Gibbs¶
- class Gibbs(*args)¶
Gibbs sampling method.
Refer to Bayesian calibration, The Metropolis-Hastings Algorithm.
- Parameters:
- samplerssequence of
MetropolisHastings
List of samplers for each bloc of the chain
- samplerssequence of
Notes
Assume we want to sample from a complicated joint distribution. The Gibbs algorithm is a Markov Chain Monte-Carlo algorithm which calls samplers (implemented as
MetropolisHastings
objects) in a predetermined sequence (by default) or randomly: seesetUpdatingMethod()
to manually choose the behavior, and note the default behavior can be changed by setting the Gibbs-DefaultUpdatingMethodResourceMap
key. Each sampler samples from the conditional distributions of one or several components.Examples
>>> import openturns as ot >>> ot.RandomGenerator.SetSeed(0) >>> chainDim = 3 >>> # Observations >>> obsDim = 1 >>> obsSize = 10 >>> y = [-9.50794871493506, -3.83296694500105, -2.44545713047953, ... 0.0803625289211318, 1.01898069723583, 0.661725805623086, ... -1.57581204592385, -2.95308465670895, -8.8878164296758, ... -13.0812290405651] >>> y_obs = ot.Sample([[yi] for yi in y]) >>> # Parameters >>> covariates = ot.Sample(obsSize, chainDim) >>> for i in range(obsSize): ... for j in range(chainDim): ... covariates[i, j] = (-2 + 5.0 * i / 9.0) ** j >>> # Model >>> fullModel = ot.SymbolicFunction( ... ['p1', 'p2', 'p3', 'x1', 'x2', 'x3'], ... ['p1*x1+p2*x2+p3*x3', '1.0']) >>> parametersSet = range(chainDim) >>> parametersValue = [0.0] * len(parametersSet) # 0.0 is a placeholder >>> linkFunction = ot.ParametricFunction(fullModel, parametersSet, parametersValue) >>> # Calibration parameters >>> # Proposal distribution >>> prop = ot.Uniform(-1.0, 1.0) >>> # Prior distribution >>> sigma0 = [10.0]*chainDim >>> # Covariance matrix >>> Q0_inv = ot.CorrelationMatrix(chainDim) >>> for i in range(chainDim): ... Q0_inv[i, i] = sigma0[i] * sigma0[i] >>> mu0 = [0.0]*chainDim >>> # x0 ~ N(mu0, sigma0) >>> prior = ot.Normal(mu0, Q0_inv) >>> # Conditional distribution y~N(z, 1.0) >>> conditional = ot.Normal() >>> # Create a Gibbs sampler >>> coll = [ot.RandomWalkMetropolisHastings(prior, mu0, prop, [i]) for i in range(chainDim)] >>> for mh in coll: mh.setLikelihood(conditional, y_obs, linkFunction, covariates) >>> sampler = ot.Gibbs(coll) >>> # Get a realization >>> mu = sampler.getRealization() >>> # Create a Gibbs sampler which updates one randomly chosen component at each step >>> sampler2 = ot.Gibbs(coll) >>> sampler2.setUpdatingMethod(ot.Gibbs.RANDOM_UPDATING) >>> mu2 = sampler2.getRealization()
Methods
Accessor to the antecedent RandomVector in case of a composite RandomVector.
Accessor to the object's name.
Accessor to the covariance of the RandomVector.
Accessor to the description of the RandomVector.
Accessor to the dimension of the RandomVector.
Accessor to the distribution of the RandomVector.
Accessor to the domain of the Event.
Accessor to the Function in case of a composite RandomVector.
Get the history storage.
getId
()Accessor to the object's id.
getMarginal
(*args)Get the random vector corresponding to the
marginal component(s).
getMean
()Accessor to the mean of the RandomVector.
Get the list of Metropolis-Hastings samplers.
getName
()Accessor to the object's name.
Accessor to the comparaison operator of the Event.
Accessor to the parameter of the distribution.
Accessor to the parameter description of the distribution.
Get the stochastic process.
Compute one realization of the RandomVector.
Get the posterior recompute flags.
getSample
(size)Compute realizations of the RandomVector.
Accessor to the object's shadowed id.
Accessor to the threshold of the Event.
Get how the order of the samplers is determined.
Accessor to the object's visibility state.
hasName
()Test if the object is named.
Test if the object has a distinguishable name.
Accessor to know if the RandomVector is a composite one.
isEvent
()Whether the random vector is an event.
setDescription
(description)Accessor to the description of the RandomVector.
setHistory
(strategy)Set the history storage.
setName
(name)Accessor to the object's name.
setParameter
(parameters)Accessor to the parameter of the distribution.
setShadowedId
(id)Accessor to the object's shadowed id.
setUpdatingMethod
(updatingMethod)Set how the order of the samplers is determined.
setVisibility
(visible)Accessor to the object's visibility state.
