction(['x'], ['sin(x) + cos(x)']) >>> distY = ot.CompositeDistribution(g, ot.Normal(1.0, 0.5))
>>> g = ot.SymbolicFunction(['x'], ['abs(x)']) >>> a = [-1.0, 0.0, 2.0] >>> v = [1.0, 0.0, 2.0] >>> distZ = ot.CompositeDistribution(g, ot.Uniform(-1.0, 2.0), a, v)
>>> distX = ot.Normal(0.0, 1.0) >>> a0 = distX.getRange().getLowerBound() >>> aN = distX.getRange().getUpperBound() >>> a = [a0[0], 0.0, 0.0, aN[0]] >>> g = ot.SymbolicFunction(['x'], ['1.0/x']) >>> v = [g(a0)[0], -ot.SpecFunc.Infinity, ot.SpecFunc.Infinity, g(aN)[0]] >>> distT = ot.CompositeDistribution(g, distX, a, v)
Draw a sample:
>>> sample = distT.getSample(5)
- __init__(*args)¶
- abs()¶
Transform distribution by absolute value function.
- Returns:
- dist
Distribution
The transformed distribution.
- dist
- acos()¶
Transform distribution by arccosine function.
- Returns:
- dist
Distribution
The transformed distribution.
- dist
- acosh()¶
Transform distribution by acosh function.
- Returns:
- dist
Distribution
The transformed distribution.
- dist
- asin()¶
Transform distribution by arcsine function.
- Returns:
- dist
Distribution
The transformed distribution.
- dist
- asinh()¶
Transform distribution by asinh function.
- Returns:
- dist
Distribution
The transformed distribution.
- dist
- atan()¶
Transform distribution by arctangent function.
- Returns:
- dist
Distribution
The transformed distribution.
- dist
- atanh()¶
Transform distribution by atanh function.
- Returns:
- dist
Distribution
The transformed distribution.
- dist
- cbrt()¶
Transform distribution by cubic root function.
- Returns:
- dist
Distribution
The transformed distribution.
- dist
- computeBilateralConfidenceInterval(prob)¶
Compute a bilateral confidence interval.
- Parameters:
- alphafloat,
The confidence level.
- alphafloat,
- Returns:
- confInterval
Interval
The confidence interval of level alpha.
- confInterval
Notes
We consider an absolutely continuous measure
with density function
.
The bilateral confidence interval
is the Cartesian product
such that there exists
which satisfies the equations
and
for all
and
.
Examples
We consider a Normal(2) distribution with zero mean, unit standard deviation and independent components. We note
its cdf. Due to symetries of the distribution, the bilateral confidence interval is
where
where
is the marginal cdf of each component. Then
is such that
. As
, then,
is equal to
and
.
>>> import openturns as ot >>> dist = ot.Normal(2) >>> confInt = dist.computeBilateralConfidenceInterval(0.9)
- computeBilateralConfidenceIntervalWithMarginalProbability(prob)¶
Compute a bilateral confidence interval.
Refer to
computeBilateralConfidenceInterval()
- Parameters:
- alphafloat,
The confidence level.
- alphafloat,
- Returns:
- confInterval
Interval
The confidence interval of level alpha.
- betafloat
The probability
.
- confInterval
Examples
We consider a Normal(2) distribution with zero mean, unit standard deviation and independent components. We note
its cdf. Due to symetries of the distribution, the bilateral confidence interval is
where
where
is the marginal cdf of each 1D marginal standard Gaussian component. Then
is such that
. As
, then,
is equal to
and
with 4 significant digits.
>>> import openturns as ot >>> dist = ot.Normal(2) >>> confInt, beta = dist.computeBilateralConfidenceIntervalWithMarginalProbability(0.9)
- computeCDF(*args)¶
Compute the cumulative distribution function.
- Parameters:
- xsequence of float, 2-d sequence of float
Point in
.
- Returns:
- Ffloat,
Point
CDF value at x.
- Ffloat,
Notes
The cumulative distribution function is defined as:
- computeCDFGradient(*args)¶
Compute the gradient of the cumulative distribution function.
- Parameters:
- xsequence of float
Point in
.
- Returns:
- dFdtheta
Point
Partial derivatives of the CDF with respect to the distribution parameters at x.
- dFdtheta
- computeCharacteristicFunction(*args)¶
Compute the characteristic function.
- Parameters:
- tfloat
Characteristic function input.
- Returns:
- phicomplex
Characteristic function value at input t.
Notes
The characteristic function is defined as:
OpenTURNS features a generic implementation of the characteristic function for all its univariate distributions (both continuous and discrete). This default implementation might be time consuming, especially as the modulus of
gets high. Only some univariate distributions benefit from dedicated more efficient implementations.
- computeComplementaryCDF(*args)¶
Compute the complementary cumulative distribution function.
- Parameters:
- xsequence of float, 2-d sequence of float
Point in
.
- Returns:
- Cfloat,
Point
Complementary CDF value at x.
- Cfloat,
See also
Notes
The complementary cumulative distribution function.
Warning
The complementary CDF is different from the survival function (except for scalar distributions).
- computeConditionalCDF(*args)¶
Compute the conditional cumulative distribution function.
- Parameters:
- xjfloat, sequence of float
Conditional CDF input.
- xcondsequence of float, 2-d sequence of float with size
,
Conditioning values for the components
.
- Returns:
- pfloat, sequence of float
Conditional CDF value at xj given xcond.
Notes
Let
be a random vector of dimension
. The conditional cumulative distribution function of the component
given that the components of indices
are fixed to
is defined by:
For
, it reduces to
.
- computeConditionalDDF(x, y)¶
Compute the conditional derivative density function of the last component.
With respect to the other fixed components.
- Parameters:
- xjfloat, sequence of float
Conditional CDF input.
- xcondsequence of float, 2-d sequence of float with size
,
Conditioning values for the components
.
- Returns:
- ddffloat,
Conditional DDF value at xj given xcond.
See also
Notes
Let
be a random vector of dimension
. The conditional derivative density function of the component
given that the components of indices
are fixed to
is defined by:
where for
:
For
, it reduces to
, ie the DDF of the first component at
.
- computeConditionalPDF(*args)¶
Compute the conditional probability density function.
Conditional PDF of the last component with respect to the other fixed components.
- Parameters:
- xjfloat, sequence of float,
Conditional CDF input.
- xcondsequence of float, 2-d sequence of float with size
,
Conditioning values for the components
.
- Returns:
- cpdffloat, sequence of float
Conditional PDF at xd, given xcond.
See also
Notes
Let
be a random vector of dimension
. The conditional probability density function of the component
given that the components of indices
are fixed to
is defined by:
where for
:
For
, it reduces to
.
- computeConditionalQuantile(*args)¶
Compute the conditional quantile function of the last component.
Conditional quantile with respect to the other fixed components.
- Parameters:
- pfloat, sequence of float,
Conditional quantile.
- xcondsequence of float, 2-d sequence of float with size
,
Conditioning values for the components
.
- pfloat, sequence of float,
- Returns:
- xjfloat
Conditional quantile of order p of the component
given that the components of indices
are fixed and equal to xcond.
See also
Notes
Let
be a random vector of dimension
. The conditional quantile of order
of the component
given that the components of indices
are fixed to
is defined by:
where
is the quantile function. For
, it reduces to
.
