PythonFunction¶
- class PythonFunction(inputDim, outputDim, func=None, func_sample=None, gradient=None, hessian=None, n_cpus=None, copy=False, functionLinearity=None, variablesLinearity=None)¶
Override Function from Python.
- Parameters:
- inputDimpositive int
Dimension of the input vector
- outputDimpositive int
Dimension of the output vector
- funca callable python object, optional
Called when evaluated on a single point. Default is None.
- func_samplea callable python object, optional
Called when evaluated on multiple points at once. Default is None.
- gradienta callable python objects, optional
Returns the gradient as a 2-d sequence of float. Default is None (uses finite-difference).
- hessiana callable python object, optional
Returns the hessian as a 3-d sequence of float. Default is None (uses finite-difference).
- n_cpusint, default=None
Number of cpus on which func should be distributed using multiprocessing. If -1, it uses all the cpus available. If 1, it does nothing. Note that you should enforce the multiprocessing guidelines to enable this option, see https://docs.python.org/3/library/multiprocessing.html#multiprocessing-programming For example on Windows, the entry point of your program should be protected using the if __name__== ‘__main__’ idiom.
- copybool, optional
If True, input sample is converted into a Python 2-d sequence before calling func_sample. Otherwise, it is passed directly to func_sample. Default is False.
- functionLinearitybool, optional
Indicates if the function is linear. Default is False.
- variablesLinearitylist of bool, optional
Indicates for each input variable if the function is linear with regard to this variable. Default is [False]*inputDim
Methods
draw
(*args)Draw the output of function as a
Graph
.drawCrossCuts
(*args)Draw the 2D and 1D cross cuts of a 1D output function as a
GridLayout
.Accessor to the number of direct calls to the function.
Accessor to the object's name.
Accessor to the description of the inputs and outputs.
Accessor to the evaluation function.
Accessor to the number of times the evaluation of the function has been called.
Accessor to the gradient function.
Accessor to the number of times the gradient of the function has been called.
Accessor to the hessian function.
Accessor to the number of times the hessian of the function has been called.
getId
()Accessor to the object's id.
Accessor to the underlying implementation.
Accessor to the description of the input vector.
Accessor to the dimension of the input vector.
getMarginal
(*args)Accessor to marginal.
getName
()Accessor to the object's name.
Accessor to the description of the output vector.
Accessor to the number of the outputs.
Accessor to the parameter values.
Accessor to the parameter description.
Accessor to the dimension of the parameter.
gradient
(inP)Return the Jacobian transposed matrix of the function at a point.
hessian
(inP)Return the hessian of the function at a point.
isLinear
()Accessor to the linearity of the function.
isLinearlyDependent
(index)Accessor to the linearity of the function with regard to a specific variable.
parameterGradient
(inP)Accessor to the gradient against the parameter.
setDescription
(description)Accessor to the description of the inputs and outputs.
setEvaluation
(evaluation)Accessor to the evaluation function.
setGradient
(gradient)Accessor to the gradient function.
setHessian
(hessian)Accessor to the hessian function.
setInputDescription
(inputDescription)Accessor to the description of the input vector.
setName
(name)Accessor to the object's name.
setOutputDescription
(inputDescription)Accessor to the description of the output vector.
setParameter
(parameter)Accessor to the parameter values.
setParameterDescription
(description)Accessor to the parameter description.
setStopCallback
(callBack[, state])Set up a stop callback.
Notes
You must provide at least func or func_sample arguments. For efficiency reasons, these functions do not receive a
Point
orSample
as arguments, but a proxy object which gives access to internal object data. This object supports indexing, but nothing more. It must be wrapped into another object, for instancePoint
in func andSample
in func_sample, or in a Numpy array, for vectorized operations.Note that if PythonFunction is distributed (n_cpus > 1), the traceback of a raised exception by a func call is lost due to the way multiprocessing dispatches and handles func calls. This can be solved by temporarily deactivating n_cpus during the development of the wrapper or by manually handling the distribution of the wrapper with external libraries like joblib that keep track of a raised exception and shows the traceback to the user.
Examples
>>> import openturns as ot >>> def a_exec(X): ... Y = [3.0 * X[0] - X[1]] ... return Y >>> def a_grad(X): ... dY = [[3.0], [-1.0]] ... return dY >>> f = ot.PythonFunction(2, 1, a_exec, gradient=a_grad) >>> X = [100.0, 100.0] >>> Y = f(X) >>> print(Y) [200] >>> dY = f.gradient(X) >>> print(dY) [[ 3 ] [ -1 ]]
Same example, but optimized for best performance with Numpy when function is going to be evaluated on large samples.