- __init__(*args)¶
- getAntecedent()¶
Accessor to the antecedent RandomVector in case of a composite RandomVector.
- Returns:
- antecedent
RandomVector
Antecedent RandomVector
in case of a
CompositeRandomVector
such as:.
- antecedent
- getClassName()¶
Accessor to the object’s name.
- Returns:
- class_namestr
The object class name (object.__class__.__name__).
- getCovariance()¶
Accessor to the covariance of the RandomVector.
- Returns:
- covariance
CovarianceMatrix
Covariance of the considered
UsualRandomVector
.
- covariance
Examples
>>> import openturns as ot >>> distribution = ot.Normal([0.0, 0.5], [1.0, 1.5], ot.CorrelationMatrix(2)) >>> randomVector = ot.RandomVector(distribution) >>> ot.RandomGenerator.SetSeed(0) >>> print(randomVector.getCovariance()) [[ 1 0 ] [ 0 2.25 ]]
- getDescription()¶
Accessor to the description of the RandomVector.
- Returns:
- description
Description
Describes the components of the RandomVector.
- description
- getDimension()¶
Accessor to the dimension of the RandomVector.
- Returns:
- dimensionpositive int
Dimension of the RandomVector.
- getDistribution()¶
Accessor to the distribution of the RandomVector.
- Returns:
- distribution
Distribution
Distribution of the considered
UsualRandomVector
.
- distribution
Examples
>>> import openturns as ot >>> distribution = ot.Normal([0.0, 0.0], [1.0, 1.0], ot.CorrelationMatrix(2)) >>> randomVector = ot.RandomVector(distribution) >>> ot.RandomGenerator.SetSeed(0) >>> print(randomVector.getDistribution()) Normal(mu = [0,0], sigma = [1,1], R = [[ 1 0 ] [ 0 1 ]])
- getDomain()¶
Accessor to the domain of the Event.
- Returns:
- domain
Domain
Describes the domain of an event.
- domain
- getFunction()¶
Accessor to the Function in case of a composite RandomVector.
- Returns:
- function
Function
Function used to define a
CompositeRandomVector
as the image through this function of the antecedent:
.
- function
- getHistory()¶
Get the history storage.
- Returns:
- history
HistoryStrategy
Used to record the chain.
- history
- getId()¶
Accessor to the object’s id.
- Returns:
- idint
Internal unique identifier.
- getMarginal(*args)¶
Get the random vector corresponding to the
marginal component(s).
- Parameters:
- iint or list of ints,
Indicates the component(s) concerned.
is the dimension of the RandomVector.
- iint or list of ints,
- Returns:
- vector
RandomVector
RandomVector restricted to the concerned components.
- vector
Notes
Let’s note
a random vector and
a set of indices. If
is a
UsualRandomVector
, the subvector is defined by. If
is a
CompositeRandomVector
, defined bywith
,
some scalar functions, the subvector is
.
Examples
>>> import openturns as ot >>> distribution = ot.Normal([0.0, 0.0], [1.0, 1.0], ot.CorrelationMatrix(2)) >>> randomVector = ot.RandomVector(distribution) >>> ot.RandomGenerator.SetSeed(0) >>> print(randomVector.getMarginal(1).getRealization()) [0.608202] >>> print(randomVector.getMarginal(1).getDistribution()) Normal(mu = 0, sigma = 1)
- getMean()¶
Accessor to the mean of the RandomVector.
- Returns:
- mean
Point
Mean of the considered
UsualRandomVector
.
- mean
Examples
>>> import openturns as ot >>> distribution = ot.Normal([0.0, 0.5], [1.0, 1.5], ot.CorrelationMatrix(2)) >>> randomVector = ot.RandomVector(distribution) >>> ot.RandomGenerator.SetSeed(0) >>> print(randomVector.getMean()) [0,0.5]
- getMetropolisHastingsCollection()¶
Get the list of Metropolis-Hastings samplers.
- Returns:
- samplerssequence of
MetropolisHastings
List of all Metropolis-Hastings samplers used in the Gibbs algorithm
- samplerssequence of
- getName()¶
Accessor to the object’s name.
- Returns:
- namestr
The name of the object.
- getOperator()¶
Accessor to the comparaison operator of the Event.
- Returns:
- operator
ComparisonOperator
Comparaison operator used to define the
RandomVector
.
- operator
- getParameter()¶
Accessor to the parameter of the distribution.
- Returns:
- parameter
Point
Parameter values.
- parameter
- getParameterDescription()¶
Accessor to the parameter description of the distribution.
- Returns:
- description
Description
Parameter names.
- description
- getProcess()¶
Get the stochastic process.
- Returns:
- process
Process
Stochastic process used to define the
RandomVector
.
- process
- getRealization()¶
Compute one realization of the RandomVector.