- computeDDF(*args)¶
Compute the derivative density function.
- Parameters:
- xsequence of float, 2-d sequence of float
The input value where the conditional derivative density function must be evaluated.
- Returns:
Notes
The derivative density function is the gradient of the probability density function with respect to
:
- computeEntropy()¶
Compute the entropy of the distribution.
- Returns:
- efloat
Entropy of the distribution.
Notes
The entropy of a distribution is defined by:
Where the random vector
follows the probability distribution of interest, and
is either the probability density function of
if it is continuous or the probability distribution function if it is discrete.
- computeGeneratingFunction(*args)¶
Compute the probability-generating function.
- Parameters:
- zfloat or complex
Probability-generating function input.
- Returns:
- gfloat
Probability-generating function value at input z.
See also
Notes
The probability-generating function is defined as follows:
This function only exists for discrete distributions. OpenTURNS implements this method for univariate distributions only.
- computeInverseSurvivalFunction(point)¶
Compute the inverse survival function.
- Parameters:
- pfloat,
Value of the survival function.
- pfloat,
- Returns:
- x
Point
Point
such that
with iso-quantile components.
- x
See also
Notes
Among the points
that satisfy
, the method returns the one which also satisfies
.
- computeLogCharacteristicFunction(*args)¶
Compute the logarithm of the characteristic function.
- Parameters:
- tfloat
Characteristic function input.
- Returns:
- phicomplex
Logarithm of the characteristic function value at input t.
See also
Notes
OpenTURNS features a generic implementation of the characteristic function for all its univariate distributions (both continuous and discrete). This default implementation might be time consuming, especially as the modulus of
gets high. Only some univariate distributions benefit from dedicated more efficient implementations.
- computeLogGeneratingFunction(*args)¶
Compute the logarithm of the probability-generating function.
- Parameters:
- zfloat or complex
Probability-generating function input.
- Returns:
- lgfloat
Logarithm of the probability-generating function value at input z.
See also
Notes
This function only exists for discrete distributions. OpenTURNS implements this method for univariate distributions only.
- computeLogPDF(*args)¶
Compute the logarithm of the probability density function.
- Parameters:
- xsequence of float, 2-d sequence of float
Point in
such that the PDF at this point is non equal to 0.
- Returns:
- ffloat,
Point
Logarithm of the PDF at x.
- ffloat,
- computeLogPDFGradient(*args)¶
Compute the gradient of the log probability density function.
- Parameters:
- xsequence of float
Point in
.
- Returns:
- dfdtheta
Point
Partial derivatives of the logPDF with respect to the distribution parameters at input x.
- dfdtheta
- computeLowerExtremalDependenceMatrix()¶
Compute the lower extremal dependence coefficients.
We assume that the distribution is
-dimensional, with
and that its copula is denoted by
. The lower extremal dependence matrix is
where
is the lower extremal dependence coefficient of the bivariate distribution
. It is defined by:
where
is the lower extremal dependence function of the bivariate distribution
defined by:
Refer to Tail dependence coefficients to get more details.
- Returns:
- dep
CorrelationMatrix
The dependence matrix.
- dep
Examples
>>> import openturns as ot >>> copula = ot.FrankCopula() >>> chiLb = copula.computeLowerExtremalDependenceMatrix()[1, 0]
- computeLowerTailDependenceMatrix()¶
Compute the lower tail dependence coefficients.
We assume that the distribution is
-dimensional, with
and that its copula is denoted by
. The lower tail dependence matrix is
where
is the lower tail dependence coefficient of the bivariate distribution
. It is defined by:
where
is the lower tail dependence function of the bivariate distribution
defined by:
Refer to Tail dependence coefficients to get more details.
- Returns:
- dep
CorrelationMatrix
The dependence matrix.
- dep
Examples
>>> import openturns as ot >>> copula = ot.FrankCopula() >>> chiL = copula.computeLowerTailDependenceMatrix()[1, 0]
- computeMinimumVolumeInterval(prob)¶
Compute the confidence interval with minimum volume.
- Parameters:
- alphafloat,
The confidence level.
- alphafloat,
- Returns:
- confInterval
Interval
The confidence interval of level alpha.
- confInterval
Notes
We consider an absolutely continuous measure
with density function
.
The minimum volume confidence interval
is the Cartesian product
where
and
with
is the Lebesgue measure on
.
This problem resorts to solving
univariate non linear equations: for a fixed value
, we find each intervals
such that:
which consists of finding the bound
such that:
To find
, we use the Brent algorithm:
with
a non linear function.
Examples
Create a sample from a Normal distribution:
>>> import openturns as ot >>> sample = ot.Normal().getSample(10) >>> ot.ResourceMap.SetAsUnsignedInteger('DistributionFactory-DefaultBootstrapSize', 100)
Fit a Normal distribution and extract the asymptotic parameters distribution:
>>> fittedRes = ot.NormalFactory().buildEstimator(sample) >>> paramDist = fittedRes.getParameterDistribution()
Determine the confidence interval of the native parameters at level 0.9 with minimum volume:
>>> ot.ResourceMap.SetAsUnsignedInteger('Distribution-MinimumVolumeLevelSetSamplingSize', 1000) >>> confInt = paramDist.computeMinimumVolumeInterval(0.9) >>> ot.ResourceMap.Reload()
- computeMinimumVolumeIntervalWithMarginalProbability(prob)¶
Compute the confidence interval with minimum volume.
Refer to
computeMinimumVolumeInterval()
- Parameters:
- alphafloat,
The confidence level.
- alphafloat,
- Returns:
- confInterval
Interval
The confidence interval of level alpha.
- marginalProbfloat
The value
which is the common marginal probability of each marginal interval.
- confInterval
Examples
Create a sample from a Normal distribution:
>>> import openturns as ot >>> sample = ot.Normal().getSample(10) >>> ot.ResourceMap.SetAsUnsignedInteger('DistributionFactory-DefaultBootstrapSize', 100)
Fit a Normal distribution and extract the asymptotic parameters distribution:
>>> fittedRes = ot.NormalFactory().buildEstimator(sample) >>> paramDist = fittedRes.getParameterDistribution()
Determine the confidence interval of the native parameters at level 0.9 with minimum volume:
>>> ot.ResourceMap.SetAsUnsignedInteger('Distribution-MinimumVolumeLevelSetSamplingSize', 1000) >>> confInt, marginalProb = paramDist.computeMinimumVolumeIntervalWithMarginalProbability(0.9) >>> ot.ResourceMap.Reload()
- computeMinimumVolumeLevelSet(prob)¶
Compute the confidence domain with minimum volume.
- Parameters:
- alphafloat,
The confidence level.
- alphafloat,
- Returns:
- levelSet
LevelSet
The minimum volume domain of measure alpha.
- levelSet
Notes
We consider an absolutely continuous measure
with density function
.
The minimum volume confidence domain
is the set of minimum volume and which measure is at least
. It is defined by:
where
is the Lebesgue measure on
. Under some general conditions on
(for example, no flat regions), the set
is unique and realises the minimum:
. We show that
writes:
for a certain
.
If we consider the random variable
, with cumulative distribution function
, then
is defined by:
Thus the minimum volume domain of confidence
is the interior of the domain which frontier is the
quantile of
. It can be determined with simulations of
.