>>> import openturns as ot >>> import numpy as np >>> def a_exec_sample(X): ... Xarray = np.asarray(X) ... Y = 3.0 * Xarray[:,0] - Xarray[:,1] ... return np.expand_dims(Y, axis=1) >>> def a_grad(X): ... dY = [[3.0], [-1.0]] ... return dY >>> f = ot.PythonFunction(2, 1, func_sample=a_exec_sample, gradient=a_grad) >>> X = [100.0, 100.0] >>> Y = f(X) >>> print(Y) [200] >>> dY = f.gradient(X) >>> print(dY) [[ 3 ] [ -1 ]]
- __init__(*args)¶
- draw(*args)¶
Draw the output of function as a
Graph
.- Available usages:
draw(inputMarg, outputMarg, centralPoint, xiMin, xiMax, ptNb, scale)
draw(firstInputMarg, secondInputMarg, outputMarg, centralPoint, xiMin_xjMin, xiMax_xjMax, ptNbs, scale, isFilled)
draw(xiMin, xiMax, ptNb, scale)
draw(xiMin_xjMin, xiMax_xjMax, ptNbs, scale)
- Parameters:
- outputMarg, inputMargint,
outputMarg is the index of the marginal to draw as a function of the marginal with index inputMarg.
- firstInputMarg, secondInputMargint,
In the 2D case, the marginal outputMarg is drawn as a function of the two marginals with indexes firstInputMarg and secondInputMarg.
- centralPointsequence of float
Central point with dimension equal to the input dimension of the function.
- xiMin, xiMaxfloat
Define the interval where the curve is plotted.
- xiMin_xjMin, xiMax_xjMaxsequence of float of dimension 2.
In the 2D case, define the intervals where the curves are plotted.
- ptNbint
The number of points to draw the curves.
- ptNbslist of int of dimension 2
The number of points to draw the contour in the 2D case.
- scalebool
scale indicates whether the logarithmic scale is used either for one or both axes:
ot.GraphImplementation.NONE or 0: no log scale is used,
ot.GraphImplementation.LOGX or 1: log scale is used only for horizontal data,
ot.GraphImplementation.LOGY or 2: log scale is used only for vertical data,
ot.GraphImplementation.LOGXY or 3: log scale is used for both data.
- isFilledbool
isFilled indicates whether the contour graph is filled or not
- outputMarg, inputMargint,
Notes
We note
where
and
, with
and
.
In the first usage:
Draws graph of the given 1D outputMarg marginal
as a function of the given 1D inputMarg marginal with respect to the variation of
in the interval
, when all the other components of
are fixed to the corresponding components of the centralPoint
. Then OpenTURNS draws the graph:
for any
where
is defined by the equation:
In the second usage:
Draws the iso-curves of the given outputMarg marginal
as a function of the given 2D firstInputMarg and secondInputMarg marginals with respect to the variation of
in the interval
, when all the other components of
are fixed to the corresponding components of the centralPoint
. Then OpenTURNS draws the graph:
for any
where
is defined by the equation:
In the third usage:
The same as the first usage but only for function
.
In the fourth usage:
The same as the second usage but only for function
.
Examples
>>> import openturns as ot >>> from openturns.viewer import View >>> f = ot.SymbolicFunction('x', 'sin(2*pi_*x)*exp(-x^2/2)') >>> graph = f.draw(-1.2, 1.2, 100) >>> View(graph).show()
- drawCrossCuts(*args)¶
Draw the 2D and 1D cross cuts of a 1D output function as a
GridLayout
.- Parameters:
- centralPointlist of float
Central point with dimension equal to the input dimension of the function.
- xMin, xMaxlist of float
Define the interval where the curve is plotted.
- pointNumber
Indices
The number of points to draw the contours and the curves.
- withMonoDimensionalCutsbool, optional
withMonoDimensionalCuts indicates whether the mono dimension cuts are drawn or not Default value is specified in the CrossCuts-DefaultWithMonoDimensionalCuts ResourceMap key.