- Returns:
- aRealization
Point
Sequence of values randomly determined from the RandomVector definition. In the case of an event: one realization of the event (considered as a Bernoulli variable) which is a boolean value (1 for the realization of the event and 0 else).
- aRealization
See also
Examples
>>> import openturns as ot >>> distribution = ot.Normal([0.0, 0.0], [1.0, 1.0], ot.CorrelationMatrix(2)) >>> randomVector = ot.RandomVector(distribution) >>> ot.RandomGenerator.SetSeed(0) >>> print(randomVector.getRealization()) [0.608202,-1.26617] >>> print(randomVector.getRealization()) [-0.438266,1.20548]
- getRecomputeLogPosterior()¶
Get the posterior recompute flags.
- Returns:
- recompute
Indices
Whether the posterior at the state received from the previous sampler is recomputed. For debug use only.
- recompute
- getSample(size)¶
Compute realizations of the RandomVector.
- Parameters:
- nint,
Number of realizations needed.
- nint,
- Returns:
- realizations
Sample
n sequences of values randomly determined from the RandomVector definition. In the case of an event: n realizations of the event (considered as a Bernoulli variable) which are boolean values (1 for the realization of the event and 0 else).
- realizations
See also
Examples
>>> import openturns as ot >>> distribution = ot.Normal([0.0, 0.0], [1.0, 1.0], ot.CorrelationMatrix(2)) >>> randomVector = ot.RandomVector(distribution) >>> ot.RandomGenerator.SetSeed(0) >>> print(randomVector.getSample(3)) [ X0 X1 ] 0 : [ 0.608202 -1.26617 ] 1 : [ -0.438266 1.20548 ] 2 : [ -2.18139 0.350042 ]
- getShadowedId()¶
Accessor to the object’s shadowed id.
- Returns:
- idint
Internal unique identifier.
- getThreshold()¶
Accessor to the threshold of the Event.
- Returns:
- thresholdfloat
Threshold of the
RandomVector
.
- getUpdatingMethod()¶
Get how the order of the samplers is determined.
Available values are:
0 (Gibbs.DETERMINISTIC_UPDATING): the samplers are sequentially called in the given order.
1 (Gibbs.RANDOM_UPDATING): one of the samplers is randomly selected to produce the next realization.
Refer to the
setUpdatingMethod()
documentation for details.- Returns:
- updatingMethodint
See above for the possible values and their meaning.
- getVisibility()¶
Accessor to the object’s visibility state.
- Returns:
- visiblebool
Visibility flag.
- hasName()¶
Test if the object is named.
- Returns:
- hasNamebool
True if the name is not empty.
- hasVisibleName()¶
Test if the object has a distinguishable name.
- Returns:
- hasVisibleNamebool
True if the name is not empty and not the default one.
- isComposite()¶
Accessor to know if the RandomVector is a composite one.
- Returns:
- isCompositebool
Indicates if the RandomVector is of type Composite or not.
- isEvent()¶
Whether the random vector is an event.
- Returns:
- isEventbool
Whether it takes it values in {0, 1}.
- setDescription(description)¶
Accessor to the description of the RandomVector.
- Parameters:
- descriptionstr or sequence of str
Describes the components of the RandomVector.
- setHistory(strategy)¶
Set the history storage.
- Parameters:
- history
HistoryStrategy
Used to record the chain.
- history
- setName(name)¶
Accessor to the object’s name.
- Parameters:
- namestr
The name of the object.
- setParameter(parameters)¶
Accessor to the parameter of the distribution.
- Parameters:
- parametersequence of float
Parameter values.
- setShadowedId(id)¶
Accessor to the object’s shadowed id.
- Parameters:
- idint
Internal unique identifier.
- setUpdatingMethod(updatingMethod)¶
Set how the order of the samplers is determined.
Available values are:
0 (Gibbs.DETERMINISTIC_UPDATING): the samplers are sequentially called in the given order.
1 (Gibbs.RANDOM_UPDATING): one of the samplers is randomly selected to produce the next realization.
Note that under DETERMINISTIC_UPDATING, all samplers are called in the specified order every time
getRealization()
is called. By contrast, under RANDOM_UPDATING, only one sampler is called bygetRealization()
, and this sampler is randomly chosen among all specified samplers.- Parameters:
- updatingMethodint
See above for the possible values and their meaning.
- setVisibility(visible)¶
Accessor to the object’s visibility state.
- Parameters:
- visiblebool
Visibility flag.
Examples using the class¶
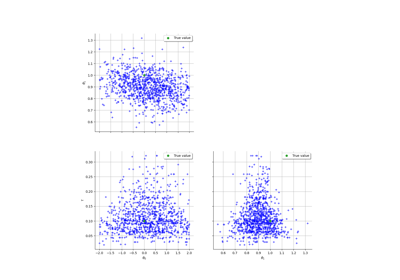
Linear Regression with interval-censored observations