Examples
Create a sample from a Normal distribution:
>>> import openturns as ot >>> sample = ot.Normal().getSample(10) >>> ot.ResourceMap.SetAsUnsignedInteger('DistributionFactory-DefaultBootstrapSize', 100)
Fit a Normal distribution and extract the asymptotic parameters distribution:
>>> fittedRes = ot.NormalFactory().buildEstimator(sample) >>> paramDist = fittedRes.getParameterDistribution()
Determine the confidence region of minimum volume of the native parameters at level 0.9:
>>> levelSet = paramDist.computeMinimumVolumeLevelSet(0.9) >>> ot.ResourceMap.Reload()
- computeMinimumVolumeLevelSetWithThreshold(prob)¶
Compute the confidence domain with minimum volume.
Refer to
computeMinimumVolumeLevelSet()
- Parameters:
- alphafloat,
The confidence level.
- alphafloat,
- Returns:
- levelSet
LevelSet
The minimum volume domain of measure alpha.
- levelfloat
The value
of the density function defining the frontier of the domain.
- levelSet
Examples
Create a sample from a Normal distribution:
>>> import openturns as ot >>> sample = ot.Normal().getSample(10) >>> ot.ResourceMap.SetAsUnsignedInteger('DistributionFactory-DefaultBootstrapSize', 100)
Fit a Normal distribution and extract the asymptotic parameters distribution:
>>> fittedRes = ot.NormalFactory().buildEstimator(sample) >>> paramDist = fittedRes.getParameterDistribution()
Determine the confidence region of minimum volume of the native parameters at level 0.9 with PDF threshold:
>>> levelSet, threshold = paramDist.computeMinimumVolumeLevelSetWithThreshold(0.9)
- computePDF(*args)¶
Compute the probability density function.
- Parameters:
- xsequence of float, 2-d sequence of float
Point in
.
- Returns:
- ffloat,
Point
PDF value at x.
- ffloat,
Notes
The probability density function is defined as follows:
- computePDFGradient(*args)¶
Compute the gradient of the probability density function.
- Parameters:
- xsequence of float
Point in
.
- Returns:
- dfdtheta
Point
Partial derivatives of the PDF with respect to the distribution parameters at input x.
- dfdtheta
Notes
Let
be the vector of parameters of the distribution. Then the gradient of the probability density function
is defined by:
- computeProbability(interval)¶
Compute the interval probability.
- Parameters:
- interval
Interval
An interval in
.
- interval
- Returns:
- pfloat
The probability of interval.
Notes
This computes the probability that the random vector
lies in
.
If the interval is rectangular, i.e. if
, then we have:
where the sum runs over the
vectors such that
with
, and
is the number of components in
such that
.
- computeQuantile(*args)¶
Compute the quantile function.
- Parameters:
- pfloat (or sequence of float),
A probability.
- tailbool, optional
True indicates that the order considered is
. Default value is False.
- pfloat (or sequence of float),
- Returns:
Notes
If the underlying variable
is scalar, then the quantile of order
, denoted by
, is defined as the generalized inverse of its cumulative distribution function:
If the distribution is scalar and discrete, then the quantile of order
is defined by:
If the underlying variable
is of dimension
, then the quantile of order
, denoted by
, is such that:
where
is the
-th marginal cdf. The last condition means that the quantile of order
is such that all the components are associated to the same order of quantile of their margin.
- computeRadialDistributionCDF(radius, tail=False)¶
Compute the cumulative distribution function of the squared radius.
For the underlying standard spherical distribution (for elliptical distributions only).
- Parameters:
- r2float,
Squared radius.
- r2float,
- Returns:
- Ffloat
CDF value at input r2.
Notes
This is the CDF of the sum of the squared independent, standard, identically distributed components:
- computeScalarQuantile(prob, tail=False)¶
Compute the quantile function for univariate distributions.
- Parameters:
- pfloat,
A probability.
- pfloat,
- Returns:
- xpfloat
Quantile of order p.
See also
Notes
The quantile of order
, denoted by
, is defined as the generalized inverse of its cumulative distribution function:
If the distribution is discrete, then the quantile of order
is defined by:
- computeSequentialConditionalCDF(x)¶
Compute the sequential conditional cumulative distribution functions.
- Parameters:
- xsequence of float, with size
Values to be taken sequentially as argument and conditioning part of the CDF.
- xsequence of float, with size
- Returns:
- Fsequence of float
Conditional CDF values at x.
Notes
The sequential conditional cumulative distribution function is defined as follows:
where for
:
The first term, for
, is
.
- computeSequentialConditionalDDF(x)¶
Compute the sequential conditional derivative density function.
- Parameters:
- xsequence of float, with size
Values to be taken sequentially as argument and conditioning part of the DDF.
- xsequence of float, with size
- Returns:
- ddfsequence of float
Conditional DDF values at x.
Notes
The sequential conditional derivative density function is defined by:
where for
:
The first term, for
, is
.
- computeSequentialConditionalPDF(x)¶
Compute the sequential conditional probability density function.
- Parameters:
- xsequence of float, with size
Values to be taken sequentially as argument and conditioning part of the PDF.
- xsequence of float, with size
- Returns:
- pdfsequence of float
Sequence of conditional PDF values at x.
Notes
The sequential conditional density function is defined as follows:
where for
:
The first term, for
, is
.
- computeSequentialConditionalQuantile(q)¶
Compute the conditional quantile function of the last component.
- Parameters:
- psequence of float in
, with size
Values to be taken sequentially as the argument of the conditional quantile.
- psequence of float in
- Returns:
- Qsequence of float
Sequence of conditional quantiles values at p
Notes
The sequential conditional quantile function is defined by:
where
is the quantile function and where
are defined recursively as
and for
,
: the conditioning part is the set of already computed conditional quantiles.
- computeSurvivalFunction(*args)¶
Compute the survival function.
- Parameters:
- xsequence of float, 2-d sequence of float
Point in
.
- Returns:
- sfloat,
Point
Value of the survival function at point x.
- sfloat,
See also
Notes
The survival function of the random vector
of dimension
is defined as follows:
Warning
This is not the complementary cumulative distribution function except for scalar distributions.
- computeUnilateralConfidenceInterval(prob, tail=False)¶
Compute a unilateral confidence interval.
- Parameters:
- alphafloat,
The confidence level.
- tailbool
True indicates the interval is bounded by an lower value. False indicates the interval is bounded by an upper value. Default value is False.
- alphafloat,
- Returns:
- confInterval
Interval
The unilateral confidence interval of level
.
- confInterval
Notes
We consider an absolutely continuous measure
.
The left unilateral confidence interval
is the cartesian product
where
for all
and which verifies
. It means that
is the quantile of level
of the measure
, with iso-quantile components.
The right unilateral confidence interval
is the cartesian product
where
for all
and which verifies
. It means that
with iso-quantile components, where
is the survival function of the measure
.
Examples
Create a sample from a Normal distribution:
>>> import openturns as ot >>> sample = ot.Normal().getSample(10) >>> ot.ResourceMap.SetAsUnsignedInteger('DistributionFactory-DefaultBootstrapSize', 100)
Fit a Normal distribution and extract the asymptotic parameters distribution:
>>> fittedRes = ot.NormalFactory().buildEstimator(sample) >>> paramDist = fittedRes.getParameterDistribution()
Determine the right unilateral confidence interval at level 0.9:
>>> confInt = paramDist.computeUnilateralConfidenceInterval(0.9)
Determine the left unilateral confidence interval at level 0.9:
>>> confInt = paramDist.computeUnilateralConfidenceInterval(0.9, True)
- computeUnilateralConfidenceIntervalWithMarginalProbability(prob, tail)¶
Compute a unilateral confidence interval.