- isFilledbool, optional
isFilled indicates whether the contour graphs are filled or not Default value is specified in the Contour-DefaultIsFilled ResourceMap key
- vMin, vMaxfloat, optional
Define the interval used to build the color map for the contours If not specified, these values are computed to best fit the graphs. Either specify both values or do not specify any.
Notes
We note
where
and
, with
and
.
In all usages, draw the 1D and 2D cross cuts of
as a function of all input coordinates for 1D cuts and all couples of coordinates for 2D cuts. Variable coordinates
are sampled regularly using
points in the interval
, when all the other components of
are fixed to the corresponding components of the centralPoint
. In the first usage, vMin and vMax are evaluated as the min and max of all samples of the function value calculated in all cross cuts performed.
For 1D cross cuts the graph shows:
for any
where
is defined by the equation:
For 2D cross cuts:
for any
where
is defined by the equation:
Examples
>>> import openturns as ot >>> from openturns.viewer import View >>> f = ot.SymbolicFunction(['x0', 'x1', 'x2'], ['sin(1*pi_*x0) + x1 - x2 ^ 2']) >>> grid = f.drawCrossCuts([0., 0., 0.], [-3., -3, -3], [3, 3, 3], [100, 20, 20], True, True) >>> View(grid).show()
- getCallsNumber()¶
Accessor to the number of direct calls to the function.
- Returns:
- calls_numberint
Integer that counts the number of times the function has been called directly through the () operator.
- getClassName()¶
Accessor to the object’s name.
- Returns:
- class_namestr
The object class name (object.__class__.__name__).
- getDescription()¶
Accessor to the description of the inputs and outputs.
- Returns:
- description
Description
Description of the inputs and the outputs.
- description
Examples
>>> import openturns as ot >>> f = ot.SymbolicFunction(['x1', 'x2'], ... ['2 * x1^2 + x1 + 8 * x2 + 4 * cos(x1) * x2 + 6']) >>> print(f.getDescription()) [x1,x2,y0]
- getEvaluation()¶
Accessor to the evaluation function.
- Returns:
- evaluation
EvaluationImplementation
The evaluation function.
- evaluation
Examples
>>> import openturns as ot >>> f = ot.SymbolicFunction(['x1', 'x2'], ... ['2 * x1^2 + x1 + 8 * x2 + 4 * cos(x1) * x2 + 6']) >>> print(f.getEvaluation()) [x1,x2]->[2 * x1^2 + x1 + 8 * x2 + 4 * cos(x1) * x2 + 6]
- getEvaluationCallsNumber()¶
Accessor to the number of times the evaluation of the function has been called.
- Returns:
- evaluation_calls_numberint
Integer that counts the number of times the evaluation of the function has been called since its creation. This may include indirect calls via finite-difference gradient or Hessian.
- getGradient()¶
Accessor to the gradient function.
- Returns:
- gradient
GradientImplementation
The gradient function.
- gradient
Examples
To get the gradient formulas when the function is a
SymbolicFunction
. The indices are. The corresponding formula is
. >>> import openturns as ot >>> f = ot.SymbolicFunction([‘x1’, ‘x2’], … [‘2 * x1^2 + x1 + 8 * x2 + 4 * cos(x1) * x2 + 6’]) >>> formula_deriv_x1 = f.getGradient().getImplementation().getFormula(0,0) >>> formula_deriv_x2 = f.getGradient().getImplementation().getFormula(1,0)
- getGradientCallsNumber()¶
Accessor to the number of times the gradient of the function has been called.
- Returns:
- gradient_calls_numberint
Integer that counts the number of times the gradient of the Function has been called since its creation. Note that if the gradient is implemented by a finite difference method, the gradient calls number is equal to 0 and the different calls are counted in the evaluation calls number.
- getHessian()¶
Accessor to the hessian function.
- Returns:
- hessian
HessianImplementation
The hessian function.
- hessian
Examples
To get the hessian formulas when the function is a
SymbolicFunction
. The indices are. The corresponding formula is
. >>> import openturns as ot >>> f = ot.SymbolicFunction([‘x1’, ‘x2’], … [‘2 * x1^2 + x1 + 8 * x2 + 4 * cos(x1) * x2 + 6’]) >>> formula_hessian_000 = f.getHessian().getImplementation().getFormula(0,0,0) >>> formula_hessian_010 = f.getHessian().getImplementation().getFormula(0,1,0) >>> formula_hessian_110 = f.getHessian().getImplementation().getFormula(1,1,0) >>> formula_hessian_001 = f.getHessian().getImplementation().getFormula(0,0,0) >>> formula_hessian_011 = f.getHessian().getImplementation().getFormula(0,1,0) >>> formula_hessian_111 = f.getHessian().getImplementation().getFormula(1,1,0)
- getHessianCallsNumber()¶
Accessor to the number of times the hessian of the function has been called.
- Returns:
- hessian_calls_numberint
Integer that counts the number of times the hessian of the Function has been called since its creation. Note that if the hessian is implemented by a finite difference method, the hessian calls number is equal to 0 and the different calls are counted in the evaluation calls number.
- getId()¶
Accessor to the object’s id.
- Returns:
- idint
Internal unique identifier.
- getImplementation()¶
Accessor to the underlying implementation.
- Returns:
- implImplementation
A copy of the underlying implementation object.
- getInputDescription()¶
Accessor to the description of the input vector.
- Returns:
- description
Description
Description of the input vector.
- description
Examples
>>> import openturns as ot >>> f = ot.SymbolicFunction(['x1', 'x2'], ... ['2 * x1^2 + x1 + 8 * x2 + 4 * cos(x1) * x2 + 6']) >>> print(f.getInputDescription()) [x1,x2]
- getInputDimension()¶
Accessor to the dimension of the input vector.
- Returns:
- inputDimint
Dimension of the input vector
.
Examples
>>> import openturns as ot >>> f = ot.SymbolicFunction(['x1', 'x2'], ... ['2 * x1^2 + x1 + 8 * x2 + 4 * cos(x1) * x2 + 6']) >>> print(f.getInputDimension()) 2
- getMarginal(*args)¶
Accessor to marginal.
- Parameters:
- indicesint or list of ints
Set of indices for which the marginal is extracted.
- Returns:
- marginal
Function
Function corresponding to either
or
, with
and
.
- marginal
- getName()¶
Accessor to the object’s name.
- Returns:
- namestr
The name of the object.
- getOutputDescription()¶
Accessor to the description of the output vector.
- Returns:
- description
Description
Description of the output vector.
- description
Examples
>>> import openturns as ot >>> f = ot.SymbolicFunction(['x1', 'x2'], ... ['2 * x1^2 + x1 + 8 * x2 + 4 * cos(x1) * x2 + 6']) >>> print(f.getOutputDescription()) [y0]
- getOutputDimension()¶
Accessor to the number of the outputs.
- Returns:
- number_outputsint
Dimension of the output vector
.
Examples
>>> import openturns as ot >>> f = ot.SymbolicFunction(['x1', 'x2'], ... ['2 * x1^2 + x1 + 8 * x2 + 4 * cos(x1) * x2 + 6']) >>> print(f.getOutputDimension()) 1
- getParameterDescription()¶
Accessor to the parameter description.
- Returns:
- parameter
Description
The parameter description.
- parameter
- getParameterDimension()¶
Accessor to the dimension of the parameter.
- Returns:
- parameterDimensionint
Dimension of the parameter.
- gradient(inP)¶
Return the Jacobian transposed matrix of the function at a point.
- Parameters:
- pointsequence of float
Point where the Jacobian transposed matrix is calculated.
- Returns:
- gradient
Matrix
The Jacobian transposed matrix of the function at point.
- gradient
Notes
Let
where the
are the components functions. Then, the gradient evaluated at
is the matrix with
rows and
columns defined by:
Examples
>>> import openturns as ot >>> f = ot.SymbolicFunction(['x1', 'x2'], ... ['2 * x1^2 + x1 + 8 * x2 + 4 * cos(x1) * x2 + 6','x1 + x2']) >>> print(f.gradient([3.14, 4])) [[ 13.5345 1 ] [ 4.00001 1 ]]
- hessian(inP)¶
Return the hessian of the function at a point.
- Parameters:
- pointsequence of float
Point where the hessian of the function is calculated.
- Returns:
- hessian
SymmetricTensor
Hessian of the function at point.
- hessian
Notes
Let
where the
are the components functions. Then, the hessian evaluated at
is a symmetric tensor with
sheets, each one composed of
rows and
columns. The
-th sheet is defined by:
Examples
>>> import openturns as ot >>> f = ot.SymbolicFunction(['x1', 'x2'], ... ['2 * x1^2 + x1 + 8 * x2 + 4 * cos(x1) * x2 + 6','x1 + x2']) >>> print(f.hessian([3.14, 4])) sheet #0 [[ 20 -0.00637061 ] [ -0.00637061 0 ]] sheet #1 [[ 0 0 ] [ 0 0 ]]
- isLinear()¶
Accessor to the linearity of the function.