Refer to
computeUnilateralConfidenceInterval()
- Parameters:
- alphafloat,
The confidence level.
- tailbool
True indicates the interval is bounded by an lower value. False indicates the interval is bounded by an upper value. Default value is False.
- alphafloat,
- Returns:
- confInterval
Interval
The unilateral confidence interval of level alpha.
- marginalProbfloat
The value
which is the common marginal probability of each marginal interval.
- confInterval
Examples
Create a sample from a Normal distribution:
>>> import openturns as ot >>> sample = ot.Normal().getSample(10) >>> ot.ResourceMap.SetAsUnsignedInteger('DistributionFactory-DefaultBootstrapSize', 100)
Fit a Normal distribution and extract the asymptotic parameters distribution:
>>> fittedRes = ot.NormalFactory().buildEstimator(sample) >>> paramDist = fittedRes.getParameterDistribution()
Determine the right unilateral confidence interval at level 0.9:
>>> confInt, marginalProb = paramDist.computeUnilateralConfidenceIntervalWithMarginalProbability(0.9, False)
Determine the left unilateral confidence interval at level 0.9:
>>> confInt, marginalProb = paramDist.computeUnilateralConfidenceIntervalWithMarginalProbability(0.9, True) >>> ot.ResourceMap.Reload()
- computeUpperExtremalDependenceMatrix()¶
Compute the upper extremal dependence coefficients.
We assume that the distribution is
-dimensional, with
and that its copula is denoted by
. The upper extremal dependence matrix is
where
is the upper extremal dependence coefficient of the bivariate distribution
. It is defined by:
where
is the upper extremal dependence function of the bivariate distribution
defined by:
Refer to Tail dependence coefficients to get more details.
- Returns:
- dep
CorrelationMatrix
The dependence matrix.
- dep
Examples
>>> import openturns as ot >>> copula = ot.FrankCopula() >>> chib = copula.computeUpperExtremalDependenceMatrix()[1, 0]
- computeUpperTailDependenceMatrix()¶
Compute the upper tail dependence coefficients.
We assume that the distribution is
-dimensional, with
and that its copula is denoted by
. The upper tail dependence matrix is
where
is the upper tail dependence coefficient of the bivariate distribution
. It is defined by:
where
is the upper tail dependence function of the bivariate distribution
defined by:
Refer to Tail dependence coefficients to get more details.
- Returns:
- dep
CorrelationMatrix
The dependence matrix.
- dep
Examples
>>> import openturns as ot >>> copula = ot.FrankCopula() >>> chi = copula.computeUpperTailDependenceMatrix()[1, 0]
- cos()¶
Transform distribution by cosine function.
- Returns:
- dist
Distribution
The transformed distribution.
- dist
- cosh()¶
Transform distribution by cosh function.
- Returns:
- dist
Distribution
The transformed distribution.
- dist
- drawCDF(*args)¶
Draw the cumulative distribution function.
- Available constructors:
drawCDF(x_min, x_max, pointNumber, logScale)
drawCDF(lowerCorner, upperCorner, pointNbrInd, logScaleX, logScaleY)
drawCDF(lowerCorner, upperCorner)
- Parameters:
- xMinfloat, optional
The min-value of the mesh of the x-axis. Defaults uses the quantile associated to the probability level Distribution-QMin from the
ResourceMap
.- xMaxfloat, optional, xMax > xMin
The max-value of the mesh of the y-axis. Defaults uses the quantile associated to the probability level Distribution-QMax from the
ResourceMap
.- pointNumberint
The number of points that is used for meshing each axis. Defaults uses DistributionImplementation-DefaultPointNumber from the
ResourceMap
.- logScalebool
Flag to tell if the plot is done on a logarithmic scale. Default is False.
- lowerCornersequence of float, of dimension 2, optional
The lower corner
.
- upperCornersequence of float, of dimension 2, optional
The upper corner
.
- pointNbrInd
Indices
, of dimension 2 Number of points that is used for meshing each axis.
- logScaleXbool
Flag to tell if the plot is done on a logarithmic scale for X. Default is False.
- logScaleYbool
Flag to tell if the plot is done on a logarithmic scale for Y. Default is False.
- Returns:
- graph
Graph
A graphical representation of the CDF.
- graph
Notes
Only valid for univariate and bivariate distributions.
Examples
View the CDF of a univariate distribution:
>>> import openturns as ot >>> dist = ot.Normal() >>> graph = dist.drawCDF() >>> graph.setLegends(['normal cdf'])
View the iso-lines CDF of a bivariate distribution:
>>> import openturns as ot >>> dist = ot.Normal(2) >>> graph2 = dist.drawCDF() >>> graph2.setLegends(['iso- normal cdf']) >>> graph3 = dist.drawCDF([-10, -5],[5, 10], [511, 511])
- drawLogPDF(*args)¶
Draw the graph or of iso-lines of log-probability density function.
- Available constructors:
drawLogPDF(x_min, x_max, pointNumber, logScale)
drawLogPDF(lowerCorner, upperCorner, pointNbrInd, logScaleX, logScaleY)
drawLogPDF(lowerCorner, upperCorner)
- Parameters:
- xMinfloat, optional
The min-value of the mesh of the x-axis. Defaults uses the quantile associated to the probability level Distribution-QMin from the
ResourceMap
.- xMaxfloat, optional, xMax > xMin
The max-value of the mesh of the y-axis. Defaults uses the quantile associated to the probability level Distribution-QMax from the
ResourceMap
.- pointNumberint
The number of points that is used for meshing each axis. Defaults uses DistributionImplementation-DefaultPointNumber from the
ResourceMap
.- logScalebool
Flag to tell if the plot is done on a logarithmic scale. Default is False.
- lowerCornersequence of float, of dimension 2, optional
The lower corner
.
- upperCornersequence of float, of dimension 2, optional
The upper corner
.
- pointNbrInd
Indices
, of dimension 2 Number of points that is used for meshing each axis.
- logScaleXbool
Flag to tell if the plot is done on a logarithmic scale for X. Default is False.
- logScaleYbool
Flag to tell if the plot is done on a logarithmic scale for Y. Default is False.
- Returns:
- graph
Graph
A graphical representation of the log-PDF or its iso_lines.
- graph
Notes
Only valid for univariate and bivariate distributions.
Examples
View the log-PDF of a univariate distribution:
>>> import openturns as ot >>> dist = ot.Normal() >>> graph = dist.drawLogPDF() >>> graph.setLegends(['normal log-pdf'])
View the iso-lines log-PDF of a bivariate distribution:
>>> import openturns as ot >>> dist = ot.Normal(2) >>> graph2 = dist.drawLogPDF() >>> graph2.setLegends(['iso- normal pdf']) >>> graph3 = dist.drawLogPDF([-10, -5],[5, 10], [511, 511])
- drawLowerExtremalDependenceFunction()¶
Draw the lower extremal dependence function.
We assume that the distribution is bivariate and that its copula is denoted by
. The lower extremal dependence function
is defined by:
Refer to Tail dependence coefficients to get more details.
- Returns:
- graph
Graph
The graph of the function
.
- graph
Examples
>>> import openturns as ot >>> copula = ot.FrankCopula() >>> graph = copula.drawLowerExtremalDependenceFunction()
- drawLowerTailDependenceFunction()¶
Draw the lower tail dependence function.
We assume that the distribution is bivariate and that its copula is denoted by
. The lower tail dependence function
is defined by:
Refer to Tail dependence coefficients to get more details.
- Returns:
- graph
Graph
Graph of the function
.
- graph
Examples
>>> import openturns as ot >>> copula = ot.FrankCopula() >>> graph = copula.drawLowerTailDependenceFunction()
- drawMarginal1DCDF(marginalIndex, xMin, xMax, pointNumber, logScale=False)¶
Draw the cumulative distribution function of a margin.
- Parameters:
- iint,
The index of the margin of interest.
- xMinfloat
The starting value that is used for meshing the x-axis.
- xMaxfloat, xMax > xMin
The ending value that is used for meshing the x-axis.
- nPointsint
The number of points that is used for meshing the x-axis.
- logScalebool
Flag to tell if the plot is done on a logarithmic scale. Default is False.
- iint,
- Returns:
- graph
Graph
A graphical representation of the CDF of the requested margin.
- graph
See also
Examples
>>> import openturns as ot >>> from openturns.viewer import View >>> distribution = ot.Normal(10) >>> graph = distribution.drawMarginal1DCDF(2, -6.0, 6.0, 100) >>> view = View(graph) >>> view.show()
- drawMarginal1DLogPDF(marginalIndex, xMin, xMax, pointNumber, logScale=False)¶
Draw the log-probability density function of a margin.
- Parameters:
- iint,
The index of the margin of interest.
- xMinfloat
The starting value that is used for meshing the x-axis.
- xMaxfloat, xMax > xMin
The ending value that is used for meshing the x-axis.
- nPointsint
The number of points that is used for meshing the x-axis.
- logScalebool
Flag to tell if the plot is done on a logarithmic scale. Default is False.
- iint,
- Returns:
- graph
Graph
A graphical representation of the log-PDF of the requested margin.
- graph
Examples
>>> import openturns as ot >>> from openturns.viewer import View >>> distribution = ot.Normal(10) >>> graph = distribution.drawMarginal1DLogPDF(2, -6.0, 6.0, 100) >>> view = View(graph) >>> view.show()
- drawMarginal1DPDF(marginalIndex, xMin, xMax, pointNumber, logScale=False)¶
Draw the probability density function of a margin.
- Parameters:
- iint,
The index of the margin of interest.
- xMinfloat
The starting value that is used for meshing the x-axis.
- xMaxfloat, xMax > xMin
The ending value that is used for meshing the x-axis.
- nPointsint
The number of points that is used for meshing the x-axis.
- logScalebool
Flag to tell if the plot is done on a logarithmic scale. Default is False.
- iint,
- Returns:
- graph
Graph
A graphical representation of the PDF of the requested margin.
- graph
Examples
>>> import openturns as ot >>> from openturns.viewer import View >>> distribution = ot.Normal(10) >>> graph = distribution.drawMarginal1DPDF(2, -6.0, 6.0, 100) >>> view = View(graph) >>> view.show()
- drawMarginal1DSurvivalFunction(marginalIndex, xMin, xMax, pointNumber, logScale=False)¶
Draw the cumulative distribution function of a margin.
- Parameters:
- iint,
The index of the margin of interest.
- xMinfloat
The starting value that is used for meshing the x-axis.
- xMaxfloat, xMax > xMin
The ending value that is used for meshing the x-axis.
- nPointsint
The number of points that is used for meshing the x-axis.
- logScalebool
Flag to tell if the plot is done on a logarithmic scale. Default is False.
- iint,
- Returns:
- graph
Graph
A graphical representation of the SurvivalFunction of the requested margin.
- graph
See also
Examples
>>> import openturns as ot >>> from openturns.viewer import View >>> distribution = ot.Normal(10) >>> graph = distribution.drawMarginal1DSurvivalFunction(2, -6.0, 6.0, 100) >>> view = View(graph) >>> view.show()
- drawMarginal2DCDF(firstMarginal, secondMarginal, xMin, xMax, pointNumber, logScaleX=False, logScaleY=False)¶
Draw the cumulative distribution function of a couple of margins.
- Parameters:
- iint,
The index of the first margin of interest.
- jint,
The index of the second margin of interest.
- xMinlist of 2 floats
The starting values that are used for meshing the x- and y- axes.
- xMaxlist of 2 floats, xMax > xMin
The ending values that are used for meshing the x- and y- axes.
- nPointslist of 2 ints
The number of points that are used for meshing the x- and y- axes.
- logScaleXbool
Flag to tell if the plot is done on a logarithmic scale for X. Default is False.
- logScaleYbool
Flag to tell if the plot is done on a logarithmic scale for Y. Default is False.
- iint,
- Returns:
- graph
Graph
A graphical representation of the marginal CDF of the requested couple of margins.
- graph
Examples
>>> import openturns as ot >>> from openturns.viewer import View >>> distribution = ot.Normal(10) >>> graph = distribution.drawMarginal2DCDF(2, 3, [-6.0] * 2, [6.0] * 2, [100] * 2) >>> view = View(graph) >>> view.show()
- drawMarginal2DLogPDF(firstMarginal, secondMarginal, xMin, xMax, pointNumber, logScaleX=False, logScaleY=False)¶
Draw the log-probability density function of a couple of margins.
- Parameters:
- iint,
The index of the first margin of interest.
- jint,
The index of the second margin of interest.
- xMinlist of 2 floats
The starting values that are used for meshing the x- and y- axes.
- xMaxlist of 2 floats, xMax > xMin
The ending values that are used for meshing the x- and y- axes.
- nPointslist of 2 ints
The number of points that are used for meshing the x- and y- axes.
- logScaleXbool
Flag to tell if the plot is done on a logarithmic scale for X. Default is False.
- logScaleYbool
Flag to tell if the plot is done on a logarithmic scale for Y. Default is False.
- iint,
- Returns:
- graph
Graph
A graphical representation of the marginal log-PDF of the requested couple of margins.
- graph
Examples
>>> import openturns as ot >>> from openturns.viewer import View >>> distribution = ot.Normal(10) >>> graph = distribution.drawMarginal2DLogPDF(2, 3, [-6.0] * 2, [6.0] * 2, [100] * 2) >>> view = View(graph) >>> view.show()
- drawMarginal2DPDF(firstMarginal, secondMarginal, xMin, xMax, pointNumber, logScaleX=False, logScaleY=False)¶
Draw the probability density function of a couple of margins.
- Parameters:
- iint,
The index of the first margin of interest.
- jint,
The index of the second margin of interest.
- xMinlist of 2 floats
The starting values that are used for meshing the x- and y- axes.
- xMaxlist of 2 floats, xMax > xMin
The ending values that are used for meshing the x- and y- axes.
- nPointslist of 2 ints
The number of points that are used for meshing the x- and y- axes.
- logScaleXbool
Flag to tell if the plot is done on a logarithmic scale for X. Default is False.
- logScaleYbool
Flag to tell if the plot is done on a logarithmic scale for Y. Default is False.
- iint,
- Returns:
- graph
Graph
A graphical representation of the marginal PDF of the requested couple of margins.