- Returns:
- linearbool
True if the function is linear, False otherwise.
- isLinearlyDependent(index)¶
Accessor to the linearity of the function with regard to a specific variable.
- Parameters:
- indexint
The index of the variable with regard to which linearity is evaluated.
- Returns:
- linearbool
True if the function is linearly dependent on the specified variable, False otherwise.
- parameterGradient(inP)¶
Accessor to the gradient against the parameter.
- Returns:
- gradient
Matrix
The gradient.
- gradient
- setDescription(description)¶
Accessor to the description of the inputs and outputs.
- Parameters:
- descriptionsequence of str
Description of the inputs and the outputs.
Examples
>>> import openturns as ot >>> f = ot.SymbolicFunction(['x1', 'x2'], ... ['2 * x1^2 + x1 + 8 * x2 + 4 * cos(x1) * x2 + 6']) >>> print(f.getDescription()) [x1,x2,y0] >>> f.setDescription(['a','b','y']) >>> print(f.getDescription()) [a,b,y]
- setEvaluation(evaluation)¶
Accessor to the evaluation function.
- Parameters:
- function
EvaluationImplementation
The evaluation function.
- function
- setGradient(gradient)¶
Accessor to the gradient function.
- Parameters:
- gradient_function
GradientImplementation
The gradient function.
- gradient_function
Examples
>>> import openturns as ot >>> f = ot.SymbolicFunction(['x1', 'x2'], ... ['2 * x1^2 + x1 + 8 * x2 + 4 * cos(x1) * x2 + 6']) >>> f.setGradient(ot.CenteredFiniteDifferenceGradient( ... ot.ResourceMap.GetAsScalar('CenteredFiniteDifferenceGradient-DefaultEpsilon'), ... f.getEvaluation()))
- setHessian(hessian)¶
Accessor to the hessian function.
- Parameters:
- hessian_function
HessianImplementation
The hessian function.
- hessian_function
Examples
>>> import openturns as ot >>> f = ot.SymbolicFunction(['x1', 'x2'], ... ['2 * x1^2 + x1 + 8 * x2 + 4 * cos(x1) * x2 + 6']) >>> f.setHessian(ot.CenteredFiniteDifferenceHessian( ... ot.ResourceMap.GetAsScalar('CenteredFiniteDifferenceHessian-DefaultEpsilon'), ... f.getEvaluation()))
- setInputDescription(inputDescription)¶
Accessor to the description of the input vector.
- Parameters:
- description
Description
Description of the input vector.
- description
- setName(name)¶
Accessor to the object’s name.
- Parameters:
- namestr
The name of the object.
- setOutputDescription(inputDescription)¶
Accessor to the description of the output vector.
- Parameters:
- description
Description
Description of the output vector.
- description
- setParameter(parameter)¶
Accessor to the parameter values.
- Parameters:
- parametersequence of float
The parameter values.
- setParameterDescription(description)¶
Accessor to the parameter description.
- Parameters:
- parameter
Description
The parameter description.
- parameter
- setStopCallback(callBack, state=None)¶
Set up a stop callback.
Can be used to programmatically stop an evaluation.
- Parameters:
- callbackcallable
Returns a bool deciding whether to stop or continue.
Examples using the class¶
Linear Regression with interval-censored observations
Calibrate a parametric model: a quick-start guide to calibration
Kolmogorov-Smirnov : get the statistics distribution
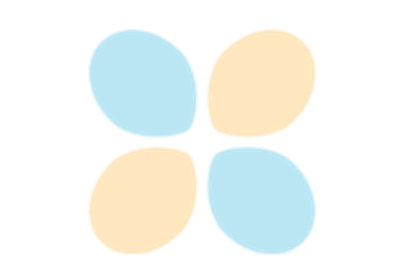
Defining Python and symbolic functions: a quick start introduction to functions
Plot the log-likelihood contours of a distribution
Gaussian Process Regression: surrogate model with continuous and categorical variables
Estimate a multivariate integral with IteratedQuadrature
Using the FORM - SORM algorithms on a nonlinear function
Non parametric Adaptive Importance Sampling (NAIS)