- graph
Examples
>>> import openturns as ot >>> from openturns.viewer import View >>> distribution = ot.Normal(10) >>> graph = distribution.drawMarginal2DPDF(2, 3, [-6.0] * 2, [6.0] * 2, [100] * 2) >>> view = View(graph) >>> view.show()
- drawMarginal2DSurvivalFunction(firstMarginal, secondMarginal, xMin, xMax, pointNumber, logScaleX=False, logScaleY=False)¶
Draw the cumulative distribution function of a couple of margins.
- Parameters:
- iint,
The index of the first margin of interest.
- jint,
The index of the second margin of interest.
- xMinlist of 2 floats
The starting values that are used for meshing the x- and y- axes.
- xMaxlist of 2 floats, xMax > xMin
The ending values that are used for meshing the x- and y- axes.
- nPointslist of 2 ints
The number of points that are used for meshing the x- and y- axes.
- logScaleXbool
Flag to tell if the plot is done on a logarithmic scale for X. Default is False.
- logScaleYbool
Flag to tell if the plot is done on a logarithmic scale for Y. Default is False.
- iint,
- Returns:
- graph
Graph
A graphical representation of the marginal SurvivalFunction of the requested couple of margins.
- graph
Examples
>>> import openturns as ot >>> from openturns.viewer import View >>> distribution = ot.Normal(10) >>> graph = distribution.drawMarginal2DSurvivalFunction(2, 3, [-6.0] * 2, [6.0] * 2, [100] * 2) >>> view = View(graph) >>> view.show()
- drawPDF(*args)¶
Draw the graph or of iso-lines of probability density function.
- Available constructors:
drawPDF(x_min, x_max, pointNumber, logScale)
drawPDF(lowerCorner, upperCorner, pointNbrInd, logScaleX, logScaleY)
drawPDF(lowerCorner, upperCorner)
- Parameters:
- xMinfloat, optional
The min-value of the mesh of the x-axis. Defaults uses the quantile associated to the probability level Distribution-QMin from the
ResourceMap
.- xMaxfloat, optional, xMax > xMin
The max-value of the mesh of the y-axis. Defaults uses the quantile associated to the probability level Distribution-QMax from the
ResourceMap
.- pointNumberint
The number of points that is used for meshing each axis. Defaults uses DistributionImplementation-DefaultPointNumber from the
ResourceMap
.- logScalebool
Flag to tell if the plot is done on a logarithmic scale. Default is False.
- lowerCornersequence of float, of dimension 2, optional
The lower corner
.
- upperCornersequence of float, of dimension 2, optional
The upper corner
.
- pointNbrInd
Indices
, of dimension 2 Number of points that is used for meshing each axis.
- logScaleXbool
Flag to tell if the plot is done on a logarithmic scale for X. Default is False.
- logScaleYbool
Flag to tell if the plot is done on a logarithmic scale for Y. Default is False.
- Returns:
- graph
Graph
A graphical representation of the PDF or its iso_lines.
- graph
See also
Notes
Only valid for univariate and bivariate distributions.
Examples
View the PDF of a univariate distribution:
>>> import openturns as ot >>> dist = ot.Normal() >>> graph = dist.drawPDF() >>> graph.setLegends(['normal pdf'])
View the iso-lines PDF of a bivariate distribution:
>>> import openturns as ot >>> dist = ot.Normal(2) >>> graph2 = dist.drawPDF() >>> graph2.setLegends(['iso- normal pdf']) >>> graph3 = dist.drawPDF([-10, -5],[5, 10], [511, 511])
- drawQuantile(*args)¶
Draw the quantile function.
- Parameters:
- qminfloat, in
The min value of the mesh of the x-axis.
- qmaxfloat, in
The max value of the mesh of the x-axis.
- nPointsint, optional
The number of points that is used for meshing the quantile curve. Defaults uses DistributionImplementation-DefaultPointNumber from the
ResourceMap
.- logScalebool
Flag to tell if the plot is done on a logarithmic scale. Default is False.
- qminfloat, in
- Returns:
- graph
Graph
A graphical representation of the quantile function.
- graph
Notes
This is implemented for univariate and bivariate distributions only. In the case of bivariate distributions, defined by its CDF
and its marginals
, the quantile of order
is the point
defined by
Examples
>>> import openturns as ot >>> from openturns.viewer import View >>> distribution = ot.Normal() >>> graph = distribution.drawQuantile() >>> view = View(graph) >>> view.show() >>> distribution = ot.JointDistribution([ot.Normal(), ot.Exponential(1.0)], ot.ClaytonCopula(0.5)) >>> graph = distribution.drawQuantile() >>> view = View(graph) >>> view.show()
- drawSurvivalFunction(*args)¶
Draw the cumulative distribution function.
- Available constructors:
drawSurvivalFunction(x_min, x_max, pointNumber, logScale)
drawSurvivalFunction(lowerCorner, upperCorner, pointNbrInd, logScaleX, logScaleY)
drawSurvivalFunction(lowerCorner, upperCorner)
- Parameters:
- xMinfloat, optional
The min-value of the mesh of the x-axis. Defaults uses the quantile associated to the probability level Distribution-QMin from the
ResourceMap
.- xMaxfloat, optional, xMax > xMin
The max-value of the mesh of the y-axis. Defaults uses the quantile associated to the probability level Distribution-QMax from the
ResourceMap
.- pointNumberint
The number of points that is used for meshing each axis. Defaults uses DistributionImplementation-DefaultPointNumber from the
ResourceMap
.- logScalebool
Flag to tell if the plot is done on a logarithmic scale. Default is False.
- lowerCornersequence of float, of dimension 2, optional
The lower corner
.
- upperCornersequence of float, of dimension 2, optional
The upper corner
.
- pointNbrInd
Indices
, of dimension 2 Number of points that is used for meshing each axis.
- logScaleXbool
Flag to tell if the plot is done on a logarithmic scale for X. Default is False.
- logScaleYbool
Flag to tell if the plot is done on a logarithmic scale for Y. Default is False.
- Returns:
- graph
Graph
A graphical representation of the SurvivalFunction.
- graph
Notes
Only valid for univariate and bivariate distributions.
Examples
View the SurvivalFunction of a univariate distribution:
>>> import openturns as ot >>> dist = ot.Normal() >>> graph = dist.drawSurvivalFunction() >>> graph.setLegends(['normal cdf'])
View the iso-lines SurvivalFunction of a bivariate distribution:
>>> import openturns as ot >>> dist = ot.Normal(2) >>> graph2 = dist.drawSurvivalFunction() >>> graph2.setLegends(['iso- normal cdf']) >>> graph3 = dist.drawSurvivalFunction([-10, -5],[5, 10], [511, 511])
- drawUpperExtremalDependenceFunction()¶
Draw the upper extremal dependence function.
We assume that the distribution is bivariate and that its copula is denoted by
. The upper extremal dependence function
is defined by:
Refer to Tail dependence coefficients to get more details.
- Returns:
- graph
Graph
Graph of the function
.
- graph
Examples
>>> import openturns as ot >>> copula = ot.FrankCopula() >>> graph = copula.drawUpperExtremalDependenceFunction()
- drawUpperTailDependenceFunction()¶
Draw the upper tail dependence function.
We assume that the distribution is bivariate and that its copula is denoted by
. The upper tail dependence function
is defined by:
Refer to Tail dependence coefficients to get more details.
- Returns:
- graph
Graph
Graph of the function
.
- graph
Examples
>>> import openturns as ot >>> copula = ot.FrankCopula() >>> graph = copula.drawUpperTailDependenceFunction()
- exp()¶
Transform distribution by exponential function.
- Returns:
- dist
Distribution
The transformed distribution.
- dist
- getAntecedent()¶
Accessor to the antecedent distribution.
- Returns:
- distX
Distribution
, univariate Antecedent distribution
.
- distX
- getCDF()¶
Return the CDF as a
Function
.- Returns:
- CDF
FunctionImplementation
The CDF of the distribution as a
Function
wrapping a copy of the distribution.
- CDF
- getCDFEpsilon()¶
Accessor to the CDF computation precision.
- Returns:
- CDFEpsilonfloat
CDF computation precision.
- getCentralMoment(n)¶
Accessor to the componentwise central moments.
- Parameters:
- kint
The order of the central moment.
- Returns:
- m
Point
Componentwise central moment of order k.
- m
See also
Notes
Central moments are centered with respect to the first-order moment:
- getCholesky()¶
Accessor to the Cholesky factor of the covariance matrix.
- Returns:
- L
TriangularMatrix
Cholesky factor of the covariance matrix.
- L
See also
- getClassName()¶
Accessor to the object’s name.
- Returns:
- class_namestr
The object class name (object.__class__.__name__).
- getCopula()¶
Accessor to the copula of the distribution.
- Returns:
- C
Distribution
Copula of the distribution.
- C
See also
- getCorrelation()¶
Accessor to the correlation matrix.
- Returns:
- R
CorrelationMatrix
The correlation matrix of the distribution.
- R
Notes
where the
are the margin standard deviations and
the covariance coefficients.
- getCovariance()¶
Accessor to the covariance matrix.
- Returns:
- Sigma
CovarianceMatrix
Covariance matrix.
- Sigma
Notes
The covariance is the second-order central moment. It is defined as:
- getDescription()¶
Accessor to the componentwise description.
- Returns:
- description
Description
Description of the components of the distribution.
- description
See also
- getDimension()¶
Accessor to the dimension of the distribution.
- Returns:
- nint
The number of components in the distribution.
- getDispersionIndicator()¶
Dispersion indicator accessor.
Defines a generic metric of the dispersion. When the standard deviation is not defined it falls back to the interquartile. Only available for 1-d distributions.
- Returns:
- dispersionfloat
Standard deviation or interquartile.
- getIntegrationNodesNumber()¶
Accessor to the number of Gauss integration points.
- Returns:
- Nint
Number of integration points.
- getInverseCholesky()¶
Accessor to the inverse Cholesky factor of the covariance matrix.
- Returns:
- Linv
TriangularMatrix
Inverse Cholesky factor of the covariance matrix.
- Linv
See also
- getInverseIsoProbabilisticTransformation()¶
Accessor to the inverse iso-probabilistic transformation.
- Returns:
- Tinv
Function
Inverse iso-probabilistic transformation.
- Tinv
Notes
The inverse iso-probabilistic transformation is defined as follows:
- getIsoProbabilisticTransformation()¶
Accessor to the iso-probabilistic transformation.
Refer to Isoprobabilistic transformations.
- Returns:
- T
Function
Iso-probabilistic transformation.
- T
See also
Notes
The iso-probabilistic transformation is defined as follows:
An iso-probabilistic transformation is a diffeomorphism [1] from
to
that maps realizations
of a random vector
into realizations
of another random vector
while preserving probabilities. It is hence defined so that it satisfies:
The present implementation of the iso-probabilistic transformation maps realizations
into realizations
of a random vector
with spherical distribution [2]. To be more specific:
if the distribution is elliptical, then the transformed distribution is simply made spherical using the Nataf (linear) transformation.
if the distribution has an elliptical Copula, then the transformed distribution is made spherical using the generalized Nataf transformation.
otherwise, the transformed distribution is the standard multivariate Normal distribution and is obtained by means of the Rosenblatt transformation.
- getKendallTau()¶
Accessor to the Kendall coefficients matrix.
- Returns:
- tau
CorrelationMatrix
Kendall coefficients matrix.
- tau
See also
Notes
The Kendall coefficients matrix is defined as:
- getKurtosis()¶
Accessor to the componentwise kurtosis.
- Returns:
- k
Point
Componentwise kurtosis.
- k
Notes
The kurtosis is the fourth-order central moment standardized by the standard deviation:
- getMarginal(*args)¶
Accessor to marginal distributions.
- Parameters:
- iint or list of ints,
Component(s) indice(s).
- iint or list of ints,
- Returns:
- distribution
Distribution
The marginal distribution of the selected component(s).
- distribution
- getMoment(n)¶
Accessor to the componentwise moments.
- Parameters:
- kint
The order of the moment.
- Returns:
- m
Point
Componentwise moment of order k.
- m
Notes
The componentwise moment of order
is defined as:
- getName()¶
Accessor to the object’s name.
- Returns:
- namestr
The name of the object.
- getPDFEpsilon()¶
Accessor to the PDF computation precision.
- Returns:
- PDFEpsilonfloat
PDF computation precision.
- getParameter()¶
Accessor to the parameter of the distribution.
- Returns:
- parameter
Point
Parameter values.
- parameter
- getParameterDescription()¶
Accessor to the parameter description of the distribution.
- Returns:
- description
Description
Parameter names.
- description
- getParameterDimension()¶
Accessor to the number of parameters in the distribution.
- Returns:
- n_parametersint
Number of parameters in the distribution.
See also
- getParametersCollection()¶
Accessor to the parameter of the distribution.
- Returns:
- parameters
PointWithDescription
Dictionary-like object with parameters names and values.
- parameters
- getPearsonCorrelation()¶
Accessor to the Pearson correlation matrix.
- Returns:
- R
CorrelationMatrix
Pearson’s correlation matrix.
- R
See also
Notes
Pearson’s correlation is defined as the normalized covariance matrix:
- getPositionIndicator()¶
Position indicator accessor.
Defines a generic metric of the position. When the mean is not defined it falls back to the median. Available only for 1-d distributions.
- Returns:
- positionfloat
Mean or median of the distribution.
- getProbabilities()¶
Accessor to the discrete probability levels.
- Returns:
- probabilities
Point
The probability levels of a discrete distribution.
- probabilities
- getRange()¶
Accessor to the range of the distribution.
- Returns:
- range
Interval
Range of the distribution.
- range
See also
Notes
The mathematical range is the smallest closed interval outside of which the PDF is zero. The numerical range is the interval outside of which the PDF is rounded to zero in double precision.
- getRealization()¶
Accessor to a pseudo-random realization from the distribution.
Refer to Distribution realizations.
- Returns:
- point
Point
A pseudo-random realization of the distribution.
- point
See also
- getRoughness()¶
Accessor to roughness of the distribution.
- Returns:
- rfloat
Roughness of the distribution.
See also
Notes
The roughness of the distribution is defined as the
-norm of its PDF:
- getSample(size)¶
Accessor to a pseudo-random sample from the distribution.
- Parameters:
- sizeint
Sample size.
- Returns:
- sample
Sample
A pseudo-random sample of the distribution.
- sample
See also
- getSampleByInversion(size)¶
Accessor to a pseudo-random sample from the distribution.
- Parameters:
- sizeint
Sample size.
- Returns:
- sample
Sample
A pseudo-random sample of the distribution based on conditional quantiles.
- sample
- getSampleByQMC(size)¶
Accessor to a low discrepancy sample from the distribution.
- Parameters:
- sizeint
Sample size.
- Returns:
- sample
Sample
A low discrepancy sample of the distribution based on Sobol’s sequences and conditional quantiles.
- sample
- getShapeMatrix()¶
Accessor to the shape matrix of the underlying copula if it is elliptical.
- Returns:
- shape
CorrelationMatrix
Shape matrix of the elliptical copula of a distribution.
- shape
See also
Notes
This is not the Pearson correlation matrix.
- getShiftedMoment(n, shift)¶
Accessor to the componentwise shifted moments.
- Parameters:
- kint
The order of the shifted moment.
- shiftsequence of float
The shift of the moment.
- Returns:
- m
Point
Componentwise central moment of order k.
- m
See also
Notes
The moments are centered with respect to the given shift
:
- getSingularities()¶
Accessor to the singularities of the PDF function.
It is defined for univariate distributions only, and gives all the singularities (i.e. discontinuities of any order) strictly inside of the range of the distribution.
- Returns:
- singularities
Point
The singularities of the PDF of an univariate distribution.
- singularities
- getSkewness()¶
Accessor to the componentwise skewness.
- Returns:
- d
Point
Componentwise skewness.
- d
Notes
The skewness is the third-order central moment standardized by the standard deviation:
- getSpearmanCorrelation()¶
Accessor to the Spearman correlation matrix.
- Returns:
- R
CorrelationMatrix
Spearman’s correlation matrix.
- R
See also
Notes
Spearman’s (rank) correlation is defined as the normalized covariance matrix of the copula (ie that of the uniform margins):
- getStandardDeviation()¶
Accessor to the componentwise standard deviation.
The standard deviation is the square root of the variance.
- Returns:
- sigma
Point
Componentwise standard deviation.
- sigma
See also
- getStandardDistribution()¶
Accessor to the standard distribution.
- Returns:
- standard_distribution
Distribution
Standard distribution.
- standard_distribution
See also
Notes
The standard distribution is determined according to the distribution properties. This is the target distribution achieved by the iso-probabilistic transformation.
- getStandardRepresentative()¶
Accessor to the standard representative distribution in the parametric family.
- Returns:
- std_repr_dist
Distribution
Standard representative distribution.
- std_repr_dist
Notes
The standard representative distribution is defined on a distribution-by-distribution basis, most of the time by scaling the distribution with bounded support to
or by standardizing (ie zero mean, unit variance) the distributions with unbounded support. It is the member of the family for which orthonormal polynomials will be built using generic algorithms of orthonormalization (see
StandardDistributionPolynomialFactory
).
- getSupport(*args)¶
Accessor to the support of the distribution.
- Parameters:
- interval
Interval
An interval to intersect with the support of the discrete part of the distribution.
- interval
- Returns:
- support
Interval
The intersection of the support of the discrete part of the distribution with the given interval.
- support
See also
Notes
The mathematical support
of the discrete part of a distribution is the collection of points with nonzero probability.
This is yet implemented for discrete distributions only.
- getSupportEpsilon()¶
Accessor to the support tolerance for discrete distributions.
- Returns:
- epsilonfloat
The support tolerance.
- hasEllipticalCopula()¶
Test whether the copula of the distribution is elliptical or not.
- Returns:
- testbool
Answer.
See also
- hasIndependentCopula()¶
Test whether the copula of the distribution is the independent one.
- Returns:
- testbool
Answer.
- hasName()¶
Test if the object is named.
- Returns:
- hasNamebool
True if the name is not empty.
- inverse()¶
Transform distribution by inverse function.
- Returns:
- dist
Distribution
The transformed distribution.
- dist
- isContinuous()¶
Test whether the distribution is continuous or not.
- Returns:
- testbool
Answer.
- isCopula()¶
Test whether the distribution is a copula or not.
- Returns:
- testbool
Answer.
Notes
A copula is a distribution with uniform margins on [0; 1].
- isDiscrete()¶
Test whether the distribution is discrete or not.
- Returns:
- testbool
Answer.
- isElliptical()¶
Test whether the distribution is elliptical or not.
- Returns:
- testbool
Answer.
Notes
A multivariate distribution is said to be elliptical if its characteristic function is of the form:
for specified vector
and positive-definite matrix
. The function
is known as the characteristic generator of the elliptical distribution.
- isIntegral()¶
Test whether the distribution is integer-valued or not.
- Returns:
- testbool
Answer.
- ln()¶
Transform distribution by natural logarithm function.
- Returns:
- dist
Distribution
The transformed distribution.
- dist
- log()¶
Transform distribution by natural logarithm function.
- Returns:
- dist
Distribution
The transformed distribution.
- dist
- setAntecedent(antecedent)¶
Fix the antecedent distribution which is push-forwarded.
- Parameters:
- distX
Distribution
, univariate Distribution of the antecedent
.
- distX
- setDescription(description)¶
Accessor to the componentwise description.
- Parameters:
- descriptionsequence of str
Description of the components of the distribution.
- setFunction(function)¶
Fix the function through which the distribution is push-forwarded.
- Parameters:
- g
Function
, the function
.
- g
- setIntegrationNodesNumber(integrationNodesNumber)¶
Accessor to the number of Gauss integration points.
- Parameters:
- Nint
Number of integration points.
- setName(name)¶
Accessor to the object’s name.
- Parameters:
- namestr
The name of the object.
- setParameter(parameter)¶
Accessor to the parameter of the distribution.
- Parameters:
- parametersequence of float
Parameter values.
- setParametersCollection(*args)¶
Accessor to the parameter of the distribution.
- Parameters:
- parameters
PointWithDescription
Dictionary-like object with parameters names and values.
- parameters
- setSolver(solver)¶
Solver accessor.
- Parameters:
- solver
Solver
The solver used for PDF/CDF computations.
- solver
- setSupportEpsilon(epsilon)¶
Accessor to the support tolerance for discrete distributions.
- Parameters:
- epsilonfloat
The support tolerance.
- sin()¶
Transform distribution by sine function.
- Returns:
- dist
Distribution
The transformed distribution.
- dist
- sinh()¶
Transform distribution by sinh function.
- Returns:
- dist
Distribution
The transformed distribution.
- dist
- sqr()¶
Transform distribution by square function.
- Returns:
- dist
Distribution
The transformed distribution.
- dist
- sqrt()¶
Transform distribution by square root function.
- Returns:
- dist
Distribution
The transformed distribution.
- dist
- tan()¶
Transform distribution by tangent function.
- Returns:
- dist
Distribution
The transformed distribution.
- dist
- tanh()¶
Transform distribution by tanh function.
- Returns:
- dist
Distribution
The transformed distribution.
- dist
Examples using the class¶
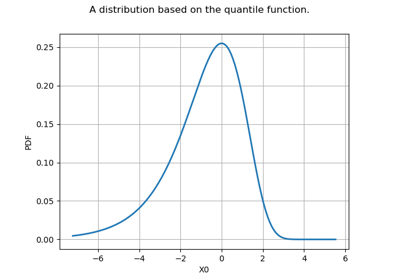
Create your own distribution given its quantile function